Cracking the Information Integration Dilemma (IID) in systems biology
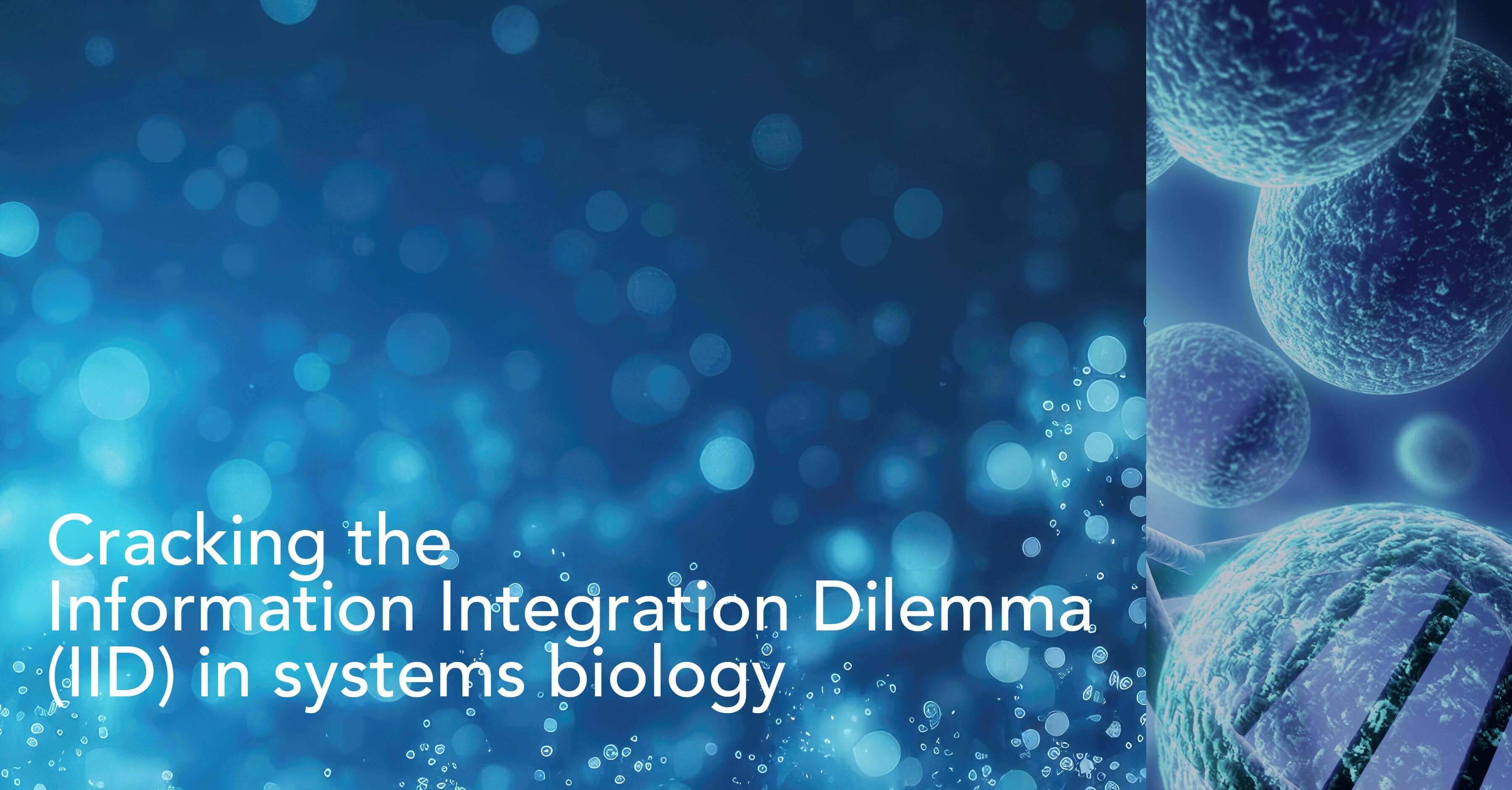
In our new LENSai blog series, we explore how data itself often becomes the bottleneck in data-driven biological and biomedical research. We dive into the data-related challenges that affect the development and advancement of different research concepts and domains, such as drug discovery, and also the importance of integrating wet lab and in silico research etc.
We start with systems biology, a holistic model that represents a radical departure from the conventional reductionist approach to understanding complex biological systems.
Biological and biomedical research in the 20th century was driven predominantly by reductionism, a pieces of life approach that seeks to understand complex biological systems as a sum of the functionalities of their individual components. Now, there is definitely value in building a systems-level perspective that is based on an aggregation of component-level functionality. After all, reductionism has played a key role in elucidating the central dogmatic principles and concepts of biology.
However, the limitations of this approach are hard to ignore. After all, a complex biological system, unlike, say, a bicycle, clearly has to be more than a sum of its parts. Systems biology is the paradigm that defines an integrative and holistic strategy to decipher complex, hierarchical, adaptive, and dynamic biological systems across multiple components and levels of organization. Complex biological systems, like those within living organisms, are much more intricate than simple objects like bicycles. Unlike bicycles, these systems are not just a sum of their parts but have unique properties that emerge when all of the parts work together.
Systems biology is an approach that helps scientists study and understand these complex biological systems by looking at the big picture. By considering how all the different parts of the system interact, scientists get a better understanding of how the entire system functions as a whole, instead of only looking at individual components in isolation.
