Integrating knowledge graphs and large language models for next-generation drug discovery
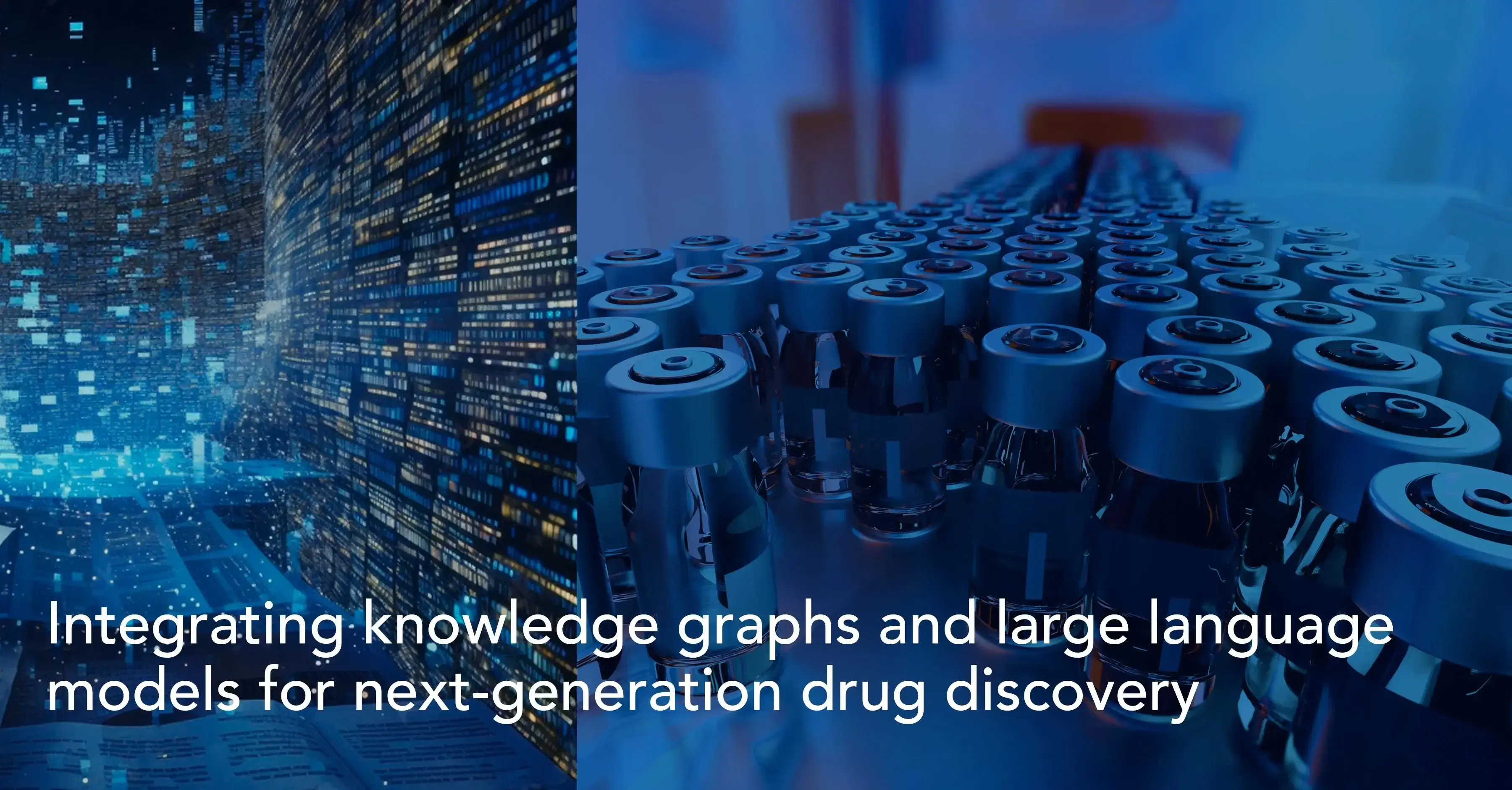
Audio version
Across several previous blogs, we have explored the importance of Knowledge Graphs, Large Language Models (LLMs), and semantic analysis in biomedical research. Today, we focus on integrating these distinct concepts into a unified model that can help advance drug discovery and development.
But before we get to that, here’s a quick synopsis of the knowledge graph, LLM & semantic analysis narrative so far.
LLMs, knowledge graphs & semantics in biomedical research
It has been established that biomedical LLMs — domain-specific models pre-trained exclusively on domain-specific vocabulary — outperform conventional tools in many biological data-based tasks. It is therefore considered inevitable that these models will quickly expand across the broader biomedical domain.
However, there are still several challenges, such as hallucinations and interpretability for instance, that have to be addressed before biomedical LLMs can be taken mainstream. A key biomedical domain-specific challenge is LLMs’ lack of semantic intelligence.
LLMs have, debatably, been described as ‘stochastic parrots’ that comprehend none of the language, relying instead on ‘learning’ meaning based on the large-scale extraction of statistical correlations. This has led to the question of whether modern LLMs really possess any inductive, deductive, or abductive reasoning abilities.
Statistically extrapolated meaning may well be adequate for general language LLM applications. However, the unique complexities and nuances of the biochemical, biomedical, and biological vocabulary, require a more semantic approach to convert words/sentences into meaning, and ultimately knowledge.
