The transformative power of text in multi-omics analysis
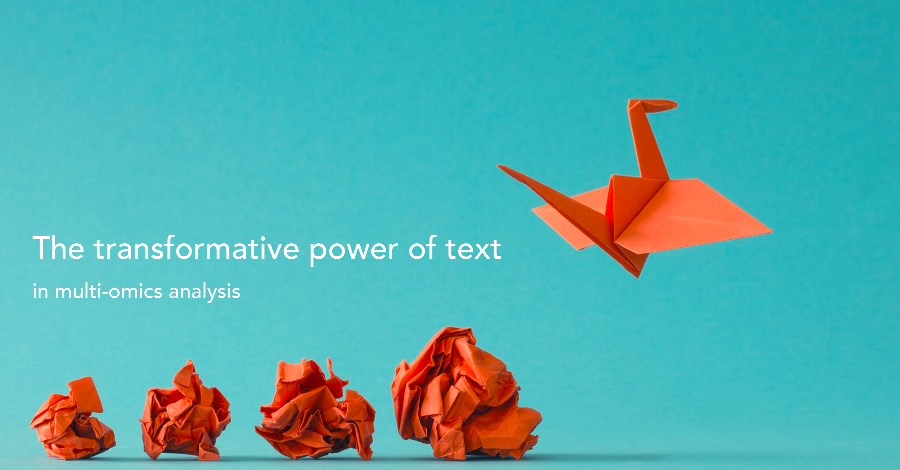
Sequence comparison, a fundamental and critical component of modern molecular biological research, has evolved tremendously since the emergence of alignment-based methods towards the end of the last century.
Today, alignment-free (AF) sequence comparison has become indispensable for data-intensive applications in next-generation sequencing data processing and analysis.
Traditional methods such as dynamic programming and heuristics have also been succeeded by techniques derived from modern computer science – like optimisation and indexing, for instance – that enable a faster, scalable, more accurate, and less resource-intensive approach to sequence retrieval and comparison.
One aspect of sequence search and comparison, however, has not changed much at all – a biological sequence in a predefined and acceptable data format is still the primary input in most research. This approach is probably and arguably valid in many if not most real-world research scenarios.
However, what if your research hypothesis stems from a broader, objective context unconstrained by the predetermined relevance of a particular biological sequence? What if you could input that context as a free text search and use the results to determine the molecular level, sequence, and pathway best aligned with your research interests?
Text-based contextual sequence search
Text-based sequence search enables users to launch their research on the basis of a search string that describes a specific but purely contextual perspective, or a general exploratory hypothesis related to a particular biological domain, phenomenon, or function. The text search generates all the sequence data that are related to this textual search string, which then forms the basis for defining research progression.
On the BioStrand platform, for instance, the research experience is centred around the free-search bar that enables researchers to choose between sequence or textual searches. Our unique technological framework ensures that even a plain text search instantaneously returns all biological sequence data, organised by DNA, RNA and Amino Acids, related to textual context.
Researchers can then leverage the platform’s built-in tools and features to drill down and filter through the results, explore relationships/associations to isolate pertinent sequences, and then define the research pathway with the most potential for delivering novel breakthroughs.
This essentially means that researchers can start with a general search term (e.g., “coronavirus), drill down to a specific element (e.g., glycoproteins), isolate a sequence of interest (e.g., a regional variation), and then decide to focus on the variant-related epitopes that are apposite to their investigation.
The primary advantage of this text-based approach is that it eliminates the need for predefined data formats and preliminary parameters and filters. With BioStrand’s extensive and continuously updated knowledge base and advanced search engine capabilities, kickstarting a research project could be as simple as typing in a topic of interest and hitting enter.
Taking text beyond sequence search
The evolution of next-generation sequencing technologies, in terms of cost, speed, accuracy, and throughput, has exponentially increased the generation of high-dimensional genomic big data.
Even as we contend with the computation and scalability challenges of this data deluge, an entire corpus of potential knowledge still exists outside the boundaries of conventional bioinformatics systems.
Take biomedical literature, for example, a vital medium for disseminating and communicating specialised knowledge about experiments, investigations, and discoveries to the global research community. And the volume of biomedical literature is exploding. According to one estimate, over 3,000 articles are published in biomedical journals every day.
A recent analysis of COVID-19–related publications in CORD-19, an open-access dataset of scholarly articles on coronaviruses, found an average of 990 articles being published every week.
Today, it is possible to use public search engines, such as PubMed and Google Scholar, to conduct keyword-based literature retrieval of relevant biomedical research articles. However, extracting knowledge from the retrieved literature is still very much a manual process. This huge gap between knowledge generation and extraction is what can be addressed effectively by advanced biomedical literature mining (BLM) techniques.
Biomedical text mining and natural language processing (BioNLP) is a specialised domain that deals with processing textual data not just from research articles and scientific journals but also from medical records and other biomedical documents.
The capability to extract knowledge from vast volumes of textual data opens up a range of new possibilities including the potential to identify links between concepts detailed in disparate literature sources in order to generate novel hypotheses.
For instance, using high-throughput metabolomics analysis to observe changes in the metabolites related to a specific condition can provide valuable insights into the cause, progression, and treatment of a disease. However, in the case of a condition like cardiac arrest, gaining insights into the underlying biochemical processes can be extremely complex and time-consuming.
But research has shown that combining metabolomics with LBD techniques significantly enriched the hypothesis generation process, enabling researchers to infer novel metabolic pathways associated with the disease and to identify novel druggable targets.
So, there is clearly huge potential for textual data to augment conventional omics research processes. But in order to maximise the value derived from text data, BioNLP must become an intrinsic part of the analytical framework of modern bioinformatics and multi-omics research. The emphasis has to be on seamlessly merging literature-based discoveries and sequence-level data and metadata to create a single source of truth for truly integrated multi-omics analysis.
A truly integrated text + sequence omics analysis framework will require a biomedical domain-specific NLP solution that can organically link all data, including sequence, text, and medical/health records.
Making text work for omics research
At BioStrand, our focus is always on making omics research more effective, efficient, and productive. And we believe that textual data has a meaningful role to play in facilitating that experience.
Our unique technology for text-based sequence retrieval gives researchers the freedom to start with a contextual query, rather than a formatted sequence, and then chart their way forward based on the subjective merit of the results.
Soon, they will also be able to harness the power of our advanced biomedical literature mining technology to enrich those results with relevant associations to scholarly articles and journals.
Watch this space.
Subscribe to our Blog and get new articles right after publication into your inbox.