Inspired by the ideas from the Santa Fe Institute, system thinking plays a crucial role in the systems biology approach. It helps researchers recognize the importance of the connections between different parts of the biological system, the influence of its surroundings, and how the system changes over time. This way, scientists can better understand health, disease, and potential treatments, leading to more effective medical therapies and diagnostic tools.
The modern form of systems biology emerged in the late 1960s and it quickly became evident that mathematics and computation would play a critical role in realizing the potential of this holistic approach. Mathematical and computational modeling based on large volumes of genome-scale data would be the key to unraveling the systems-level complexity of biological phenomena.
Today, the availability of sophisticated computational techniques and the exponential generation of high-throughput biomedical data provide the perfect foundation for a systems approach to tackling biological complexity.
But here’s where things get a bit complicated.
Complex biological phenomena and systems are defined by complex biological data. A data-driven systems approach requires the integrated analysis of all available complex biological data in order to identify relevant interactions and patterns of a biosystem. However, the sheer complexity of biological data poses a major challenge for efficient data integration and curation that is required for generating a holistic view of complex biological systems.
A quick overview of biological data complexity
The James Webb Space Telescope generates up to 57 gigabytes each day. By comparison, one of the world’s largest genome sequencing facilities sequences DNA at a rate equivalent to a human genome, roughly 140 gigabytes in size, every 3.2 minutes. And that is just genomic data, which is expected to reach exabase-scale within a decade, from just one sequencing facility.
Despite the continuing exponential increase of publicly-available biological data, data volume is perhaps one of the more manageable complexities of biological big data. Then there’s the expanding landscape of biological data, from single-cell omics data to genome-scale metabolic models (GEMs), that reflect the inherent complexity and heterogeneity of biological systems and vary in format, and scale. Data formats can also vary based on the technologies and protocols used to characterize different levels of biological organization. From a data integration perspective, there also has to be due consideration for organizing structured and unstructured data as well as multi-format data from numerous databases that specialize in specific modalities, layers, organisms, etc.
Over and above all this, novel complexities continue to emerge as technological advancements open up new frontiers for biological research. Moving on from simple static models derived from static data, the scope of research is now expanding to characterize biological complexity along the dynamic fourth dimension of time. For instance, rather than merely integrating single-time-point omics sequence data across biological levels, the emerging framework of temporal omics compares sequence data across time in order to evaluate the temporal dynamics of biological processes.
So the big question is how to integrate, standardize, and curate all this complexity into one comprehensive, contextual, scalable data matrix that solves the Information Integration Dilemma in systems biology.
The LENSai Integrated Intelligence Platform for Systems Biology
Information Integration Dilemma (IID) refers to how the challenges of integrating, standardizing, and analyzing complex biological data have created a bottleneck in the holistic, systems-level analysis of biological complexity. Currently integrating data, across diverse data modalities, formats, platforms, standards, ontologies, etc., for systems biology data analysis is not a trivial task. The process requires multiple tools and techniques for different tasks such as harmonizing and standardizing data formats, preprocessing, integration, and fusion. Moreover, there is no single analytical framework that scales across the complex heterogeneity and diversity of biological data.
The LENSai Integrated Intelligence Platform addresses these shortcomings of conventional solutions by incorporating the key organizing principles of intelligent data management and smart big data systems.
One, the platform leverages AI-powered intelligent automation to organize and index all biological data, both structured and unstructured. HYFT®, a proprietary framework that leverages advanced machine learning (ML) and natural language processing (NLP) technologies, seamlessly integrates and organizes all biological and textual data into a unified multidimensional network of data objects. The network currently comprises over 660 million data objects with multiple layers of information about sequence, syntax, and protein structure. Plus, HYFT® enables researchers to integrate proprietary research into the existing data network. This network is continuously updated with new data, metadata, relationships, and links, ensuring that the LENSai data biosphere is always current.
Two, smart big data is not just about the number of data objects but also about latent relationships between those data sets. The LENSai data biosphere is further augmented by a knowledge graph that currently maps over 25 billion cross-data relationships and makes it easier to visualize the interrelatedness of different entities. This visual relationship map is continuously updated with contextual biological information to create a constantly expanding knowledge resource.
Now that we have an organized, high-quality, contextualized data catalog, the next step is to provide comprehensive search and access capabilities that empower users to curate, customize and organize data sets to specific research requirements. For instance, the computational modeling of biological systems could follow two broad research directions — bottom-up theory-driven modeling, based on contextual links between model terms and known mechanisms of a biological system, or two, top-down data-driven modeling, where relationships between different variables in biological systems are extracted from large volumes of data without prior knowledge of underlying mechanisms. So, an intelligent data catalog must enable even non-technical users to organize and manipulate data in a way that best serves their research interests.
Multiscale Data Integration with the LENSai Platform
Biological systems operate across multiple and diverse spatiotemporal scales, with each represented by datasets with very diverse modalities. The systems biology approach requires the concurrent integration of all of these multimodal datasets into one unified analytical framework in order to obtain an accurate, systems-level simulation of biological complexity. However, there are currently no bioinformatics frameworks that facilitate the multiscale integration of vast volumes of complex, heterogeneous, system-wide biological data.
But BioStrand’s patented HYFT® technology and LENSai platform enable true multiscale data unification — including syntactical (sequence) data, 3D structural data, unstructured scientific information (e.g. scientific literature), etc. — into one integrated, AI-powered analytical framework. By completely eliminating the friction in the integration of complex biological data, LENSai shifts the paradigm in data-driven biological research.
Subscribe to our Blog and get new articles right after publication into your inbox.