Closing the Gap of Text and the Biosphere with LENSai™
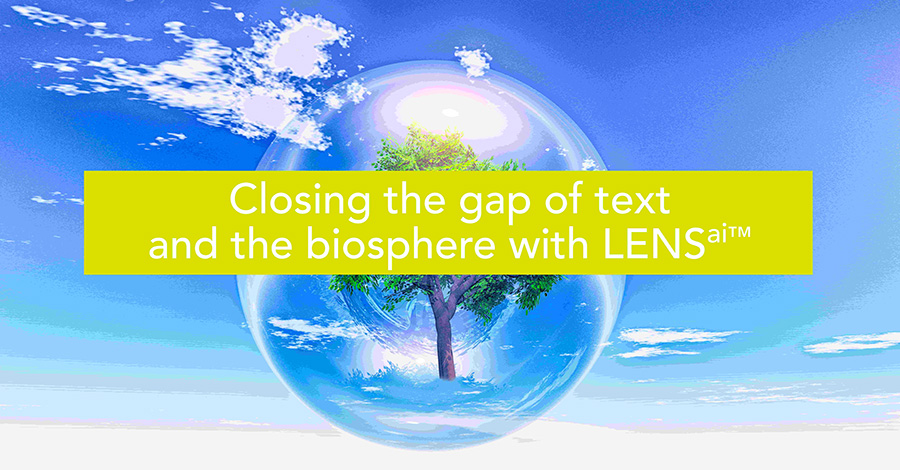
Bridging the gap between raw sequence data and the accumulated knowledge embedded in scientific literature and other textual data sources is one of the most significant barriers to integrated multi-omics analytics.
The distinction is often made between structured and unstructured omics data, where structured data is that which can be processed with standard computer algorithms. But the reality is that all omics data is unstructured – just to different degrees. For instance, raw reads can be considered unstructured data as they need to be mapped to known references before they are ready for any meaningful analysis. Data from secondary research is typically unstructured as it is derived from a variety of technologies, rendered in a diversity of types and formats, and stored across distributed data repositories. Researchers depend on skilled bioinformaticians to integrate these data. Data from scientific literature and other text-based information sources is, of course, unstructured data. These are data that are often ignored because most conventional analytical tools cannot handle textual data.
With LENSᵃⁱ™ Integrated Intelligence Platform, our mission is to help bridge the data analytics gap by building the next generation of technology to bring structure to all omics data. To do this, we designed a revolutionary solution to encode omics data centered around HYFT® patterns. HYFT® patterns provide a universal framework to organize heterogeneous pools of omics data. Each HYFT serves as a data object enriched with DNA, RNA, and amino acid data. More importantly, each HYFT is also embedded with metadata comprising high-level information about position, function, structure, cell type, species, pathogenicity, pathway, Mechanism of Action (MoA) role, etc. All HYFTs are connected in a network of data objects to provide information on the whole biosphere. It is this network of HYFT that functions as the data foundation for the LENSai platform.
What Google was to the internet, the Lensai solution is to the biosphere, streaming access to vast pools of heterogeneous data. LENSᵃⁱ organizes the entire biosphere as a multidimensional network of 660 million data objects integrating layers of multi-omics information – including data about sequence, syntax, and protein structure. With LENSᵃⁱ, researchers can now seamlessly access all raw sequence data as well as secondary data from across public and proprietary data repositories.
The next big opportunity is to augment the volumes of structural information embedded in the Lensai biosphere by integrating unstructured data from text-based knowledge sources such as scientific journals, electronic health records (EHRs), clinical notes, etc. Combining structured sequence data and metadata with unstructured text data can significantly amplify the efficiency and productivity of drug discovery and development processes. For example, integrating unstructured data can elevate preclinical development in terms of target deconvolution, biological risk assessment, information streaming for target identification, lead selection, IP analysis, interaction screening, and determination of MoA during lead selection.
LENSai for unstructured text data
Our innovative natural language processing (NLP )solution focuses on text mining and analysis to link knowledge and insights from text-based information sources, like biomedical literature, to the sequence data. This integrated data-driven model enables the real-time integration and analysis of petabytes of all research-relevant structured and unstructured data to accelerate discovery.
However, when it comes to biomedical NLP, it is not as simple as training general-purpose NLP solutions on specialist literature or clinical notes. BioNLP solutions have to be purpose-built for this specific domain, with a focus on certain high-level requirements.
High-level requirements for a BioNLP pipeline
A typical NLP pipeline in drug discovery and development comprises pre-processing methodologies, like tokenization, lemmatization, etc., combined with a selection of NLP functionalities, like Named Entity Recognition (NER), relation extraction etc. Even though BioNLP configurations can diverge, based on objectives and applications, the general workflow is as follows.
