A year in review: Advancing antibody discovery and beyond
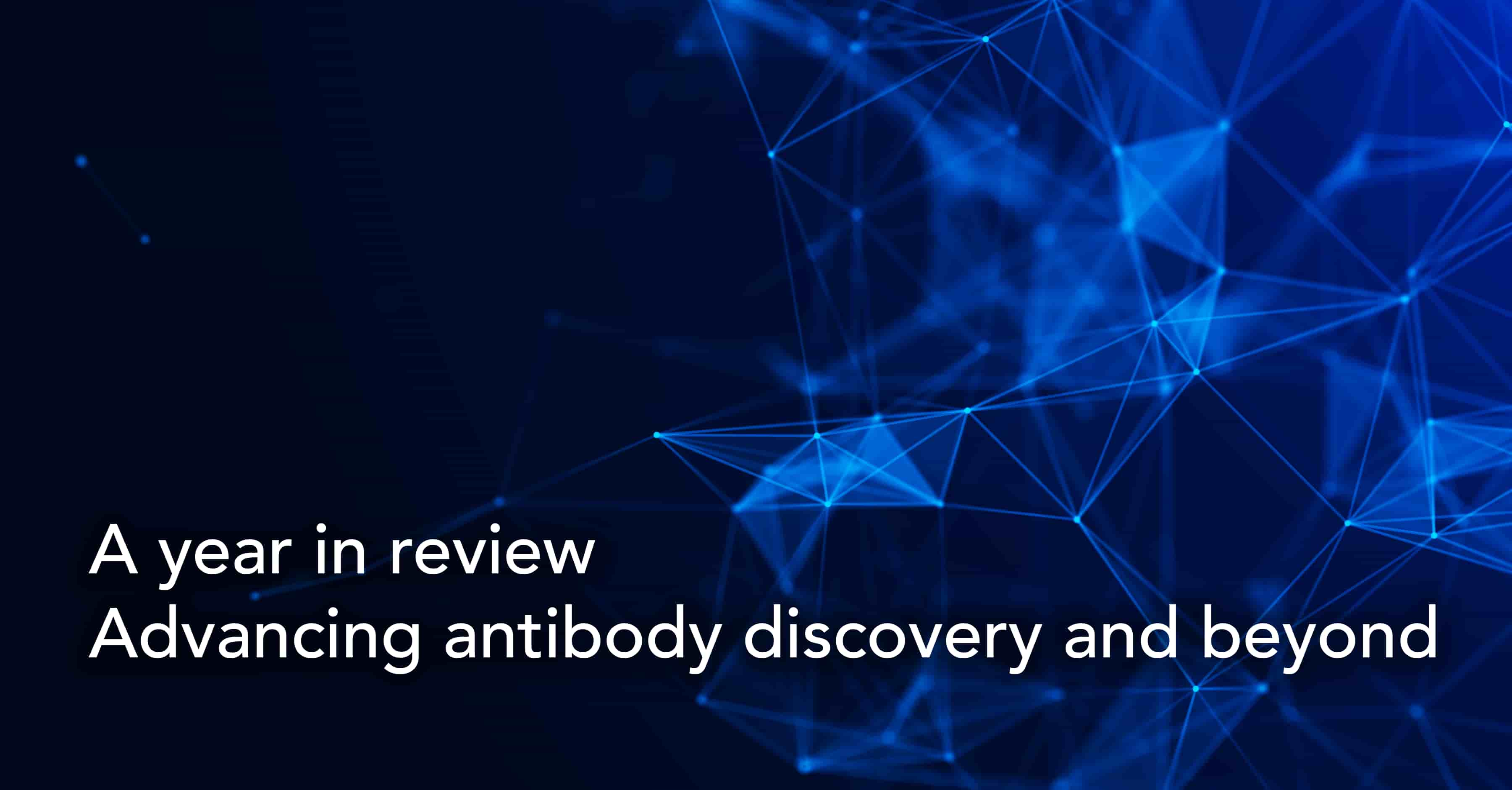
Audio version
As we step into 2025, we’re reflecting on a year full of significant advancements, particularly in enhancing our SaaS platform. These improvements have been instrumental in supporting our antibody discovery and drug development efforts. Here’s a closer look at the progress we’ve made and the exciting direction we’re heading next.
