Key challenges of single-cell data analysis
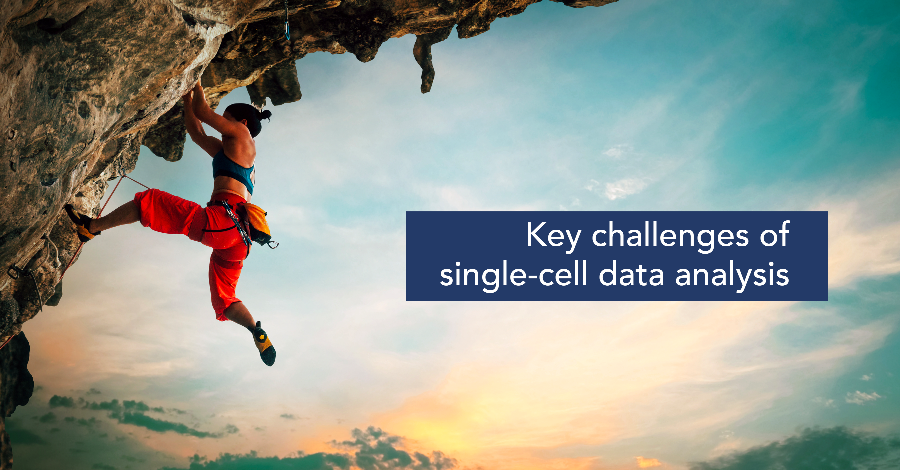
Tissue-level bulk transcriptomic studies can successfully cut through the clinical and genetic heterogeneity of autism spectrum disorder (ASD) and highlight common genes and pathways that are affected in the neocortex of autism patients. However, understanding the cell-level pathology of ASD and assessing its impact on specific cell types within the brain requires single-cell techniques.
With single-cell technologies, it is now possible to identify specific sets of genes that correlate to the clinical severity of ASD and even isolate ASD-specific gene expression changes that represent high-priority therapeutic targets for ASD.
The cellular diversity of the brain and the inadequacy of conventional methods to resolve the cellular heterogeneity of neurodegenerative diseases have made single-cell genomics an ideal match for neuroscience. However, these techniques have also expanded significantly into other areas in the biological sciences including biochemical, diagnostic, and biomedical research.
The potential of single-cell analysis
Single-cell analysis is characterized by two cardinal features: 1). Enabling the isolation, barcoding and sequencing of different types of molecules from individual cells, and 2). Facilitating the integrative analysis of molecules in order to define cell types in terms of their functions in pathophysiological processes.
The first feature, i.e., the ability to sequence individual cells at a molecular level, represents a host of transformative benefits for the biological sciences.
Granular biological information: Where tissue-level techniques tend to homogenise cell type and functionality, single-cell analysis helps elucidate the inherent heterogeneity of constituent cell types and functions and its subsequent role in the functioning of complex biological systems. As a result, it delivers more granular biological information with a whole range of potential applications in functional genomics, neurology, immunology, oncology, and stem cell biology.
Analysis of rare/complex cell types: Single-cell resolution data can be extremely useful in studying cells in tissues with complex morphology, such as the brain and endometrium. In terms of the analysis of rare cell types, conventional methods are restricted to large cell population data and not to trace quantity samples. Single-cell techniques, on the other hand, can optimally analyse rare cell types based on an abundant or limited volume of data.
Synergize with Single + Bulk: Many research studies have already established that combining single-cell and bulk analysis techniques can help smooth over each method’s rough edges or even enable a more omprehensive elucidation of molecular mechanisms. For instance, blending bulk sequencing and single-cell methods for detecting allelic expression imbalance addresses the limitations of each individual approach to enable the successful characterisation of AEI.
Expand the frontiers of biological research: In recent years, the scope for single-cell sequencing has been steadily expanding beyond single-cell RNA sequencing to multiple omics layers and the analysis of genomic DNA, protein expression and epigenetic signatures. At the same time, the scope for single-cell is expanding beyond routine analyses, like cell type identification, alternative splicing detection, trajectory, and GRN inference, to the extraction of more valuable information, regarding cell-to-cell communications, RNA velocity, large-scale CNVs and chromatin accessibility, from single-cell data.
