Scaling up structural biology with AlphaFold2
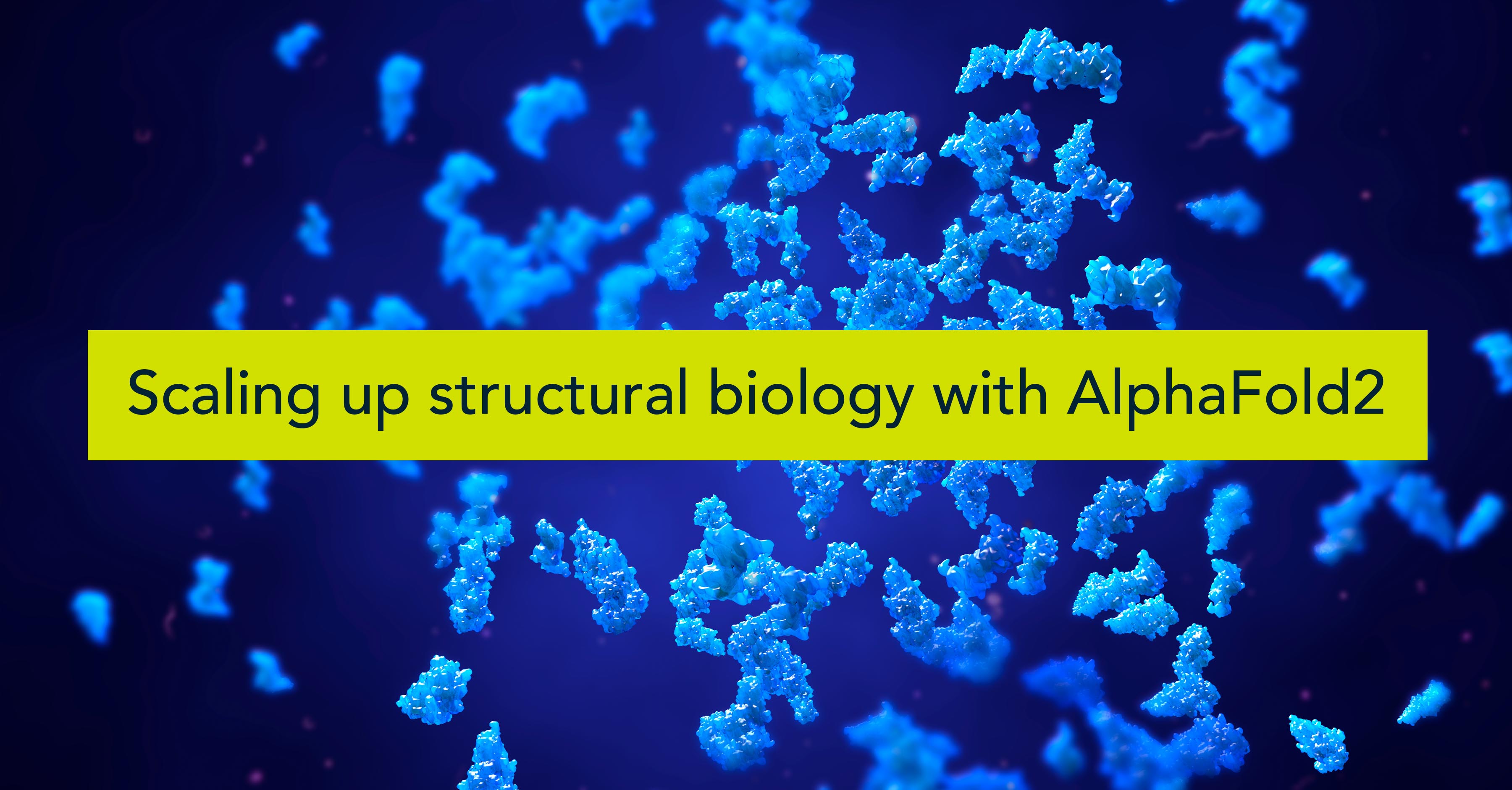
The unveiling of AlphaFold2 during the Critical Assessment of Structure Prediction (CASP) 14th edition has been a turning point in the field of structural biology as a solution to the protein structure problem. This problem is a decades old issue which stem from the observation that a protein structure can be almost uniquely determined by its sequence of amino acid (or primary structure). This implies that there is a general design law, which could be used to model the 3-dimensional structure of proteins if their sequence is known. Despite increasingly more important computer power, such design law has remained unknown. More worrisome, first-principles based methods have historically lacked performance compared to some other template-based methods such as homology modelling, which seemed to go against the empirically observed deterministic nature of protein folding.
With AlphaFold2, DeepMind provided a convincing argument that de novo protein structure prediction is possible, and that a Deep Learning model could somehow capture the aforementioned design law. The performance of AlphaFold2 in contrast its competitors at CASP14 lead to a humongous amount of hype (Fig. 1), with some high figures in the field such as John Moult (co-founder of CASP) claiming that the protein structure prediction problem was solved to some extent [1]. Since then, the AlphaFold2 source code and model weights have been released, which prompted most research groups to incorporate it in their research (Fig. 2), with several possible downstream applications, such as empirical model refinement [4], molecular dynamics, etc. In addition, several Deep Learning-based competitors’ algorithms with comparable performance have appeared, such as RoseTTAFold [5] (Baker Lab) or ESMFold [6] (Meta). Nowadays, there is sufficient hindsight to understand to which extent protein structure prediction has become transformative with respect to how research is performed.
