The evolution of bioinformatics
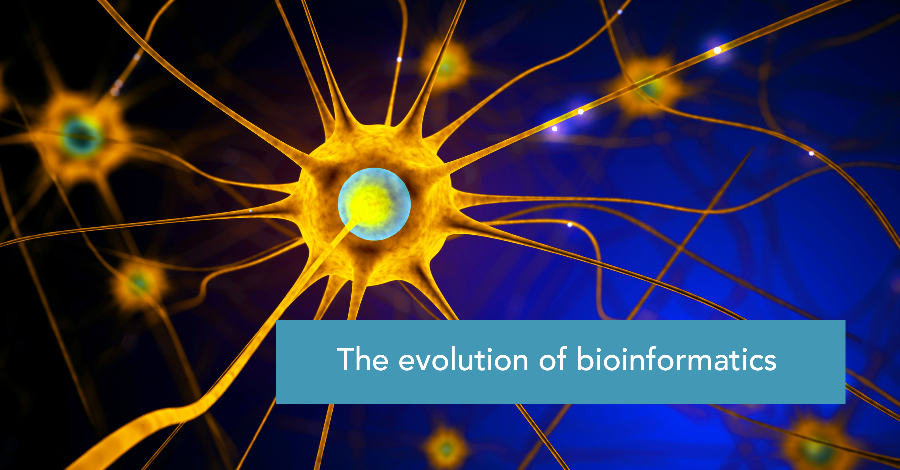
Conventional vaccine development, still based predominantly on systems developed in the last century, is a complex process that takes between 10-15 years on average. Until the COVID-19 pandemic, when two mRNA vaccines went from development to deployment in less than a year, the record for the fastest development of a new vaccine, in just four years, had gone unchallenged for over half a century.
This revolutionary boost to the vaccine development cycle stemmed from two uniquely 21st century developments: first, the access to cost-effective next-generation sequencing technologies with significantly enhanced speed, coverage and accuracy that enabled the rapid sequencing of the SARS-CoV-2 virus.
And second, the availability of innovative state-of-the-art bioinformatics technologies to convert raw data into actionable insights, without which NGS would have just resulted in huge stockpiles of dormant or dark data. In the case of COVID-19, cutting edge bioinformatics approaches played a critical role in enabling researchers to quickly hone in on the spike protein gene as the vaccine candidate.
NGS technologies and advanced bioinformatics solutions have been pivotal to mitigate the global impact of COVID-19, providing the tools required for detection, tracking, containment and treatment, the identification of biomarkers, the discovery of potential drug targets, drug repurposing, and exploring other therapeutic opportunities.
However, the combination of gene engineering and information technologies is already creating the foundation for the fourth generation of sequencing technologies for faster and more cost-effective whole-genome sequencing and disease diagnosis. As a result, continuous innovation has become an evolutionary imperative for modern bioinformatics as it has to keep up with the developmental pace of NGS technologies and accelerate the transformation of an exponentially increasing trove of data into knowledge.
However, the raw volume and velocity of data sequences is just one facet of big data genomics. Today, bioinformatics solutions have to cope with a variety of complex data, in heterogeneous formats, from diverse data sources, from different sequencing methods connected to different -omes, and relating to different characteristics of genomes.
More importantly, the critical focus of next-generation bioinformatics technologies has to be on catalysing new pathways and dimensions in biological research that can drive transformative change in precision medicine and public health.
In the following section, we look at the current evolutionary trajectory of bioinformatics in the context of three key omics analysis milestones.
Three key milestones in the evolution of bioinformatics
The steady evolution of bioinformatics over the past two decades into a cross-disciplinary and advanced computational practice has enabled several noteworthy milestones in omics analysis. The following, however, are significant as they best showcase the growth and expansion of omics research across multiple biological layers and dimensions, all made possible by a new breed of bioinformatics solutions.
Searching and aligning sequences are in its essence a problem of matching letters on a grid and assigning regions of high similarity versus regions of high variation. But nature has done a great deal to make this a challenging task.
Integrated multi-omics
For years, omics data has provided the requisite basis for the molecular characterisation of various diseases. However, genomic studies of diseases, like cancer for example, invariably include data from heterogeneous data sources and understanding cross-data associations and interactions can reveal deep molecular insights into complex biological processes that may simply not be possible with single-source analysis.
