Beyond AlphaFold 2: The next frontier in macromolecular structure prediction
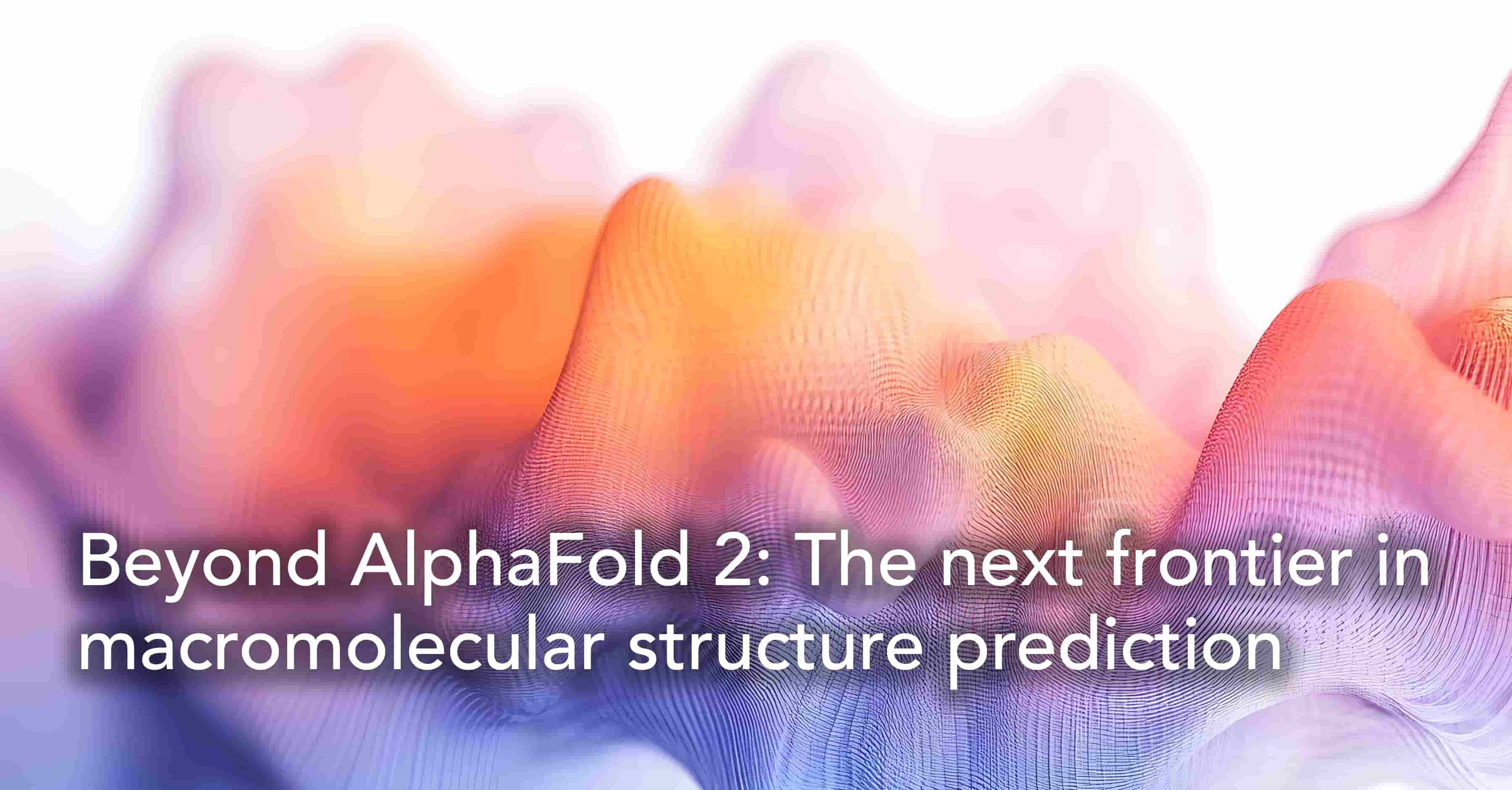
Audio version
Introduction
As a testament to the recent breakthrough of deep-learning technologies in the field of (structural) bioinformatics, half of the Nobel Prize in Chemistry 2024 [1] has been awarded to John Jumper and Demis Hassabis, the main contributors to AlphaFold 2, the other half to Prof. David Baker (University of Washington, Seattle). Speaking about breakthroughs is not an understatement: as of this time of writing, the original AlphaFold2 publication [2] has been cited more than 27,800 times (according to Google Scholar [3]). For comparison, on Feb 21, 2023 (roughly 1.5 years ago), the number of citations was just 8,783. AlphaFold 2 is a solution to the protein folding problem and can predict with near experimental accuracy the structure of proteins as long as their primary structures (the sequence of amino acids along the protein chain) are known. This technology has been integrated with the LENSᵃⁱ™ in silico discovery platform, and we have discussed it in length in different blog posts since the public release of AlphaFold2 [4, 5, 6]. In this new blog post, we will review how these latest developments are impacting drug discovery, what can be technically achieved with current technology, and assess limitations that hinder discovery processes and future outcomes. Finally, we will briefly present how these breakthrough technologies are integrated within the BioStrand LENSai platform.
