Why omics data analysis needs to be remade
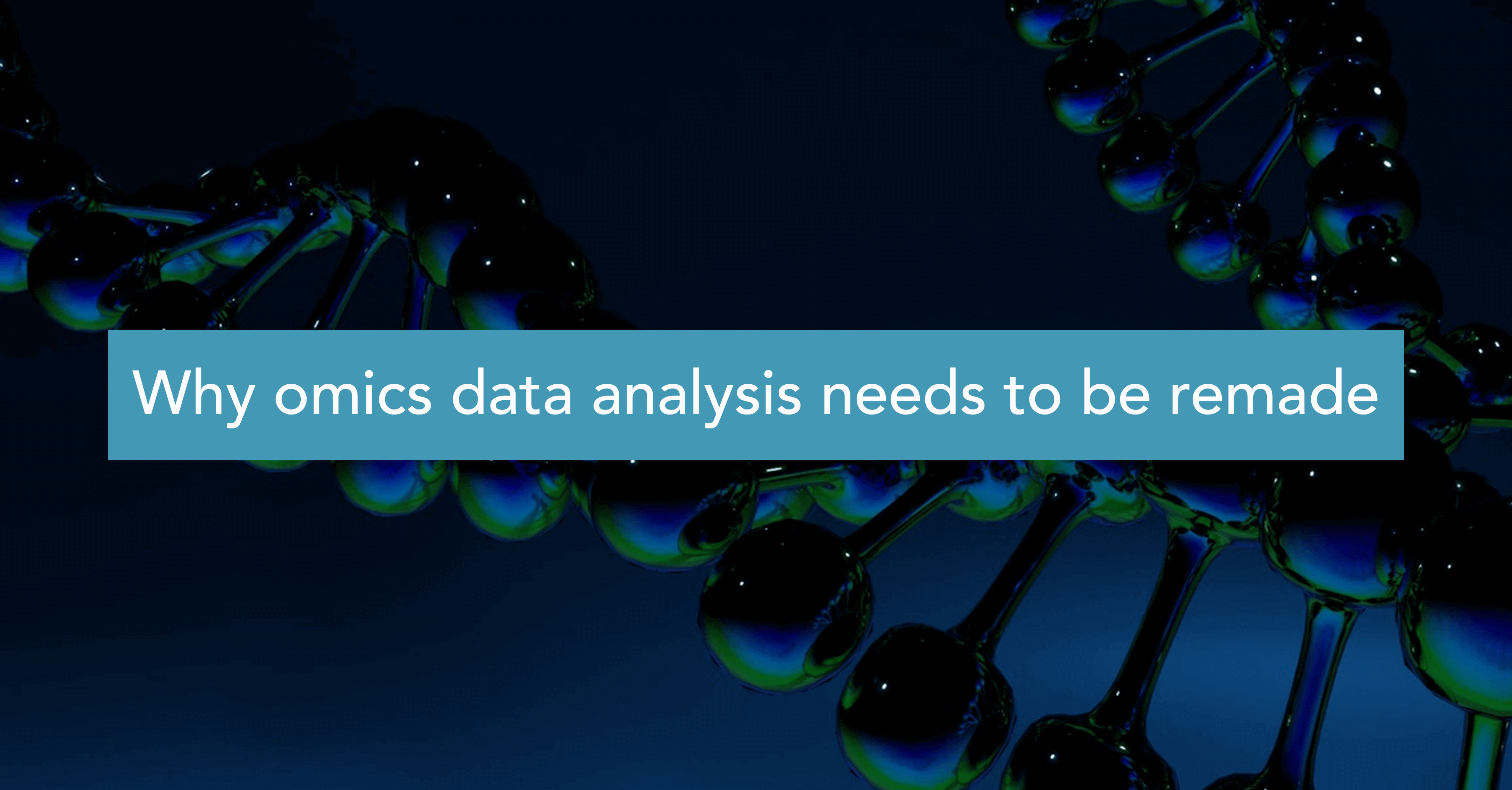
Today, more than ever, massive amounts of biological data are at our disposal.
Yet, our ability to organise, analyse and interpret data and to therefore extract therefrom biologically relevant information, is trailing behind.
Creating a faultless system, integrating fundamental changes necessary to advance the field of omics analysis will equip researchers with the tools and information leading to important scientific breakthroughs.
The ultimate goal of omics data analysis is to identify every gene in human profiles and other organisms, and to match each gene with the protein it encodes and reveal the structure and function of each protein.
Understanding these biological processes at the molecular level contributes to understanding metabolic pathways and mechanisms of disease.
However, with the current omics data analysis technologies, conceptual challenges and weaknesses have been exposed. Data analysis is complex and time-consuming and requires highly trained professionals.
There is a lot of fragmentation, such as many different data-formats, databases, and a multitude of analysis tools for very specific purposes or domains. As a consequence, current data analysis solutions are not able to keep pace with data generation.
So, our ability to organise, analyse and interpret data and to therefore extract biologically relevant information, is trailing behind. This hampers evolutions in different fields of science and the transfer of knowledge to clinical practice and other domains.
History of omics analysis
Over the last few decades, array systems like mass spectrometers and sequencers have been developed to read sequences of DNA, RNA and proteins. Already more than 15 years ago, the first complete sequencing of the human genome was achieved within the human genome project.
To fuel these evolutions, ample technical progress has been required, spanning from advances in sample preparation and sequencing methods, to data acquisition, data processing and the development of different analysis methodologies. New scientific fields emerged and developed, including genomics, transcriptomics, proteomics and bioinformatics.