Combining data across metabolomics, genomics, transcriptomics, and proteomics can reveal hidden associations and interactions between omics variables, elucidate the complex relationships between molecular layers and enable a holistic, pathway-oriented view of biology.
An integrated and unified approach to multiple omics analysis has a range of novel applications in the prediction, detection, and prevention of various diseases, in drug discovery, and in designing personalised treatments.
And, thanks to the development of next-generation bioinformatics platforms, it is now possible to integrate not just omics data but all types of relevant medical, clinical, and biological data, both structured and unstructured, under a unified analytical framework for a truly integrated approach to multi-omics analysis.
Single-cell multi-omics
Where multi-omics approaches focus on the interactions between omics layers to clarify complex biological processes, single-cell multi-omics enable the simultaneous and comprehensive analysis of the unique genotypic and phenotypic characteristics of single cells as well as the regulatory mechanisms that are evident only at single-cell resolution.
Earlier approaches to single-cell analysis involved the synthesis of data from individual cells and then computationally linking different modalities across cells. But with next-generation multi-omics technologies, it is now possible to directly look at each cell in multiple ways and perform multiple analyses at the single-cell level.
Today, advanced single-cell multi-omics technologies can measure a wide range of modalities, including genomics, transcriptomics, epigenomics, and proteomics, to provide ground-breaking insights into cellular phenotypes and biological processes.
Best-in-class solutions provide the framework required to seamlessly integrate huge volumes of granular data across multiple experiments, measurements, cell types, and organisms, and facilitate the integrative and comprehensive analysis of single-cell data.
Spatial transcriptomics
Single-cell RNA sequencing enabled a more fine-grained assessment of each cell’s transcriptome. However, single-cell sequencing techniques are limited to tissue-dissociated cells that have lost all spatial information.
Delineating the positional context of cell types within a tissue is important for several reasons, including the need to understand the chain of information between cells in a tissue, to correlate cell groups and cellular functions, and to identify cell distribution differences between normal and diseased cells.
Spatial single-cell transcriptomics, or spatialomics, considered to be the next wave after single-cell analysis, combines imaging and single-cell sequencing to map the position of particular transcripts on a tissue, thereby revealing where particular genes are expressed and indicating the functional context of individual cells.
Even though many bioinformatics capabilities for the analysis of single-cell RNA-seq data are shared with spatially resolved data, analysis pipelines diverge at the level of the quantification matrix, requiring specialised tools to extract knowledge from spatial data.
However, there are advanced analytics platforms that use a unique single data framework to ingest all types of data, including spatial coordinates, for integrated analysis.
Quo Vadis, bioinformatics?
Bioinformatics will continue to evolve alongside, if not ahead of, emerging needs and opportunities in biological research. But if there is one key takeaway from the examples cited here, it is that a reductionist approach – one that is limited to a single omics modality or discipline or even dimension – yields limited and often suboptimal results.
If bioinformatics is to continue driving cutting edge biological research to tackle some of the most complex questions of our times, then the focus needs to be on developing a more holistic, systems bioinformatics approach to analysis.
Bioinformatics systems biology analysis is not an entirely novel concept, though its application is not particularly commonplace. But systems bioinformatics applies a well-defined systems approach framework to the entire spectrum of omics data with the emphasis on defining the level of resolution and the boundary of the system of interest in order to study the system as a whole, rather than as a sum of its components.
The focus is on combining the bottom-up approach of systems biology with the data-driven top-down approach of classical bioinformatics to integrate different levels of information.
The advent of multi-omics has, quite paradoxically, only served to accentuate the inherently siloed nature of omics approaches. Even though the pace of bioinformatics innovations has picked up over the past couple of decades, the broader practice itself is still mired in a fragmented multiplicity of domain, project, or data specific solutions and pipelines.
There is still a dearth of integrated end-to-end solutions with the capabilities to integrate multi-modal datasets, scale effortlessly from the study of specific molecular mechanisms to system-wide analysis of biological systems, and empower collaboration across disciplines research communities.
Integration at scale and across disciplines, datasets, sources, and computational methodologies is now the grand challenge for bioinformatics and represents the first step towards a future of systems bioinformatics.
Subscribe to our Blog and get new articles right after publication into your inbox.