A contextual review on our 3rd blogiversary
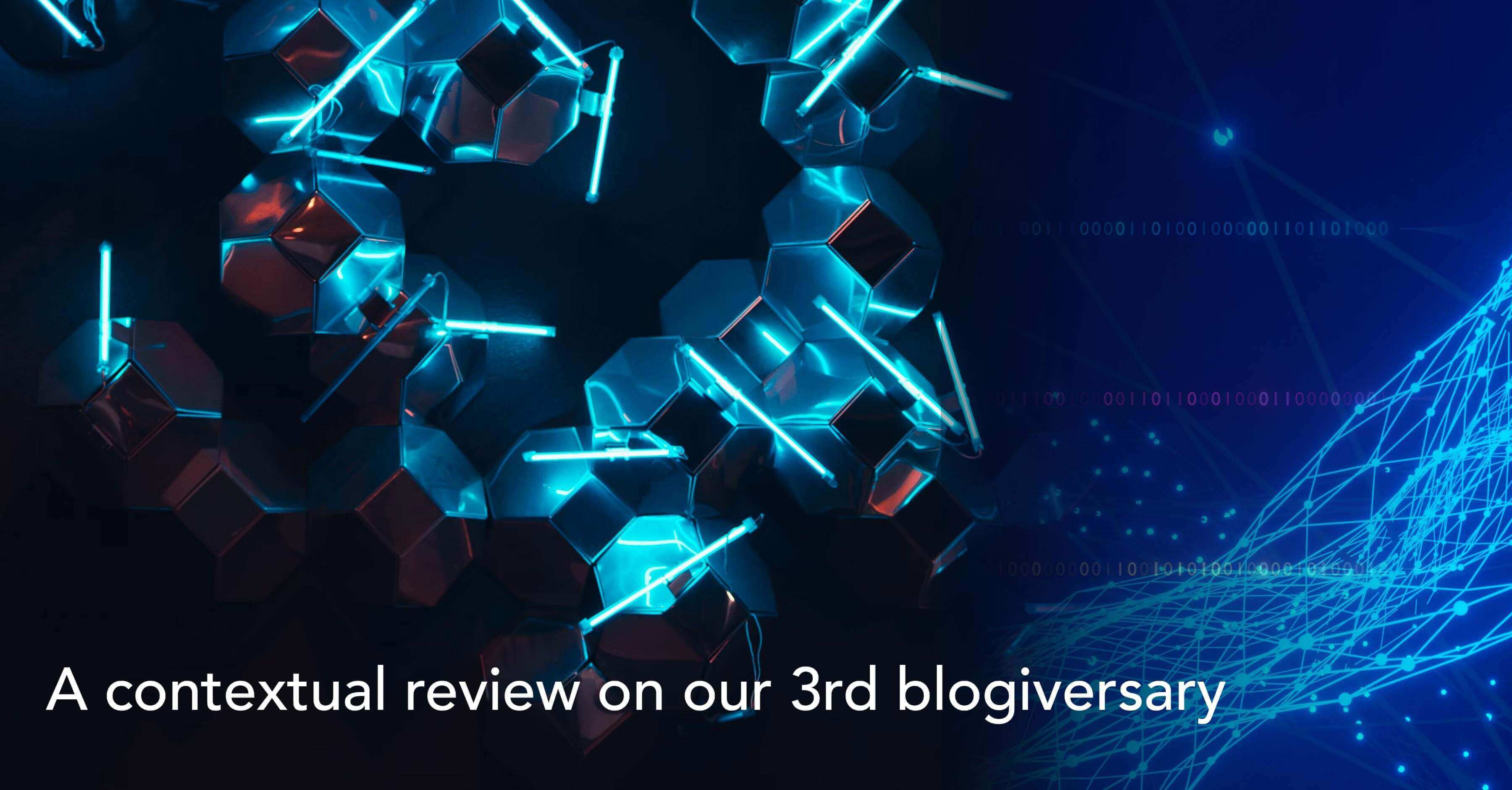
It’s been another eventful year for the life sciences industry and for the team at BioStrand, and much of the ideas, trends, topics, and technologies are often reflected in our Powering Biotherapeutic Intelligence blogs. As we approach our third blogiversary, here’s a contextual synopsis of the narrative we have developed over the past year or so.
We start with in-silico drug discovery, a pertinent anchor point for this briefing.
In-silico drug discovery
With drug discovery becoming increasingly data-driven, combining in-silico models, powered by advanced technologies like Artificial Intelligence (AI), Machine Learning (ML), and Deep Learning (DL), and experimental approaches is rapidly becoming the norm. Despite certain challenges, such as reproducibility, for example, AI/ML-based, in-silico drug discovery models are driving transformative disruption in terms of cost- and time-efficiently converting biological big data into pharmaceutical value. The emergence of computationally intensive AI/ML-powered in silico models is shifting the focus to cloud-based platforms that empower life sciences companies to continuously adapt and upgrade to the latest technologies and capabilities.
Increased computing power, however, is only part of the solution to realizing end-to-end AI-driven in-silico drug discovery. Unless the much more complex challenge of the Information Integration Dilemma (IID) in systems biology is addressed, no amount of computing power will enable the holistic, systems-level analysis of biological complexity.
The Information Integration Dilemma
The Information Integration Dilemma (IID) refers to the challenges of integrating, standardizing, and analyzing complex, multimodal biological data. Drug discovery and development encompasses an astonishing volume and variety of data types, sources, and formats that exemplify the gravity of the dilemma. There are compound libraries scaling into the millions and dispersed across several multi-format multi-domain silos. Then there’s multi-omics data with the added complexity of different omics layers, characterized by different technologies and assays and represented by the heterogeneity of datasets with different sources, modalities, formats, etc. This is just the structured biomedical data, which leaves the typically un-/under-utilized biomedical data embedded as free-text information in electronic health records (EHRs), clinical notes, scientific literature, and other real-world data sources.