Technologies like next generation sequencing revolutionised the process of sequencing DNA and RNA (primary analysis). Sequencing was suddenly possible at a massive scale and at relatively low cost. Yet, a similar technological breakthrough in the secondary and tertiary analysis steps, which would be necessary to interpret the data coming out of all these array systems at sufficient speed and accuracy, did not occur.
Data analysis methodologies to assemble data, to align data to a reference genome, to annotate, to detect variations, etc. have evolved over the last years, but the current state-of-the-art algorithms remain largely insufficient to process the vast amount of sequence information that is being generated today.
The fragmented landscape of omics data analysis technologies
Although the core questions in genetic research are related to disentangling the associations between DNA, RNA and protein, the current tools and methods of data analysis are not oriented towards integration of knowledge.
Today, data analysis is characterised by fragmentation. Whether you are interested in finding similarities or in detecting variations, all omics data analysis is organised in silo’s with different applications for each analytical step. Along the different steps in the analysis process, outputs are generated in different formats.
Although automated pipelines are available, the process of analysis remains time consuming and very complex. Only highly trained professionals are able to perform these analyses.
The integration of biological databases is also lacking. So, it is very difficult to query more than one database at a time. Currently, there is also no way to combine analysis in genomics, transcriptomics and proteomics which has proven to be a blocking factor. It is certainly not very helpful in maintaining oversight and easily detecting novel relationships.
Moreover, the current algorithms are far from flawless and result in an accumulation of errors during the analysis process. Furthermore, most algorithms are computationally very intensive which results in slow processing times.
True innovation requires disruption. Without disrupting the data analysis technologies, the lack of accuracy and accessibility will continue to bear ongoing issues. We need to develop algorithms that are able to process vast amounts of data in the blink of an eye. Yet, a high level of accuracy is indispensable when processing data related to life sciences, pharmaceuticals and treatments.
For example, determining the genetic variations in subgroups of patients who show a different response to a cancer treatment is very important in improvement of personalised medicine. Being able to deliver results fast and accurately is of great importance in this kind of research, as it accelerates the drug development and evaluation process. The same is true for agriculture and food technology, where accuracy is also a prerequisite.
What are the roadblocks for omics analysis and what are the possibilities
Proven roadblocks for omics data analysis include genomics, transcriptomics and proteomics evaluated with an individual and unconnected approach which results in monothematic knowledge as opposed to integrated knowledge.
New developments in omics data analysis technologies should be aimed at integration of knowledge and at increasing precision of analysis. This would bring a high level of accessibility, efficiency and accuracy to the field.
Further downstream advanced analysis methods such as machine learning or graph reasoning can only produce meaningful insights and predictions when the data that serve as input are of high quality.
There exists no classification or prediction algorithm that can compensate for the quality of input data. So, in order to make better models such as in relation to disease mechanisms, or for drug target development, we need algorithms for detection of similarities and variations in DNA, RNA and proteins that produce highly accurate results.
Only then, we will be able to deliver better insights and better predictions leading to real advancements in precision medicine and other fields of science.
Integration of data analysis between genomics, transcriptomics and proteomics would not only expand the search field but also bridge the gap between isolated silo’s. It would facilitate the discovery of novel relationships such as between species, in gene transcription processes and other kinds of knowledge necessary for progression in medicine, and other life sciences.
Dire need for change
Although omics data analysis technologies have contributed over the years to obtaining better insights in life sciences, currently the lack of major developments in this field has led to a major bottleneck. Data analysis is not able to keep pace with data generation.
The lack of integration in data analysis between genomics, transcriptomics and proteomics, the inaccuracy in data analysis and inability to process the vast amount of data that is generated, leads to a general deceleration in research and development. This means a slowdown in the translation of knowledge from bench to bedside, from lab to farmer, from lab to sustainable food production and more.
If we are able to simplify and streamline the procedure of data analysis and bring it in sync with the process of data collection, obstructions that currently exist in omics analysis will cease to exist. Creating a faultless system, integrating fundamental changes necessary to advance the field of omics analysis will equip researchers with the tools and information leading to important scientific breakthroughs.
Most of the current challenges of omics analysis – like fragmented and siloed data, a profusion of analytical tools and workflows, etc. – can be traced back to just one factor: the heterogeneity of omics data.
The BioStrand approach to multi-omics analysis addresses this issue with an integrated solution that can be applied to the universe of biological data. BioStrand’s proprietary HYFTs™ IP makes all biological data, including metadata, instantly computable across species, domain and regulation, structured and unstructured data types, and public and proprietary data sources. With the BioStrand Platform, it is even possible to add data from textual sources such as sequence descriptions, annotations, scientific/medical literature, healthcare data, electronic patient records, etc. This automated framework for the normalisation and integration of pan-omics data provides the foundation for our integrated model of biological research.
With BioStrand, all research-relevant data is pooled into a unified data repository and encoded into a unified, integrated, and intelligent analytical engine that enables efficient, accurate, scalable, and holistic genomics research. BioStrand’s flexible analytical framework and intuitive UI allows researchers to customise and optimise their research pathways for maximum productivity and accelerated knowledge extraction.
Whether you are a domain specialist with no computer science background or a skilled bioinformatician or data scientist, the BioStrand end-to-end, SaaS platform provides you with all the capabilities required to make your multi-omics research more efficient, effective, and accurate.
Subscribe to our Blog and get new articles right after publication into your inbox.