Creating a unified data + information architecture for scalable AI
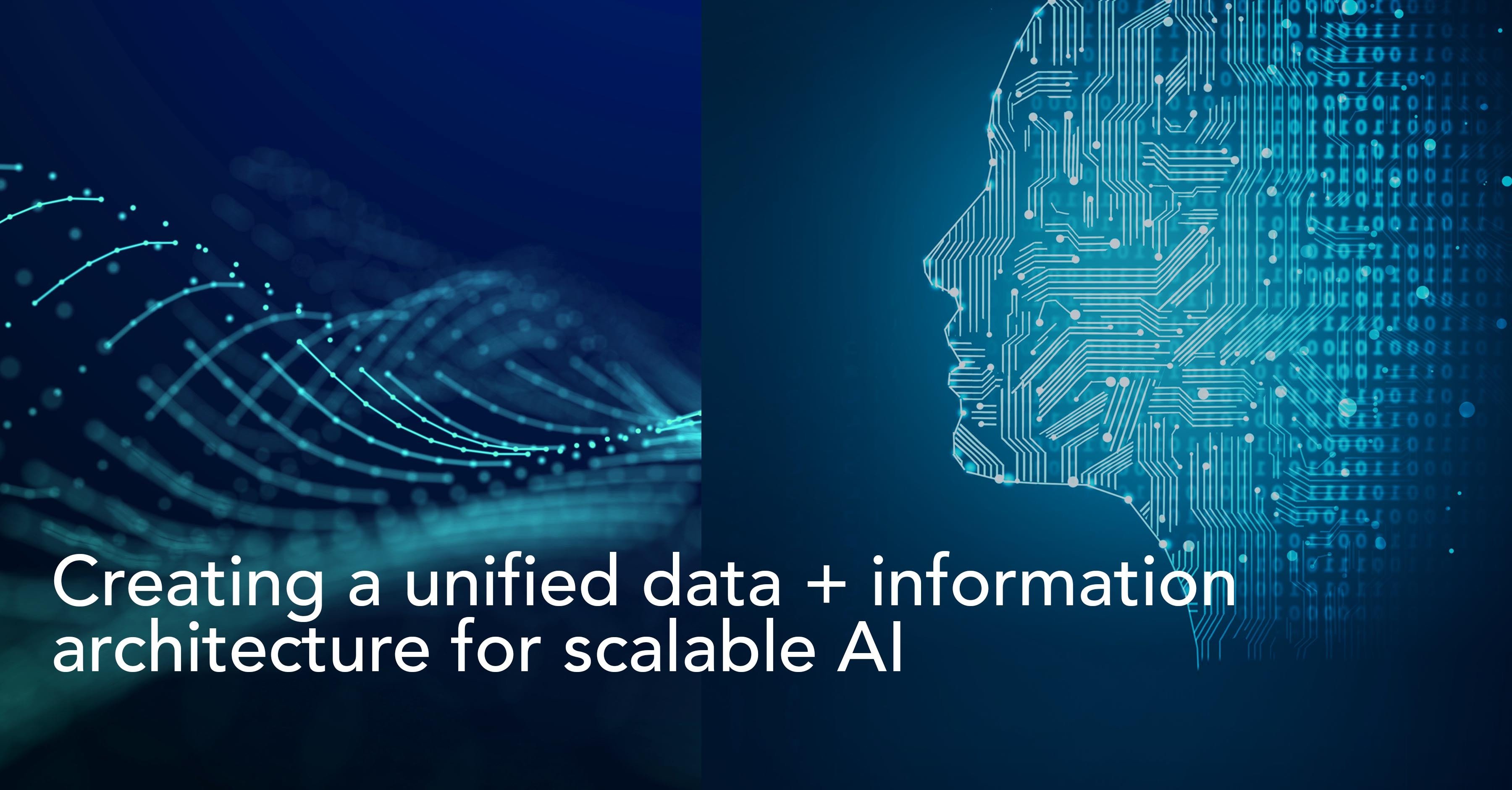
The first blog in our series on data, information and knowledge management in the life sciences, provided an overview of some of the most commonly used data and information frameworks today.
In this second blog, we will take a quick look at the data-information-knowledge continuum and the importance of creating a unified data + information architecture that can support scalable AI deployments.
In 2000, a seminal knowledge management article, excerpted from the book Working Knowledge: How Organizations Manage What They Know, noted that despite the distinction between the terms data, information, and knowledge being just a matter of degree, understanding that distinction could be key to organizational success and failure.
The distinction itself is quite straightforward, Data refers to a set of discrete, objective facts with little intrinsic relevance or purpose and provide no sustainable basis for action. Data endowed with relevance and purpose becomes information that can influence judgment and behavior. And knowledge, which includes higher-order concepts such as wisdom and insight, is derived from information and enables decisions and actions.
Today, in the age of Big Data, AI (Artificial Intelligence), and the data-driven enterprise, the exponential increase in data volume and complexity has resulted in a rise in information gaps due to the inability to turn raw data into actionable information at scale. And the bigger the pile of data, the more the prevalence of valuable but not yet useful data.