AlphaFold 3: Expanding the horizons of structural biology
In May 2024, DeepMind and Isomorphic Labs (a subsidiary of Alphabet founded by Demis Hassabis) released AlphaFold 3, with a closed-source web server accessible to academic researchers. At the protein structure prediction, AlphaFold 3 is an improvement over AlphaFold 2: it is better at predicting monomeric and multimeric structures [7], specifically in the field of antibody-antigen complex modeling where AlphaFold 2 was notoriously lacking [8].
In addition to proteins, AlphaFold 3 introduces capabilities for predicting the structures of nucleic acids (such as RNA) and small molecules. This expanded versatility makes it a powerful tool for drug discovery, as it can model the interactions between proteins and ligands. These substantial improvements are critical advancements for biotherapeutic development, where understanding these interactions is essential for developing targeted therapies like monoclonal antibodies and, in the broader sense, developing in silico screening strategies.
While the authors' study in the original publication shows beyond state-of-the-art performance for many tasks, third-party benchmarks are still missing for AlphaFold 3, partly due to the limited capacity of the web server and its initial closed-source nature. As announced earlier in the year [13], the source code was released in November 2024, although with a restricted license; thus AlphaFold 3 is less susceptible to tweaking, in-depth analysis, and integration to protein design pipelines compared to AlphaFold 2 [6].
In addition to the base AlphaFold 3 code, several third-party initiatives have taken the initiative to reproduce the architecture of the model, as it was done for AlphaFold 2 before its release [3], and many AlphaFold 3-like prediction pipelines have been released, such as Boltz-1 or Chai-1.
AlphaFold 3 success rate on different benchmark sets, for (from the left to the right) ligand docking, nucleic acids, covalent modifications and protein predictions; and compared to state-of-the-art methods. Adapted from Ref. 7.
So far, the substantial improvements of AlphaFold 3 outbalance its known limitations: for instance, the algorithm struggles with molecule chirality. Atomic clashes also occur, specifically for large proteins, so molecules can partially overlap, which is physically impossible. As success rates for some tasks remain low, “hallucinations” may happen. Finally, predictions remain static in nature and completely ignore any dynamical aspect of molecular interaction. These limitations are, of course, not specific to AlphaFold 3, and there are many ways to mitigate these shortcomings by integrating structure prediction within a broader framework for molecular modeling. For instance, models generated by AlphaFold can be used in Molecular Dynamics simulations to assess conformational dynamics, interaction energies between molecular partners, and much more.
Structure prediction in practice
Despite their fame, the practical use of structure prediction tools such as AlphaFold is not often well understood. These tools works within the paradigm that for a given input of sequential molecular data (sequence of amino acids for protein, nucleic acids, …), there is a “static” 3D structure (atomic position) which can be predicted solely from this data, representative on the interaction between all involved atoms. While this picture is simplistic and ignores the dynamical nature of macromolecular interactions, which is only partially captured by static representations.
Within this paradigm, ideally, one would expect that a given input yields a single prediction. Yet, this is not the case. For AlphaFold 2 monomers, there are 5 trained model weights which outputs 5 predictions for a single inputs. These predictions are scored and ranked by the model, using a so-called confidence metric. The most accurate model is expected to be ranked at the top. For AlphaFold 2 Multimer, it has been found that more than 5 predictions are necessary to obtain accurate models; thus the standard pipeline outputs 25 models which can be later inspected.
However, it is not always guaranteed that the most accurate prediction (compared to a ground structure structure) is always ranked at the top. Typically, a criterion is defined, and the top ranking model match that criterion, then the prediction is considered correct. In benchmarks, the top-N success rate is the number of correct predictions up to rank N. For instance, the top-1 success rate is the number of case with a successful top-1 prediction over the full dataset, the top-5 success rate consider all ranks up to 5, and so on. For a given set of prediction, the probability of finding a correct prediction increases.
In the case of protein complexes, it is notoriously hard to predict bound conformations using traditional docking techniques. The top-1 success rate for traditional methods (docking) is typically low (a few percent), and for these methods, it is often necessary to consider a wider pool of predictions along with complementary methods such as molecular dynamics to assess what is the likely correct method. AlphaFold 2 Multimer became the gold standard for protein complex predictions, and AlphaFold 3 extends to a much larger landscape of interactions, involving nearly all kinds of molecules in life science.
