Data-Driven Biomedical Research
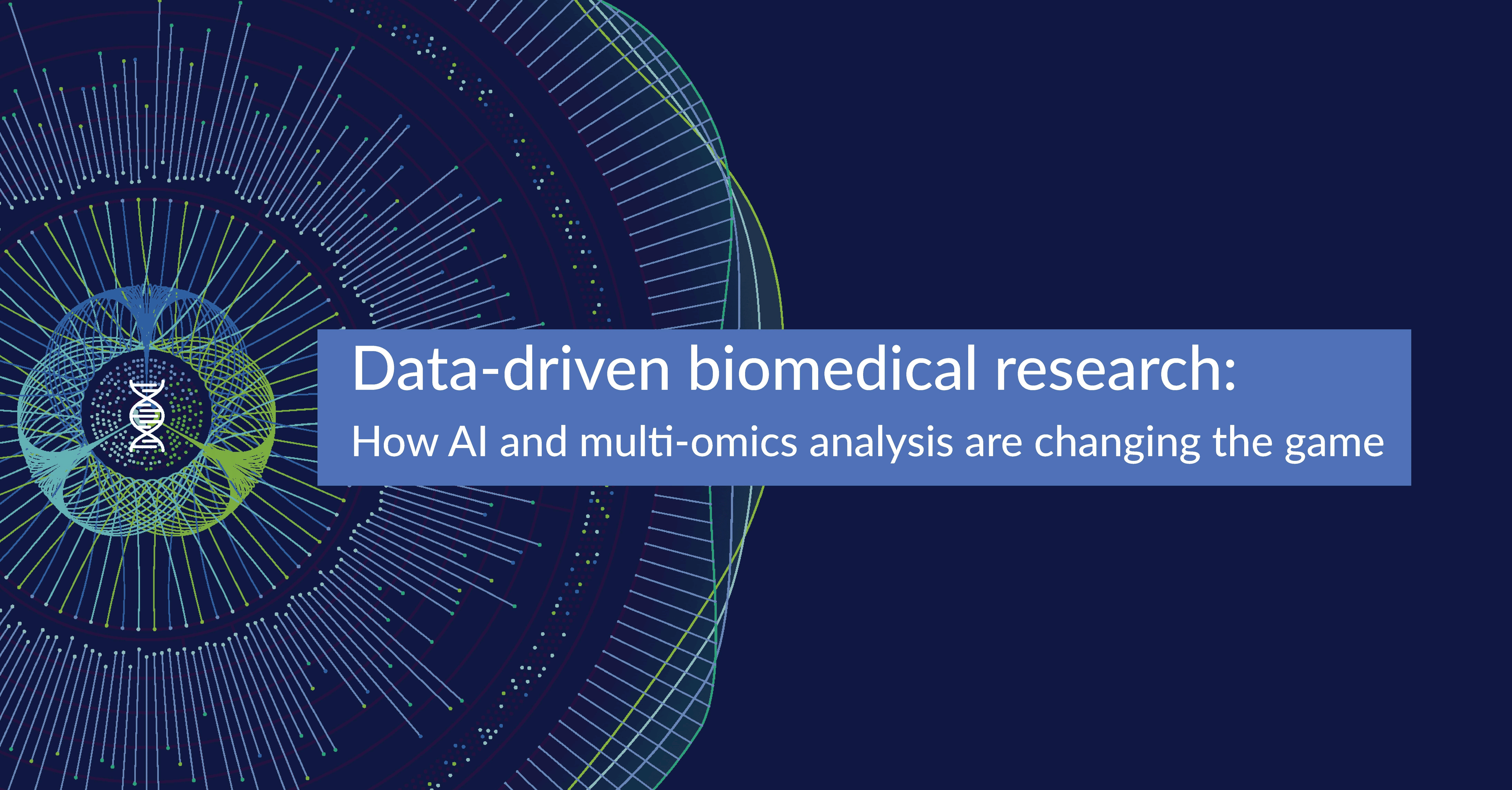
Data-driven biomedical research is the foundation of effective precision medicine (PM). Of course, over the long term, the success of the PM model will be determined as much by the productivity of data-driven research as it will be by our ability to translate research‐based theories and discoveries into clinical precision medicine.
The expanding universe of biomedical data
This paradigm shift from a symptom-specific to a patient-specific approach to biomedical research and medicine is powered by exponential volumes of multimodal data from a wide range of domains including molecular data from primary/secondary biomedical research, clinical data from laboratory studies and EHRs, and non-clinical data pertaining to environmental, lifestyle and socio-economic factors.
Since the advent of the first omics discipline of genomics, the scope of omics technologies has constantly been expanding across multiple biological layers. Advances in NGS and mass spectrometry technologies have significantly expanded our knowledge of the biomolecular milieu and ushered in a new era of multi-omics analysis.