InterSystems award: Recognizing excellence
This recognition, the 2024 Impact Award sponsored by InterSystems, celebrated our LENSai™ technology, chosen from over 1,000 projects for its groundbreaking contributions. Our LENSai demo, which leverages the underlying HYFT-based technology to merge omics data and literature through vector databases, was a true showcase of how our technology can facilitate both the integration of these diverse life science data sources in and be in an application ready format to support analytical pipelines.
BioStrand receives the prestigious 2024 Impact Award for LENSai™ Technology
A year of conferences: Building connections and sharing insights
This year, we participated in both computationally focused AI/ML conferences and antibody-focused events. We shared insights from these events in blogs like PEGS Boston 2024, BioTechX 2024, and PMWC 2024. It was inspiring to witness the growing adoption of AI/ML-driven analyses and to see business cases proving the positive impact of these technologies. We had the pleasure of meeting incredible individuals and showcasing our progress in AI-based antibody engineering. With our unique setup and outstanding results, we solidified our position at the forefront of AI-driven drug discovery companies.
BioStrand team at the BioTechX 2024 conference
TECHday 2024: Showcasing our first public demo of the LENSai SaaS Platform
Welcoming our first users was an absolute highlight of 2024. Our platform is now fully operational, supporting the transfer of data to initiate programs and serving as a single source of truth for exploring project results. Additionally, it’s becoming a launching board for self-service analyses on top of a well-organized data management layer. Feedback from early onboarded users has been invaluable in shaping our 2025 roadmap.
IPA TECHday in Boston
Expanding our capabilities: Expansion of LENSai platform applications
This year, we introduced new pipelines to the LENSai platform, addressing key challenges in antibody discovery and development. Each pipeline is thoughtfully designed to enhance processes like epitope binning, epitope mapping, immunogenicity screening, hit expansion, and large NGS dataset processing. These solutions are built to support scientific rigor and scalability, aligning with the demands of modern research. Here's a closer look at what we’ve launched:
Epitope binning pipeline: cluster antibodies based on epitope recognition and select representative amongst clusters
In the early phase of a discovery campaign, reliably organizing a large pool of (weak) antibody binders to a target is of vital importance for success further down the road. An important way of organizing antibody binders is by grouping them in clusters of antibodies that all bind to a similar antigen region. We solve this problem completely in silico by determining a large set of properties for each antibody, which allows us to measure similarities between each pair of binders. The properties include sequential, structural, chemical and statistical information, providing an as complete as possible characterization for each antibody. Our epitope binning pipeline can be used for sets up to sizes of 10,000 antibodies. Upon completion of the in silico binning , selecting antibodies from each cluster maximizes diversity in terms of target recognition and allows for an efficient reduction of the total amount of binders to test in follow-up assays. A natural in silico assay following epitope binning is epitope mapping, which is also described in this blog post.
Check out our epitope binning use case
Epitope mapping pipeline: Map antibodies to their respective epitope
2024 marked the launch of our epitope mapping pipeline. The pipeline addresses a central question in the drug discovery process: Which residues on a target are engaging in binding with a given antibody? Answering this question allows researchers to get a view on the mode of action of the antibody, which is vital for reaching its desired drug-like properties. Needing only a sequence for the antibody and a structural model for the target, our pipeline combines physics-based modeling and machine-learning approaches to provide the user with a confidence score for each residue in the target protein indicating the likelihood to be part of the epitope. The pipeline is fully automated and generates a report, as well as data files relevant to the problem. The automation allows us to reach timelines of a few hours for small targets (less than 100 amino acids) to at most a few days (for targets of more than a thousand amino acids), which is in stark contrast to similar campaigns in the wet lab. Furthermore, our scalable cloud environment allows us to perform multiple epitope mapping runs in parallel. The epitope mapping pipeline became available through our LENSai portal, allowing a seamless user experience.
Check out our epitope mapping use case
Immunogenicity screening pipeline
It has become more and more evident that continuous screening of immunogenic risks is critical for the overall success of any drug discovery campaign and already starts from the earliest stages on. Throughout this year we have continuously enhanced and improved the scientific model underlying the LENSai Immunogenicity screening pipeline. . We have also enabled the immunogenicity screening of a wider variety of antibody formats such as bi- and multi-specifics as well as general peptides. The pipeline is now accessible via our web portal and we’ve made sure to make user experience as user-friendly and intuitive as possible.
