The importance of NLP in biomedical research
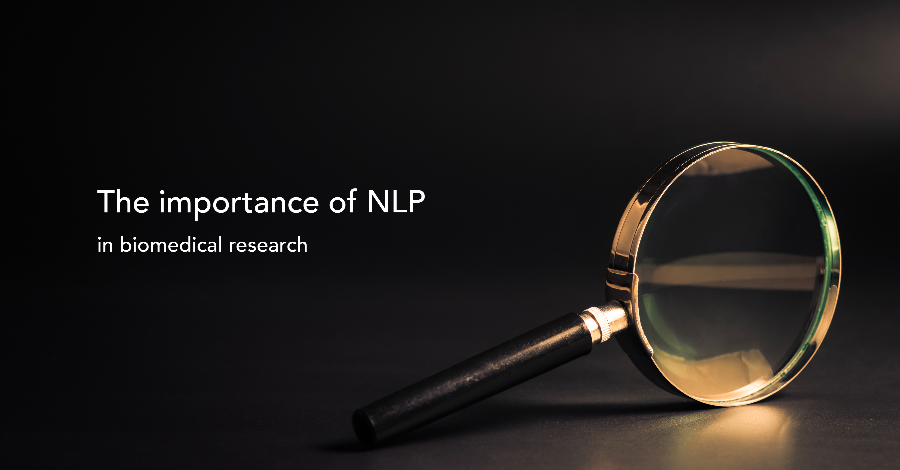
There will be more than twice as much digital data created over the next five years as has been generated since the advent of digital storage. And a vast majority of that data, more than 80 per cent, will be unstructured and estimated to be growing at 55-65% per year.
Textual data, in the form of documents, journal articles, blogs, emails, electronic health records and social media posts, is one of the most common types of unstructured data. This is where AI-based technologies like NLP, can help extract meaning and context from large volumes of unstructured textual data.
NLP unlocks access to valuable new data sources that were hitherto beyond the purview of conventional data integration and analysis frameworks. Biomedical-domain-specific NLP techniques open up a gamut of possibilities in automating the extraction of statistical and biological information from large volumes of text including scientific literature and medical/clinical data.
More importantly, they bring several new benefits in terms of productivity, efficiency, performance and innovation.
Key benefits of NLP
Enabling scale, across multiple dimensions
Scientific journals and other specialized online publications are critical to the dissemination of experiments and studies in biomedical and life sciences research. Every biomedical research project can benefit significantly from extracting relevant scientific knowledge, like protein-protein interactions, for example, embedded in this distributed information trove.
And with an estimated 3000 biomedical articles being published every day, NLP becomes an indispensable tool for the collation and propagation of knowledge. It is a similar situation in the clinical context, where NLP can quickly extract meaning and context from a sprawl of unstructured text records such as EHRs, diagnostic reports, medical notes, lab data etc.
NLP methods have also been successfully reimagined to scale across structured biological information like sequence data.
Today, high-throughput sequencing technologies are generating more biological sequence data that still lack interpretation or biological information. This creates a major integration and analysis bottleneck for conventional downstream frameworks.
For instance, at BioStrand we have applied NLP methods to transcribe the universal language of all omics data and develop a unified framework that can instantly scale across all omics data.
Uncovering new actionable insights
Using NLP to expand the scope of biomedical research to textual data can lead to the discovery of insights that lie outside the realm of clinical and biological data.
In the clinical context, for example, effective patient-physician communication is vital for enhancing patient understanding of treatment and adherence in order to improve clinical outcomes and patient quality of life. And patient-reported outcome measures (PROMs) are often used to assess and improve communication.
However, one study set out to complement conventional approaches by extracting a patient-centred view of diseases and treatments through social media analytics. The strategy was to use a text-mining methodology to analyse health-related forums to understand the therapeutic experience of patients affected by hypothyroidism and to detect possible adverse drug reactions (ADRs) that may not necessarily be communicated in the formal clinical setting.
The analysis of reported ADRs revealed that a pattern of well-known side effects uncertainties about proper administration was causing anxiety and fear. The other key finding was that some reported symptoms quite frequently posted online, like dizziness, memory impairment, and sexual dysfunction were usually not discussed at in-person consultations.
Empowering researchers, accelerating research
NLP technologies significantly expand the scope and potential of biological research by putting into play vast volumes of information that were hitherto underutilised. By automating the analysis of unstructured textual data, it empowers researchers with more data points to explore more correlations and possibilities.
In addition, it relieves them from tedious, repetitive tasks thereby allowing them to focus on activities that add real value and accelerate time-to-insight.
Take rare disease drug development, for example, a field characterised by small patient populations and a shortage of data. To account for the inherent data scarcity, researchers had to manually scour through large volumes of information to identify any links between rare diseases and specific genes and gene variants.
The advent of NLP relieves researchers from the tedium of manual search, instantly expands their data universe and helps accelerate the drug development process for rare diseases.
Enabling innovation
NLP can help disrupt and reinvent tried and tested processes that have become part of the established convention in many industries.
Take biological research, for example, where sequence search and comparison is the launch point for a lot of projects. In this standard process, users typically input a research-relevant biological sequence, in a predefined and acceptable data format, and use relevant search results to chart their research pathway.
Even though the underlying frameworks, models and algorithms have evolved considerably over the years, the standard process still remains the same; users input a sequence to obtain a list of all pertinent sequences.
However, NLP-based innovations, like the BioStrand Platform, for example, can completely disrupt this process to yield significant improvements in efficiency, productivity and performance. In the NLP-based model, users can start with a simple text input, say COVID, to launch their search.
More importantly, the model surfaces all relevant results, both at the sequence and text levels, thereby facilitating a more data-inclusive and integrative approach to genomics research.
Integrative research with BioStrand
BioStrand Platform is our latest technology innovation in our continuing quest to make omics research more efficient, productive and integrative. By adding literature analysis to our existing omics and metadata integration framework, we now offer a unified solution that scales across sequence data and unstructured textual data to facilitate a truly integrative and data-driven approach to biological research.
Our platform's semantics-driven analysis framework is fully domain-agnostic and uses a bottom-up approach which means that even proprietary literature with custom words can be easily parsed.
Our integrated framework traverses omics data, metadata and textual data to capture all correlated information across structured and unstructured data at one shot. This provides researchers with a ‘single pane of glass’ view of all entities, associations and relationships that are relevant to their research.
And we believe that enabling this singular focus on all the most relevant data points and correlations that exist between a specific research purpose and all prior knowledge can help researchers significantly accelerate time to insight and value.