However, the explosion of data from primary and secondary research only created new challenges in multi-omics data integration. It has been estimated that genome sequence data alone will be the biggest Big Data domain of all by 2025, the same year that healthcare data is expected to start doubling every 73 days.
All this meant that biomedical research needed new computational methods that were better at dealing with such huge volumes of data than traditional statistical approaches.
AI/ML in biomedical research
As omics data sets become more multi-layered and multidimensional, advanced computational methods powered by intelligent AI/ML technologies became the key to integrating and transforming complex multi-omics data sets into actionable knowledge.
These technologies soon expanded beyond the omics domain into new fields, such as radiogenomics, a complementary field in precision medicine. ML/DL models have helped automate the assessment of radiological images for diagnosis, staging, and tumor segmentation while improving accuracy and reducing the time required. Deep learning networks have also demonstrated high performance across low-level and high-level tasks in the rather complex field of histopathology image analysis. Similar algorithmic approaches are now being adopted across a range of image analysis applications covering CT scans, mammographies, MRI, etc.
AI-based technologies like NLP have helped unlock access to unstructured textual data, i.e. documents, journal articles, blogs, emails, electronic health records, social media posts, etc., and bring the knowledge embedded in these data sets within the purview of integrated biomedical research.
Notwithstanding the immense potential of AI/ML technologies in biomedical research and, therefore, precision medicine, there are still several technical and ethical challenges that need to be addressed. The PM model is built on the principle of the integrated analysis of vast volumes of data, including proprietary, personal, and often sensitive information. The efficiency and accuracy of data-intensive AI/ML models depend on troves of representative data. However, this immediately raises a host of compliance issues around security, privacy, ownership, and consent. Then there are the ethical questions of reliability, explainability, opacity, bias, trustworthiness, traceability, fairness, and moral responsibility.
All these technical and ethical factors will have a collective influence on the evolution of data-driven research into a comprehensive and practical precision medicine model. However, as mentioned at the outset, successful implementation of the PM model will also depend on the critical ability to translate research‐based theories and discoveries into clinical practice.
Translational research & precision medicine
According to a frequently cited statistic, there is a 17-year gap between the discovery of scientific evidence and its implementation in clinical practice. This gap between laboratory research and clinical practice is what the evolving scientific discipline of translational research seeks to address.
Translational research enables the transformation of theoretical and experimental knowledge into innovations at the point of care while also ensuring that there is a reverse flow of clinical information and insights to biomedical research.
A discovery-to-practice precision medicine pipeline will require the integration of humongous volumes of biomedical data from high-throughput multi-omics technologies, multi-modal imaging and clinical data, secondary research, EHRs, scientific publications, real-world data and non-clinical digital devices and health apps, amongst others. The real-time integration and analysis of all this data will require the deployment of advanced AI tools and technologies in the clinical setting.
However, the deployment of AI in clinical care, according to a 2022 report from the Council of Europe’s Steering Committee for Human Rights in the fields of Biomedicine and Health (CDBIO), remains nascent. Some of the report’s key observations include a huge lag between the scale of research activity vis-a-vis demonstrating clinical efficacy, inadequate generalization of performance from trials to clinical practice, and inability to translate research, development, and testing into broader clinical deployment.
A multiparametric practice like personalized medicine requires advanced AI/ML capabilities that span the spectrum of translational medicine from research to testing, clinical practice, and patient management. Despite increasing ambitions and investments in ML for Health (ML4H) in routine clinical care, the deployment of these models is currently limited to isolated workflows, like radiology, for example. This gap between research AI and clinical AI is now being addressed by the new field of translational ML or translational AI. The futuristic vision for this approach is to combine discrete AI agents from across the spectrum of translational medicine into one collaborative translational AI that will enable real-time lab-to-clinic analysis.
Translational omics and precision medicine
A more immediate approach to expanding the use of AI/ML in clinical practice could be to use omics as an AI strategy. AI-driven translational omics, the clinical utilization of molecular data derived from multiple biological domains, can create the framework for precision medicine initiatives. Combining state-of-the-art multi-omics technologies with AI-based integration and analysis strategies has had a significant impact on cancer precision medicine, in terms of early screening, diagnosis, response assessment, and prognosis prediction. AI technologies have the proven potential to streamline the integration of multi-modal omics with imaging, phenotypic, EHR, and patient-specific data to generate more precise insights into disease biology and enhance routine clinical decision-making. Therefore, an AI-enabled multi-omics strategy may be the first evolutionary step required toward realizing the vision of an end-to-end research-lab-to-clinical-care model of precision medicine.
Subscribe to our Blog and get new articles right after publication into your inbox.