Hit Expansion pipeline: cluster antibodies based on multi-modal properties and suggest potential leads
In antibody discovery and development, selecting the right candidates for further characterization is a critical step and involves identifying the most promising antibodies from a larger pool, often based on specific characteristics such as binding affinity, specificity, and structural diversity. The goal is to optimize spend of resources by focusing on the set of antibodies that are predicted to be the most likely effective therapeutic candidates. A crucial aspect of selecting hits is ensuring diversity within the chosen set of antibodies, which increases the likelihood of finding candidates with unique mechanisms of action, broad reactivity against a target or superior therapeutic properties. Traditional approaches rely on CDR-homology, phylogenetic tree construction, or germline origins – which does not always ensure the preservation of the highest sequence diversity in the selected antibodies. BioStrand’s expansion pipeline takes a multi-modal approach. It allows for the inclusion of sequential and structural alignment components as well as embedding-based characteristics derived from various protein large language models. By using multiple information layers, the likelihood of selecting a highly diverse and functionally relevant set of antibodies increases.
Large NGS dataset processing - preprocessing of NGS data up to 40GB file size
Large NGS datasets can be analyzed fast and easy, including high-throughput reads from NovaSeq. The pipeline provides read alignment, annotation, and enrichment analysis. The repertoire sequencing pipeline delivers fastQC reports to ensure data quality and supports advanced workflows for parsing bulk, UMI-barcoded and single-cell (10x) datasets. It includes customizable settings for quality control, masking steps (to handle primer masking), input formats, and several other technical parameters. The pipeline supports data from different species and offers flexibility for datasets not yet grouped into R1 and R2 paired-end reads. For such cases, the platform provides tools to correctly group data that does not inherently match forward and reverse reads, ensuring accurate downstream analysis. These features make this pipeline highly adaptable, enabling users to tailor workflows to their specific experimental needs while maintaining data integrity and analytical precision.
Molecular dynamics pipeline
Building on these advancements,we have successfully developed a suite of automated end-to-end Molecular Dynamics (MD) simulation pipelines, optimized for high-performance GPU instances. These pipelines enable detailed exploration of the dynamic behavior of proteins at the molecular level in near-physiological environments. They support a diverse range of protein complexes, including multi-chain proteins, antigen-antibody complexes, glycosylated proteins, protein-ligand complexes, and membrane proteins embedded in explicit lipid membrane environments. Additionally, the pipelines generate comprehensive outputs, including key MD trajectory statistics and interaction analyses such as RMSD, RMSF, contact numbers, contact maps, binding energies, pairwise and per-residue energy decompositions, providing valuable insights to end users. Notably, we have implemented an advanced Constant pH MD simulation workflow, enabling the study of pH-dependent biomolecular behavior and interactions in solution—a critical aspect of many biological processes. Our automated MD protocols are invaluable for refining empirical and predicted biomolecular models and complexes, offering in-depth insights into their energetic and dynamic properties. These tools significantly enhance our understanding of biological mechanisms and contribute to the acceleration of antibody and drug design.
Collaborative growth: Partnering with Intel
At BioStrand, we are dedicated to continuously optimizing our pipelines. This involves evaluating multiple cloud providers and seeking the fastest hardware solutions. To further this mission, BioStrand joined the Intel Liftoff program. This initiative provides companies with access to advanced computational resources to tackle their most critical technical challenges. Through Intel Liftoff, we benefit from resources like the Intel Tiber AI Cloud and Intel’s comprehensive software portfolio, which features next-generation AI hardware.
Our efforts extend beyond hardware; we’re also refining our code to run more efficiently and effectively on designated platforms. Collaborating closely with the Intel labs team, we are enhancing our internal pipelines for peak performance.
Looking ahead to 2025, expect even faster pipelines and significant cost savings. Stay tuned for the next wave of innovation at BioStrand!
Closing thoughts and looking ahead
Looking ahead into 2025, we want to thank everyone who collaborated with us in 2024. It’s been a year of growth and milestones for our LENSai platform. Heading into 2025, we’re focused on building momentum and delivering even more value. Our focus remains on continuously improving the discovery and engineering of antibodies through user-friendly in silico tools. Additionally, we are expanding our analytical portfolio to include de-novo and generative capabilities. We’re excited for what’s to come —stay tuned for updates and new developments as we continue driving progress.
BioStrand team in BioVille
Subscribe to our Blog and get new articles right after publication into your inbox.