The challenge, therefore, is to organize the entire biosphere into one vast, easily accessible multi-level biotherapeutic intelligence network that includes data/metadata about sequence, syntax, and protein structure and unstructured data from free-text sources.
At IPA, our revolutionary solution based on HYFT® patterns already enables us to organize heterogeneous pools of omics data into a unified network of data objects, with each data object enriched with DNA, RNA, and amino acid data and embedded with metadata comprising high-level information about position, function, structure, cell type, species, pathogenicity, pathway, Mechanism of Action (MoA) role, etc. This network provides comprehensive information on the entire biosphere and serves as the data foundation for the Lensai platform.
This year much of our blog narrative has been focused on the best technologies, techniques, and frameworks to closing the gap of text and the biosphere in life sciences research. Our approach to navigating this complex, expansive topic broadly falls into two streams, with the first covering emerging trends in data management frameworks and data architectures, and the second exploring the role of technologies like natural language processing (NLP), large language models (LLMs), and knowledge graphs (knowledge graphs ).
Closing the gap between biomedical text and the Biosphere
1. Data architecture & data management essentials
In a three-part data management blog series, we addressed several points related to data architectures and data management frameworks that would be critical to realizing at-scale AI-powered in-silico life sciences research.
The key emphasis of the data series was on the No AI Without IA theorem. The reasoning itself is fairly straightforward: the successful deployment of scalable, high-outcome, future-proof AI will require a modern information architecture (IA) to ensure data quality and data governance integrated with a solid data foundation capable of transforming and integrating heterogeneous data, at scale. Ergo, a unified data + information architecture will be critical to standardizing and streamlining the AI/ML lifecycle and enabling AI development and operationalization at scale.
Data architectures are also evolving into the active metadata era. Modern approaches such as the data fabric provide a more centralized approach to connecting and unifying accessing multi-format, multi-location data. A key advantage of this approach is the use of metadata combined with semantic knowledge graphs and AI/ML to enable an AI-powered model of data integration and management where all existing and incoming data is automatically and contextually integrated.
Concurrently, the approach to information and data management strategy also has to evolve. The FAIR principles define the foundational attributes of an effective scientific data management model. However holistic data management in life sciences will require a more composite approach that combines the best elements and practices of different standards and frameworks into one coherent strategy.
2. NLP, LLMs, and knowledge graphs
Over the past couple of years, we have covered NLP technologies from multiple perspectives, including their importance in biomedical research and their role in enhancing the ROI of drug discovery.
This year, apart from delving a bit deeper into the nuanced differences between NLP, natural language generation (NLG), and natural language understanding (NLU), we expanded our focus to the emerging trend of bio LLMs.
LLMs have the potential to transform life sciences research and have been shown to substantially outperform contemporary bioNLP tools. In fact, combining domain-specific biomedical LLMs with ontology-driven systems could help expand the scope and capabilities of bioNLP applications. However, there are still a few critical concerns regarding LLMs, such as the lack of domain-specific knowledge, semantic understanding, access to up-to-date information, and interpretability and explainability, are addressed.
The good news is that combining knowledge graphs and LLMs can create a synergistic model that enhances the capabilities of each system while mitigating the limitations of both. Take, for instance, the black box concern related to the interpretability and explainability of transformer-based LLMs. A unified approach that combines ontology-driven bioNLP, natural language-driven LLMs, and domain-specific knowledge graphs, ontologies, and dictionaries can provide LLMs with the traceable factual knowledge required to address interpretability concerns. LLMs, in return, can enrich knowledge graphs with real-world data, from EHRs, scientific publications, etc.,
LLMs’ also lack semantic intelligence, relying instead on statistically extrapolated meaning, an approach that is not optimal for dealing with complex biomedical vocabulary. Semantic analysis is a critical capability for accurately converting words into meaning for bioNLP applications. Knowledge graphs, that are structured around domain-specific ontologies and the semantic relationships between different entities, can provide LLMs with the semantic intelligence required to cope with complex life sciences applications.
The knowledge cut-off limitation of LLMs also means that they are unable to retrieve up-to-date information from external sources. This limitation can be addressed by Retrieval Augmented Generation (RAG), an approach that leverages external knowledge retrieval mechanisms to enhance the factual accuracy and the continuous knowledge enrichment of LLMs.
For the life sciences industry, the value of knowledge graphs is vastly more than simply streamlining LLM deployment.
The key subtext, however, of this narrative arc is that it will take a unified platform, combining HYFT® patterns, ontology-driven bioNLP, AI-driven LLMs, semantic knowledge graphs, and retrieval-augmented generation models, to address the information integration dilemma in data-driven life sciences research. To see such a platform in action, please drop us a line here.
Protein prediction, microbiomes, and new talent at BioStrand
To round off this review, we start with two deep dives from our data sciences team.
On the topic of protein structure prediction, Sébastien Lemal forecasts that the success of AlphaFold2 with the single protein structure problem opens up opportunities for tackling even more complicated challenges, such as the prediction of protein complexes and interactions. Read the Tom Vieijra follow-through on combining structure prediction with a physics-based approach to enhance the accuracy of protein structure prediction.
Explore the microbiome-immune axis and the microbiome's impact on immunotherapy efficacy with Julie Delanote.
And finally, say hello to the newest members of the ever-expanding BioStrand team. If you are interested in a career at the cutting edge of the biotech AI revolution, Apply Now.
Subscribe to our Blog and get new articles right after publication into your inbox.