Once a satisfying prediction is obtained, downstream tasks may be performed with other tools than AlphaFold. Long molecular dynamics simulations can be used to sample the conformational landscape, identifying key functional domains, assessing the stability, performing mutagenesis analysis, and so on. Structure prediction is thus one of the early step in the drug discovery phases, and must be complemented with additional analyses.
Is AlphaFold 2 obsolete?
With the release of AlphaFold 3, one might wonder if AlphaFold 2 is now outdated. The answer is rather nuanced. While AlphaFold 3 offers improvements in specific areas like nucleic acid/protein predictions and ligand docking, AlphaFold 2 remains highly relevant.
The reality is that AlphaFold 2 has been integrated within more intricate workflows, which, in some cases, extends its use beyond simple structure prediction and, in other cases, significantly improves its performance in specific tasks such as multimeric predictions as witnessed from the results of CASP15 [14]. For example, AlphaFold 2 and ProteinMPNN have been integrated into a pipeline for a complete de novo complex protein fold design with targeted properties [23, 24]. Another example is protein complex prediction, which is highly improved through techniques like massive sampling and dropout layer activation during inference [15]. This improvement beyond base performance is done through slight tweaking, without re-training or fine-tuning the neural networks.
Antibody-antigen modeling: A persistent challenge
One particular shortcoming of the first release of the AlphaFold 2 pipeline is its lack of accuracy for predicting antibody-antigen or nanobody-antigen bound complexes [8]. The problem itself is notoriously difficult, and it comes as no surprise that the observed accuracy of AlphaFold 2 on many other tasks motivated further inquiry with respect to their performance on this specific use case. An initial benchmark showed very low success rate (~10%) in this area [8], compared to other tasks.
It has been argued that while the integration of coevolution data was as the source of AlphaFold 2’s overall performance, such data do not exist for antibody-antigen binding, which partially explains this lack of accurate results.
Nevertheless, a much more recent study [17] highlighted increased performance for newer versions of AlphaFold Multimer (2.2 and 2.3) compared to the initial release. Moreover, novel strategies, such as the aforementioned augmented sampling approach, have shown larger leap in success rates. Indeed, a key feature of AlphaFold 2 (and successors) is the ability to rank its own predictions using predicted accuracy metrics: in massive sampling approaches, such metrics can be used to identify conformational models of relevance [16]. Using a benchmark dataset of 37 antibody-antigen complexes (not part of the training set of AlphaFold 2), it has been reported [17] that the top-1 success rate was ~60%, which is quite close to the ~64% top-1 success rate of AlphaFold 3 (albeit on a much larger dataset [7], sampling 1,000 seeds); similar metrics were reported by other groups as well on other benchmark datasets [9]. In less than two years, the top-1 success rate has been multiplied by a factor of 6!
If we consider larger pools of predictions from the top ranked one, up to top-25, the success-rate come close to 75% percent, meaning there is at least one correct prediction amongst 25, in 3 out of 4 cases. Combining physics-based approaches with deep-learning predictions typically increase complex structure prediction success rate. In massive sampling approaches, a large amount of predictions are analysed (a few thousands at least), and in practice correct predictions have a large probability of being retrieved in the set.
Antibody–antigen success rate by different AlphaFold versions/implementation. The success rate is calculated based on the percentage of cases that had at least one model among their top N predictions that met a specified level of CAPRI accuracy. Adapted from Ref. 17
Powering up drug discovery with LENSai
At BioStrand, we have integrated AlphaFold 2 into our LENSai platform to enhance drug discovery workflows. The platform allows users to perform protein structure predictions within an optimized environment that balances speed and accuracy. Most comparable services contain limitations such as limited sequence lengths or reduced database search (~600 GB of storage, compared to the 2.62 TB of storage for the full database), which are tradeoffs to accommodate heavy usage, with a potential drop in accuracy in some cases.
AlphaFold workflows readily available in AWS HealthOmics Ready2Run.
Improvements like GPU acceleration (at inference and structure relaxation levels) may be desired, especially if the input sequences are large. To further improve performance, parallelization (which is not a feature of the official DeepMind release) may be highly desired in the case of augmented sampling. Beyond standard structure prediction tasks, LENSai incorporates advanced features like automated reporting and augmented sampling to improve prediction confidence.
Moreover, LENSai integrates AlphaFold into specialized pipelines such as Epitope Mapping and Affinity Maturation (a case study has been documented and is accessible in the following link [22]). These pipelines exploit state-of-the-art methodologies (physics- and data-driven approaches) to accelerate discovery rates in biotherapeutic research.