Figure 1 - Interest of AlphaFold over time, measured by Google Trends (21 Feb 2023). The first peak corresponds to the CASP14 conference week, whereas the second peak corresponds to the public release of the model. Adapted from Google Trends.
Figure 2 - Number of citations of the original AlphaFold2 manuscript [3] (to not be confounded with the original AlphaFold paper), measured by Scopus (21 Feb 2023). The number may differ across databases. For instance, Google Scholar repertories 8783 citations (21 Feb 2023). Adapted from Scopus.
Structure Prediction as a routine bio-informatic task
Until recently, the field of bioinformatics revolved mainly around sequence data and annotations. Indeed, with the advent of New Generation Sequencing platforms, sequence data production has been growing exponentially, raising many challenges on data integration and analysis at massive scale. A similar trend has been observed for protein structure, albeit to a much lower scale (Fig. 3). This is due to different reasons, the main one being that empirical studies of protein structure remain too costly to scale up with the availability of sequence data.
Before AlphaFold2, the main method to produce a model in silico was homology modelling, which produced satisfying models based on templates with large sequence similarity. Unfortunately, it meant that all cases where sequence similarity is not high enough would not produce quality model. Moreover, the choice of a good template is critical, which means that such methods cannot be easily automated. On the other hand, AlphaFold2 requires very little input, only the protein sequence(s) which need to be modelled, which facilitate automation. This leads to the release of AlphaFoldDB [7], which now contains more than 200 millions protein structures predicted from sequences stored in the UniProt database. In comparison, at the time of writing (21 Feb 2023), there are only 201,515 entries in PDB [8], a factor of ~1,000 in term of size!
While AlphaFold2 cannot be run from any standard laptop, it can be run on High-Performance Computing (HPC) environment, and scaled up for routine predictions, as we do at BioStrand on our cloud-based setup.
Figure 3 - Number of Released PDB Structures per Year. Adapted from PDB RCSB.
Can predicted structural models be substituted to empirical models?
The prospective of predicting protein structure modeled with precision challenging experimental methods has strong implication for accelerating research. The distribution of structure resolution in PDB shows that most models have a resolution of about 2 Angstroms (Fig. 4). In benchmark such as CASP14, AlphaFold2 demonstrated such capacities, with a mean root-mean square deviation of 1.6 Angstrom on Cα atoms. These early results supported the claim that structure de novo prediction precision reached the boundary of empirical model precision.
Figure 4 - Distribution of structure resolution (in Angstroms), data shown include structures solved by X-ray crystallography or electron microscopy. Adapted from RCSB.
A recent review [9] of the first database of structure predicted by AlphaFold2 (365,198 protein models) highlighted the strengths and limitations of AlphaFold2’s predictions and related output metrics, which give local and non-local confidence score atomic precision (see previous blog post). The authors argue that, for the 11 proteomes covered by the database, an average of 25% additional residues are confidently modelled compared to structures built through homology modelling. These high-confidence regions can be used for downstream modelling tasks (for instance protein-ligand docking). However, not all of AlphaFold2 predictions can be trusted and used for downstream tasks. Roughly 50% of the residues in the database of 11 proteomes are of low confidence (low pLDDT). These residues have been argued to often correspond to intrinsically disordered proteins/regions (IDPs/IDRs). The authors of the paper used AlphaFold2 to benchmark its prediction against other tools to predict IDPs/IDRs and showed that AlphaFold2 outperformed state-of-the-art algorithms such as IUPred2. The authors also compared the AlphaFold2 Multimer to state-of-the-art protein/protein docking algorithm and argue that it also outperformed them for predicting complexes, also confirmed by other groups [10].
One particular domain where AlphaFold2 does not outperform traditional approaches is antibody-antigen docking [10]. This relates to the requirement of co-evolution data used at the beginning of AlphaFold2’s pipeline. Indeed, the antibody-antigen binding strength does not result from co-evolution, but from somatic hypermutation and affinity maturation. Hence, the key component of AlphaFold2’s strength, the Multiple Sequence Alignment (MSA) embedding cannot help for this particular use case. Such shortcomings were also highlighted during the CASP15 conference, which occurred last December in Antalya (Turkey) [11].
The legacy of AlphaFold2
AlphaFold2 is bound to leave a lasting legacy in the field of structural biology. Despite the notable absence of DeepMind at CASP15, the top performing methods incorporated AlphaFold2 as part of the prediction pipeline, and for single domain prediction, it can be expected that improvements will only be substantial from now on. Instead, an increase in protein complexes prediction performance was noted, as various group integrated/hacked through the AlphaFold2 pipeline to predict models which AlphaFold Multimer, the default pipeline for multimeric complex prediction, failed to correctly predict [11].
