Connecting the dots: Why knowledge graphs matter
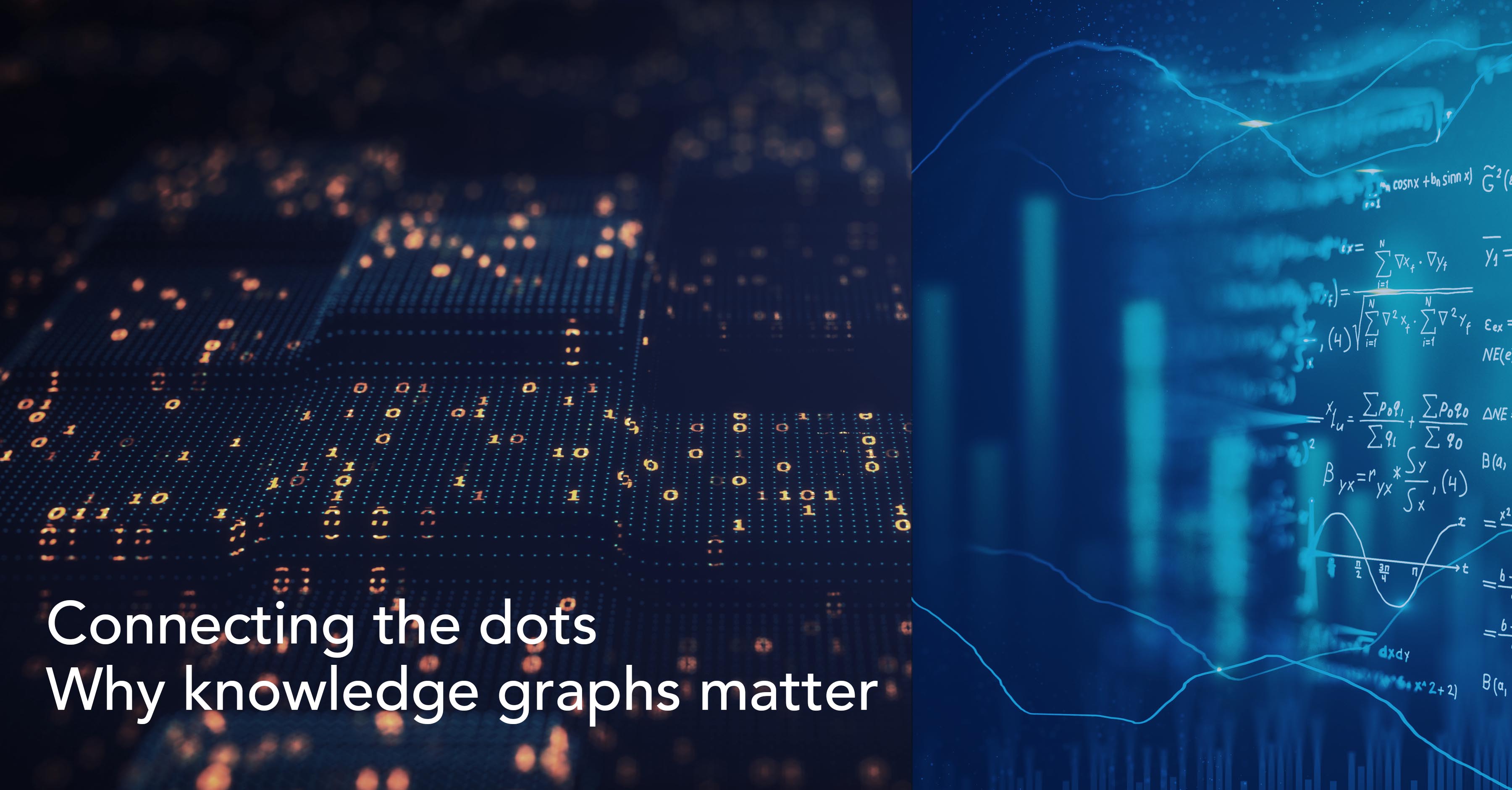
Knowledge Graphs (KGs) have become a must-know innovation that will drive transformational benefits in data-centric AI applications across industries. KGs, big data and AI are complementary concepts that together address the challenges of integrating, unifying, analyzing and querying vast volumes of diverse and complex data.
There are several inherent advantages to the KG approach to organizing and representing information. Unlike traditional flat data structures, for instance, a KG framework is designed to model multilevel hierarchical, associative, and causal relationships that more accurately represent real-world data. The application of a semantic layer to data also makes it easier for both humans and machines to understand the context and significance of information.