Conclusion: The future of AI-driven structural biology
The field of structural biology witnessed groundbreaking progress within the past few years. AlphaFold’s journey from version 1 to version 3 represents a transformative leap in our ability to predict biological macromolecule structures with unprecedented accuracy. While AlphaFold 3 expands into new territories like nucleic acids and small molecules, it does not render its predecessor obsolete. Both versions offer unique strengths that can be leveraged depending on specific research needs and pave new ways toward more intricate in silico and de novo generation of biotherapeutics to be integrated within pre-clinical research workflows. As we continue to integrate these models into platforms like LENSai, we are improving our ability to predict protein structures and accelerating the entire drug discovery process—from target identification to lead optimization. The future is bright for AI-driven structural biology, and BioStrand is at the forefront of this exciting revolution.
References
[1] https://www.nobelprize.org/prizes/chemistry/2024/press-release/ , consulted 2024/10/09
[2] Jumper, John, et al. "Highly accurate protein structure prediction with AlphaFold." Nature 596.7873 (2021): 583-589.
[3] https://scholar.google.com/scholar?cites=6286436358625670901, consulted 2024/10/21
[4] https://blog.biostrand.ai/explained-a-brief-look-into-alphafold-2 , consulted 2024/10/21
[5] https://blog.biostrand.ai/explained-how-to-plot-the-prediction-quality-metrics-with-alphafold2 , consulted 2024/10/21
[6] https://blog.biostrand.ai/scaling-up-structural-biology-with-alphafold2 , consulted 2024/10/21
[7] Abramson, Josh, et al. "Accurate structure prediction of biomolecular interactions with AlphaFold 3." Nature (2024): 1-3.
[8] Yin, R., Feng, B. Y., Varshney, A., & Pierce, B. G. (2022). Benchmarking AlphaFold for protein complex modeling reveals accuracy determinants. Protein Science, 31(8), e4379.
[9] Bernard, C., Postic, G., Ghannay, S., & Tahi, F. (2024). Has AlphaFold 3 reached its success for RNAs?. bioRxiv, 2024-06.
[12] Editorial, Nature 629, 728 (2024)
[13] https://x.com/pushmeet/status/1790086453520691657 , consulted 2024/10/21
[14] Proteins: Structure, Function, and Bioinformatics: Volume 91, Issue 12 - Special Issue: CASP15: Critical Assessment of methods for Structure Prediction, 15th round, C1-C4, 1535-1951 (2023)
[15] Wallner, B. (2023). Improved multimer prediction using massive sampling with AlphaFold in CASP15. Proteins: Structure, Function, and Bioinformatics, 91(12), 1734-1746.
[16] Raouraoua, N., Lensink, M., & Brysbaert, G. (2024). Massive sampling strategy for antibody-antigen targets in CAPRI Round 55 with MassiveFold. Authorea Preprints.
[17] Yin, R., & Pierce, B. G. (2024). Evaluation of AlphaFold antibody–antigen modeling with implications for improving predictive accuracy. Protein Science, 33(1), e4865.
[18] Hitawala, F. N., & Gray, J. J. (2024). What has AlphaFold3 learned about antibody and nanobody docking, and what remains unsolved?. bioRxiv, 2024-09.
[19] Harmalkar, A., Lyskov, S., & Gray, J. J. (2023). Reliable protein-protein docking with AlphaFold, Rosetta, and replica-exchange. bioRxiv.
[20] Gao, M., & Skolnick, J. (2024). Improved deep learning prediction of antigen–antibody interactions. Proceedings of the National Academy of Sciences, 121(41), e2410529121.
[21] Zheng, W., Wuyun, Q., Freddolino, P. L., & Zhang, Y. (2023). Integrating deep learning, threading alignments, and a multi‐MSA strategy for high‐quality protein monomer and complex structure prediction in CASP15. Proteins: Structure, Function, and Bioinformatics, 91(12), 1684-1703.
[22] https://www.biostrand.ai/insight-hub/use-cases , consulted 2024/10/21
[23] Goverde, C. A., Pacesa, M., Goldbach, N., Dornfeld, L. J., Balbi, P. E., Georgeon, S., ... & Correia, B. E. (2024). Computational design of soluble and functional membrane protein analogues. Nature, 1-10.
[24] Dauparas, J., Anishchenko, I., Bennett, N., Bai, H., Ragotte, R. J., Milles, L. F., ... & Baker, D. (2022). Robust deep learning–based protein sequence design using ProteinMPNN. Science, 378(6615), 49-56.
Subscribe to our Blog and get new articles right after publication into your inbox.