Around the same period, Meta Fundamental AI Research Protein Team (FAIR) released ESM-2, a Protein Language Model, as well as ESMFold, a protein structure prediction engine built on top of ESM-2. While ESMFold is not as performant as AlphaFold2, it has a notable feature (or lack of): the MSA pre-processing step is missing from the ESMFold pipeline. This MSA rely on a 2 terabytes database scan, which account for most of the runtime of an AlphaFold2 prediction. Instead, ESMFold rely on the information stored in the ESM-2 model weights to produce accurate models. Meta produced over 600 million models, which have been released in the ESM Metagenomic Atlas [12].
The sudden increase in structure prediction performance from AlphaFold2 highlights the remaining challenges for protein structure modelling. The current state-of-the-art methods output static models from sequence input. However, the reality is that protein structures are far from static: part of proteins are less rigid than other and protein motion can be of crucial importance for function. Many proteins can adopt different conformations depending on the context. The protein structure problem formulated as done in the introduction of this blog post suggests that there is a one-to-one mapping between sequence and structure, whereas this is far from being the case, and it highlights our biased thinking toward this simplification. While the PDB database is biased toward single structure model, it still displays heterogeneity in its structural data. In a recent publication [13], Thomas J. Lane (CFEL-PBIO) argues in favor of continuous distribution of protein structure as model, instead of single snapshots. Some particular attention is brought to AlphaFold2’s prediction with respect to its training data: by analyzing the distribution of root-mean squared deviations (RMSD) between PDB models of the SARS-CoV-2 Main Protease (Mpro) and, the distribution of RMSD between AlphaFold2 predictions and these PDB models, it turns out that the distributions overlap, but have distinct peaks. This means on average, that two randomly selected PDB models are more likely to be more similar to each other than to an AlphaFold2 prediction. It is also argued that AlphaFold2 models can lie in between conformational states represented in PDB: an example is given in term of hemoglobin state – unbound, or bound to ligands (such as O2 or CO). The AlphaFold2 structure is shown to lie in between, as some kind of averaged structure which does not correspond to a real stable physical state. Finally, protein domains with low confidence metrics output by AlphaFold2 have also been shown correspond to regions with structural flexibility. This further support the need to move away from the single structure paradigm.
In summary, AlphaFold2 marked a turning point in structural biology, and arguably resolved the single protein structure problem. This leads to a change of focus toward even more complicated challenges, such as the prediction of proteins complexes and interactions. Single protein structure prediction can now be a routine preliminary task for downstream applications and research, for instance, modelling folding mechanisms in molecular dynamics simulation, protein-ligand docking, etc. These downstream applications will be a topic of discussion in this blog, so stay tuned!
References
[1] https://www.deepmind.com/blog/alphafold-a-solution-to-a-50-year-old-grand-challenge-in-biology, accessed 2023/02/21
[2] https://github.com/deepmind/alphafold, accessed 2023/02/21
[3] Jumper, John, Richard Evans, Alexander Pritzel, Tim Green, Michael Figurnov, Olaf Ronneberger, Kathryn Tunyasuvunakool et al. "Highly accurate protein structure prediction with AlphaFold." Nature 596, no. 7873 (2021): 583-589.
[4] Barbarin-Bocahu, Irène, and Marc Graille. "The X-ray crystallography phase problem solved thanks to AlphaFold and RoseTTAFold models: a case-study report." Acta Crystallographica Section D: Structural Biology 78, no. 4 (2022).
[5] Baek, Minkyung, Frank DiMaio, Ivan Anishchenko, Justas Dauparas, Sergey Ovchinnikov, Gyu Rie Lee, Jue Wang et al. "Accurate prediction of protein structures and interactions using a three-track neural network." Science 373, no. 6557 (2021): 871-876.
[6] Lin, Zeming, Halil Akin, Roshan Rao, Brian Hie, Zhongkai Zhu, Wenting Lu, Nikita Smetanin et al. "Evolutionary-scale prediction of atomic level protein structure with a language model." bioRxiv (2022): 2022-07.
[7] https://alphafold.ebi.ac.uk/, accessed 2023/02/21
[8] https://www.rcsb.org/stats/growth/growth-released-structures, accessed 2023/02/21
[9] Akdel, Mehmet, Douglas EV Pires, Eduard Porta Pardo, Jürgen Jänes, Arthur O. Zalevsky, Bálint Mészáros, Patrick Bryant et al. "A structural biology community assessment of AlphaFold2 applications." Nature Structural & Molecular Biology (2022): 1-12.
[10] Yin, Rui, Brandon Y. Feng, Amitabh Varshney, and Brian G. Pierce. "Benchmarking AlphaFold for protein complex modeling reveals accuracy determinants." Protein Science 31, no. 8 (2022): e4379.
[11] Ewen Callaway, “After AlphaFold: protein-folding contest seeks next big breakthrough”, Nature no. 613 (2023), 13-14.
[12] https://esmatlas.com/, accessed 2023/02/21.
[13] Lane, Thomas J. "Protein structure prediction has reached the single-structure frontier." Nature Methods (2023): 1-4.
Subscribe to our Blog and get new articles right after publication into your inbox.