Single-cell analysis can potentially seed the next generation of clinical diagnostics and medical research. The field is already witnessing a lot of activity in terms of profiling a wide range of human cell types, including whole organs. In fact, several major organs have already been profiled. There are also multiple ‘atlas’ projects – such as Human Cell Atlas, JingleBells, and conquer – underway across the globe to provide uniformly processed single-cell genomics data for downstream bioinformatics research.
And this is where the conversation turns from the potential to the challenges of single-cell data analysis.
The challenges of single-cell data science
The primary challenge for downstream bioinformatics analysis is to seamlessly integrate huge volumes of granular data and then facilitate the integrative analysis of single-cell data.
This is where the ability of conventional tools and frameworks is called into question, either in terms of integrating single-cell genomics data in a meaningful way, scaling to handle explosive growth in data volume, or enabling access to easy-to-use analysis tools that do not require specialised expertise in complex bioinformatics techniques.
Above and beyond these conceptual data science concerns, there are several challenges that are new and unique to the emergent and rapidly evolving practice of single-cell data science (SCDS).
Here we focus on three of the key challenges of single-cell analysis.
Coping with scale and uncertainty
Cell atlases provide a standardised reference framework of cell types and states that is essential for bioinformatics analyses. However, these frameworks are still evolving, and this can create several flexibility and scalability challenges for downstream analytics tools and frameworks.
For instance, bioinformatics solutions must have the flexibility to operate across multiple levels of resolution and across transient cell states. Over time, these solutions must be able to scale to incorporate more cells, more features, and broader coverage of types and states. As reference atlases continue to expand across multiple data dimensions, analytical solutions and frameworks must be capable of scaling to integrate multi-modal data in real-time.
Another significant challenge in single-cell data is that the increase in resolution comes with a reduction in signal stability. This means that single-cell data is more uncertain and sequence analysis tools must be capable of adequately capturing and quantifying these uncertainties into statistically sound qualifiers for downstream processes.
Finding meaning in spatial information
Single-cell spatial transcriptomics or proteomics technologies retain spatial coordinates of cells or even transcripts within a tissue. Another key challenge centres on the best way to leverage spatial information to determine patterns, infer cell types/functions and classify cells. Though there are currently several methods for grouping cells by type or function, none of them involves spatial information.
The central challenge, therefore, is to find a framework that combines spatial information and transcript/gene expression for assigning cells to types, classes, or functional groups independent of research questions or investigated cell types.
Integrating data across multiple dimensions
A comprehensive analysis of single-cell data will require an extremely rigorous and yet completely flexible framework for integrating data across multiple experiments, measurements, cell types and organisms. For instance, analytics solutions must be capable of integrating datasets across samples in one experiment, datasets across experiments, multiple measurement types from the same cell, multiple measurement types from different cells, multiple measurements across different time points, and so on. Over time, the data maps documenting the links between different types and sources will only get more complex.
Bringing the BioStrand edge to single-cell data
Designed for scalability
The BioStrand SaaS platform’s container-based architecture is designed for autoscaling to over 200 petabytes of data with zero on-ramping issues.
However, at BioStrand, volume is just one metric of scalability. Our platform is also designed to scale and retrieve data from multiple reference databases, with the capacity to classify one billion reads in just 12 hours. As the datasets in reference cell atlases grow broader, deeper and richer, the platform will scale, without any loss in speed or accuracy, to automatically integrate multi-source data in near real-time.
Designed for heterogeneity
Using a unique single data framework based on the HYFT™ IP, the BioStrand platform integrates all types of data and metadata, including spatial coordinates, and makes them computable. Plus, it provides ready-made research access to pre-compiled, pre-computed, and pre-indexed multimodal multi-omics datasets from across 11 public databases. So, irrespective of whether it’s structured or unstructured data, if it’s biological data researchers can normalise and integrate it with a single click.
Designed for dimensionality
BioStrand’s unique HYFT™ framework tokenizes all biological data, across type, domain, function and organism, to a common omics language that facilitates the integrated analysis of multidimensional data. HYFTs™ simplify the normalisation and integration of data from multiple experiments, measurements, cell types and organisms and make complex single-cell analysis computationally feasible.
With unified access to all relevant data, researchers can then leverage the integrated analytical workflows, advanced annotation and analysis capabilities and the AI-powered features of the BioStrand platform to succeed at single-cell data science.
Subscribe to our Blog and get new articles right after publication into your inbox.