Here then are some of the key features and benefits of knowledge graphs.
Efficient Data Integration:
Integrate disparate data sources and break down information silos
AI-specific data management, including automated data and metadata integration, is a critical component in successful data-centric AI. However, factors such as data complexity, quality, and accessibility pose integration challenges that are barriers to AI adoption. Data-centric AI requires a modern approach to data integration that integrates all organizational data entities into one unified semantic representation based on context (ontologies, metadata, domain knowledge, etc.) and time (temporal relationships).
Knowledge graphs (KGs) have become the ideal platform for the contextual integration and representation of complex data ecosystems. They enable the integration of information from multiple data sources and map them to a common ontology in order to create a comprehensive, consistent, and connected representation of all organizational data entities. The scalability of this approach, across large volumes of heterogeneous, structured, semi-structured, and multimodal unstructured data from diverse data sources and silos, makes them ideal for automated data acquisition, transformation, and integration. Knowledge extraction methods can be used to classify entities and relations, identify matching entities (entity linking, entity resolution), combine entities into a single representation (entity fusion), and match and merge ontology concepts to create a KG graph data model.
There are several advantages to KG data models. They have the flexibility to scale across complex heterogeneous data structures. When integrated with natural language technologies (NLT), KGs can help train language models on domain-specific knowledge and natural language technologies can streamline the construction of knowledge models. They allow for more intuitive querying of complex data even by users without specialized data science knowledge. They can evolve to assimilate new data, sources, definitions, and use cases without manageability and accessibility loss. They provide consistent and unified access to all organization knowledge that is typically distributed across different data silos and systems.
Rich Contextualization:
Capture relationships and provide a holistic view of data
Context is a critical component of learning, for both humans and machines. Contextual information will be key to the development of next-generation AI systems that adopt a human approach to transform data into knowledge that enables more human-like decision-making.
KGs leverage the powers of context and relations to embed data with intelligence. By organizing data based on factual interconnections and interrelations, they add real-world meaning to data that makes it easier for AI systems to extract knowledge from vast volumes of data.
A key organizing principle of KGs is the provision of an additional metadata layer that organizes data based on context to support logical reasoning and knowledge discovery. The organizing principle could take many forms including controlled vocabularies, such as taxonomies, ontologies, etc., entity resolution and analysis, and tagging, categorization, and classification.
With KGs, smart behavior is encoded directly into the data so that the graph itself can dynamically understand connections and associations between entities, eliminating the need to manually program every new piece of information.
Knowledge graphs provide context for decision support and can be further classified based on use cases as actioning KGs (data management) and decisioning KGs (analytics), and as context-rich KGs (internal knowledge management), external-sensing KGs (external data mapping), and natural language processing KGs.
Enhanced Search and Discovery:
Enable precise and context-aware search results
The first step towards understanding how KGs transform the data search and discovery function is to understand the distinction between data search and data discovery.
Data search broadly refers to a scenario in which users are looking for specific information that they know or assume to exist. This is a framework that allows users to seek and extract relevant information from volumes of non-relevant data. Data discovery is focused more on proactively enabling users to surface and explore new information and ideas that are potentially related to the actual search string. Discovery essentially is search powered by context.
KGs contextually integrate all entities and relationships across different data silos and systems into a unified semantic layer. This enables them to deliver more accurate and comprehensive search results and to provide context-relevant connections and relationships that promote knowledge discovery. Users can then follow the contextual links that are most pertinent to their interest to delve deeper into the data thereby boosting data utilization and value. And perhaps equally importantly, the intuitive and flexible querying capabilities of KGs allow even non-technical users to explore data and discover new insights.
It is estimated that graph-based models can help organizations enhance their ability to find, access, and reuse information by as much as 30% and up to 75% faster.
Knowledge graphs in life sciences
Knowledge graphs are transformative frameworks that enable a structured, connected, and semantically-enhanced approach to organize and interpret data holistically. They provide the foundations for companies to create a uniform data fabric across different environments and technologies and operationalizing AI at scale.
For the life sciences industry, knowledge graphs represent a powerful tool for integrating, harmonizing, and governing heterogeneous and siloed data while ensuring data quality, lineage, and compliance. They enable the creation of a centralized, shared and holistic repository of knowledge that can be continually updated and enriched with new entities, relationships, and attributes.
According to Gartner, graph technologies will drive 80% of data and analytics innovations by 2025. If you are interested in integrating the innovative potential of KGs and AI/ML to your research pipeline, please drop us a line.
Subscribe to our Blog and get new articles right after publication into your inbox.