Ingesting text from diverse document types
Unstructured text data can come in various formats – for instance, as text encoded in an HTML or pdf document, or even embedded within images. BioNLP solutions must be capable of normalizing different types of text input so they can all be processed in the same manner.
Resolving conceptual entities
This task is essentially about finding the right word boundaries to define concepts in a sentence and to determine which bits of information are relevant. The fundamental approach here is to chunk sentences into words that represent a specific concept within that sentence. For instance, the statement "Two patients are suffering from congestive heart failure" has two different concepts. The first is "two patients" – a concept that is distinct from "one patient" or the indeterminate "patients". Similarly, the second concept is "congestive heart failure" as opposed to "congestive arthritis" or just "heart failure", which are both completely different.
Classifying entities
Once the right word boundaries have been obtained, each entity – i.e., word or combination of words – has to be mapped to its meaning. There are different approaches to classifying entities. It is possible to map each entity to dictionaries or ontologies containing all classified entities in order to extract their meaning. Another approach is through Named Entity Recognition (NER) which is typically done through machine learning models that can classify this concept through a neural network.
Extracting relations between entities
Once the entities have been classified, the next requirement is to understand the relationship between different conceptual entities. For instance, the relationship between the concepts "two patients" and "congestive heart failure" is "are suffering from". This can be approached as a classifying task relying either on pre-defined relations and labels, or on rules and heuristics.
The first approach requires annotated data based on an advanced understanding of all possible relations that will be found in text. The obvious limitation of this approach is that any new relation that emerge in the text will not be recognized by ML algorithms. The second approach tends to be more efficient but can lead to noisy data. Additionally, there are hybrid approaches that combine both these methods.
Integrating processed data within a queryable knowledge base
All the data extracted in the ingestion process must then be integrated within a queryable knowledge base. This means that the structure of this knowledge base has to be planned and defined in advance – based on the nature of downstream processing tasks.
Apart from these broad conceptual requirements, there are also the underlying models, algorithms, and architectures that power many of these tasks and which have evolved significantly over the years. Machine Learning (ML) approaches have been central to NLP development. By 2017, Recurrent Neural Networks were considered state-of-the-art for NLP tasks. Today, Transformers have become the dominant choice for NLP – indeed, most of the predominant language models for NLP, such as BERT, GPT-3, and XLNet are Transformer-based.
The BioStrand NLP offering
LENSᵃⁱ is a comprehensive pipeline for data ingestion and knowledge extraction for a range of BioNLP tasks.
Our NLP ingestion pipeline automates the ingestion and normalization of all types of biomedical text data. The solution also integrates dictionary matching with multiple dictionaries, including SNOMED, UMLS, and HUGO, to enable the mapping of concepts extracted from the ingestion pipeline.
The integration of Named Entity Recognition and Named Entity Normalisation allows researchers to assign both class and entity using deep learning approaches. A relation extraction plus relation normalization mechanism transforms unstructured text data into structured data, which is then stored within the queryable knowledge base.
Our unique approach to data ingestion means that out-of-the-box researchers have ready-to-use access to a queryable knowledge base containing over 33 million abstracts from the PubMed biomedical literature database. More importantly, the BioStrand solution will enable researchers to expand this core knowledge base by directly integrating data from multiple sources and knowledge domains, including proprietary databases. For instance, researchers can easily upload proprietary text documents, including licensed documents not intended for public consumption, to construct an NLP knowledge base that is unique to their research objectives. The focus at BioStrand, therefore, is to provide a comprehensive and easy-to-use set of tools and functions that will allow researchers to seamlessly ingest any textual data that can extend their research.
And finally, LENSᵃⁱ offers integrated graph extractors that serve as an information extraction pipeline. These graph extractors allow researchers to extract semantic and proximity graphs from the knowledge base. Users can focus their extraction queries using a number of different parameters and the resulting graphs can then be exported to Cytoscape. As a result, our platform is not just a solution for downstream processing but a powerful tool for literature exploration.
The BioStrand HYFTs + NLP advantage
At BioStrand we have developed a unique bottom-up approach to bring meaning to data at the sequence and text level. Our HYFTs innovation enables frictionless data integration of all omics sequence and metadata from across the biosphere. With our platform, we offer a specialized BioNLP solution that streamlines data ingestion and accelerates knowledge extraction. Considered together, our solutions provide access to compute-ready structured omics data covering over 400 million sequences with 33 million documents.
With LENSᵃⁱ and the HYFT network, researchers have access to the entire biosphere organized as a multidimensional, multimillion-node network integrating transversal multi-omics information. With the BioStrand platform researchers can now integrate sequence and text and use advanced AI/ML technologies to explore the connection between structured omics data and textual omics knowledge. Our solution gives researchers direct access to integrated sources from multiple knowledge domains with easy-to-use functions to extend their research.
Subscribe to our Blog and get new articles right after publication into your inbox.