The information gap in life sciences
The overwhelming nature of life sciences data typically expressed in exabase-scales, exabytes, zettabytes, or even yottabytes, and the imperative to convert this data deluge into information has resulted in the industry channeling nearly half of its technology investments into three analytics-related technologies — applied AI, industrialized ML (Machine Learning), and cloud and edge computing. At the same time, the key challenges in scaling analytics, according to life sciences leaders, were the lack of high-quality data sources and data integration.
Data integration is a key component of a successful enterprise information management (EIM) strategy. However, data professionals spend an estimated 80 percent of their time on data preparation, thereby significantly slowing down the data-insight-action journey. Creating the right data and information infrastructure (IA), therefore, will be critical to implementing, operationalizing, and scaling AI. Or as it’s commonly articulated, No AI Without IA.
The right IA for AI
Information and data architectures share a symbiotic relationship in that the former accounts for organization structure, business strategy, and user information requirements while the latter provides the framework required to process data into information. Together, they are the blueprints for an enterprise’s approach to designing, implementing, and managing a data strategy.
The fundamental reasoning of the No AI Without IA theorem is that AI requires machine learning, machine learning requires analytics, and analytics requires the right IA. Not accidental IA, a patchwork of piecemeal efforts to architect information or traditional IA, a framework designed for legacy technology, but a modern and open IA that creates a trusted, enterprise-level foundation to deploy and operationalize sustainable AI/ML across the organization.
AI information architecture can be defined in terms of six layers: data sources, source data access, data preparation and quality, analytics and AI, deployment and operationalization, and information governance and information catalog. Some of the key capabilities of this architecture include support for the exchange of insights between AI models across IT platforms, business systems, and traditional reporting tools. Empowering users to develop and manage new AI artifacts, managing cataloging and governance of these artifacts, and promoting collaboration. And ensuring model accuracy and precision across the AI lifecycle.
An IA-first approach to operationalizing AI at scale
The IA-first approach to AI starts with creating a solid data foundation that facilitates the collection and storage of raw data from different perspectives and paradigms including batch collection and streaming data, structured and unstructured data, transactional and analytical data, etc. For life sciences companies, a modern IA infrastructure will address the top hurdle in scaling AI, i.e. the lack of high-quality data sources, time wasted on data preparation, and data integration. Creating a unified architectural foundation to delay with life sciences big data will have a transformative impact on all downstream analytics.
The next step is to make all this data business-ready and data governance plays a critical role in building the trust and transparency required to operationalize AI. In the life sciences, this includes ensuring that all data is properly protected and stored from acquisition to archival, ensuring the quality of data and metadata, engineering data for consumption, and creating standards and policies for data access and sharing. A unified data catalog that conforms to the information architecture will be key to enabling data management, data governance, and query optimization at scale.
Now that the data is business-ready, organizations can turn their focus to executing the full AI lifecycle. The availability of trusted data opens up additional opportunities for prediction, automation, and optimization plus prediction capabilities. In addition, people, processes, tools, and culture will also play a key role in scaling AI. The first step is to streamline AI processes with MLOps to standardize and streamline the ML lifecycle and create a unified framework for AI development and operationalization. Organizations must then choose the right tools and platforms, from a highly fragmented ecosystem, to build robust, repeatable workflows, with an emphasis on collaboration, speed, and safety. Scaling AI will then require the creation of multidisciplinary teams organized as a Center of Excellence (COE) with management and governance oversight, as decentralized product, function or business unit teams with domain experts, or as a hybrid. And finally, culture is often the biggest impediment to AI adoption at scale and therefore needs the right investments in AI-ready cultural characteristics.
However, deployment activity alone is not a guarantee for results with Deloitte reporting that despite accelerating full-scale deployments outcomes are still lagging. The key to successfully scaling AI is to correlate technical performance with business KPIs and outcomes. Successful at-scale AI deployments are more likely to have adopted leading practices, like enterprise-wide platforms for AI model and application development, documented data governance and MLOps procedures, and ROI metrics for deployed models and applications. Such deployments also deliver the strongest AI outcomes measured in revenue-generating results such as expansion into new segments and markets, creation of new products/services, and implementation of new business/service models.
The success of AI depends on IA
One contemporary interpretation of Conway's Law argues that the outcomes delivered by AI/ML deployments can only be as good as their underlying enterprise information architecture. The characteristics and limitations of, say, fragmented or legacy IA will inevitably be reflected in the performance and value of enterprise AI. A modern, open, and flexible enterprise information architecture is therefore crucial for the successful deployment of scalable, high-outcome, future-proof AI. And this architecture will be defined by a solid data foundation to transform and integrate all data, an information architecture that ensures data quality and data governance and a unified framework to standardize and streamline the AI/ML lifecycle and enable AI development and operationalization at scale.
In the next blog in this series, we will look at how data architectures have evolved over time, discuss different approaches, such as ETL, ELT, Lambda, Kappa, Data Mesh, etc., define some hyped concepts like ‘big data’ and ‘data lakes’ and correlate all this to the context of drug discovery and development.