Biomedical Knowledge Graphs address this key capability gap in LLMs by going beyond statistical correlations to bring the power of context to biomedical language models. Knowledge graphs help capture the inherent graph structure of biomedical data, such as drug-disease interactions and protein-protein interactions, and model complex relationships between disparate data elements into one unified structure that is both human-readable and computationally accessible.
Knowledge graphs accomplish this by emphasizing the definitions of, and the semantic relationships between, different entities. They use domain-specific ontologies that formally define various concepts and relations to enrich and interlink data based on context. A combination, therefore, of semantic knowledge graphs and biomedical LLMs will be most effective for life sciences applications.
Semantic knowledge graphs and LLMs in drug discovery
There are three general frameworks for unifying the power of LLMs and knowledge graphs.
The first, knowledge graph-enhanced LLMs, focuses on using the explicit, structured knowledge of knowledge graphs to enhance the knowledge of LLMs at different stages including pre-training, inference, and interpretability. This approach offers three distinct advantages: it improves the knowledge expression of LLMs, provides LLMs with continuous access to the most up-to-date knowledge, and affords more transparency into the reasoning process of black-box language models. Structured data from knowledge graphs, related to genes, proteins, diseases, pathways, chemical compounds, etc., combined with the unstructured data, from scientific literature, clinical trial reports, and patents. etc, can help augment drug discovery by providing a more holistic domain view.
The second, LLM-augmented knowledge graphs, leverages the power of language models to streamline graph construction, enhance knowledge graph tasks such as graph-to-text generation and question answering, and augment the reasoning capabilities and performance of knowledge graph applications. LLM-augmented knowledge graphs combine the natural language capabilities of LLMs with the rich semantic relationships represented in knowledge graphs to empower pharmaceutical researchers with faster and more precise answers to complex questions and to extract insights based on patterns and correlations. LLMs can also enhance the utility of knowledge graphs in drug discovery by constantly extracting and enriching pharmaceutical knowledge graphs.
The third approach is towards creating a synergistic biomedical LLM plus biomedical knowledge graph (BKG) model that enables bidirectional data- and knowledge-based reasoning. Currently, the process of combining generative and reasoning capabilities into one symbiotic model is focused on specific tasks. However, this is poised to expand to diverse downstream applications in the near future.
Even as research continues to focus on the symbiotic possibilities of a unified knowledge graph-LLM framework, these concepts are already having a transformative impact on several drug discovery and development processes.
Take target identification, for instance, a critical step in drug discovery with consequential implications for downstream development processes. AI-powered language models have been shown to outperform state-of-the-art approaches in key tasks such as biomedical named entity recognition (BioNER) and biomedical relation extraction. Transformer-based LLMs are being used in chemoinformatics to advance drug–target relationship prediction and to effectively generate novel, valid, and unique molecules. LLMs are also evolving beyond basic text-to-text frameworks to multi-modal large language models (MLLMs) that bring the combined power of image plus text adaptive learning to target identification and validation. Meanwhile, the semantic capabilities of knowledge graphs enhance the efficiencies of target identification by enabling the harmonization and enrichment of heterogeneous data into one connected framework for more holistic exploration and analysis.
AI-enabled LLMs are increasingly being used across the drug discovery and development pipeline to predict drug-target interactions (DTIs) and drug-drug interactions, molecular properties, such as pharmacodynamics, pharmacokinetics, and toxicity, and even likely drug withdrawals from the market due to safety concerns. In the drug discovery domain, biomedical knowledge graphs are being across a range of tasks including polypharmacy prediction, DTI prediction, adverse drug reaction (ADR) prediction, gene-disease prioritization, and drug repurposing.
The next significant point of inflection will be the integration of these powerful technologies into one synergized model to drive a stepped increase in performance and efficiency.
Optimizing LLMs for biomedical research
There are three key challenges — knowledge cut-off, hallucinations, and interpretability — that must be addressed before LLMs can be reliably integrated into biomedical research. There are currently two complementary approaches to mitigate these challenges and optimize biomedical LLM performance.
The first approach is to leverage the structured, factual, domain-specific knowledge contained in biomedical knowledge graphs to enhance the factual accuracy, consistency, and transparency of LLMs. Using graph-based query languages, the pre-structured data embedded in knowledge graph frameworks can be directly queried and integrated into LLMs.
Another key capability for biomedical LLMs is to retrieve information from external sources, on a per-query basis, in order to generate the most up-to-date and contextually relevant responses. There are two broad reasons why this is a critical capability in biomedical research: first, it ensures that LLMs' internal knowledge is supplemented by access to the most current and reliable information from domain-specific, high-quality, and updateable knowledge sources. And two, access to the data sources means that responses can be checked for accuracy and provenance. The Retrieval Augmented Generation (RAG) approach combines the power of LLMs with external knowledge retrieval mechanisms to enhance the reasoning, accuracy, and knowledge recall of biomedical LLMs.
Combining the knowledge graph- and RAG-based approaches will lead to significant improvements in LLM performance in terms of factual accuracy, context-awareness, and continuous knowledge enrichment.
LENSai: The next-generation RAG-KG-LLM platform
At BioStrand, we have successfully actualized a next-generation unified knowledge graph-large language model framework for holistic life sciences research. At the core of our LENSai platform is a comprehensive and continuously expanding knowledge graph that maps 25 billion relationships across 660 million data objects, linking sequence, structure, function, and literature information from the entire biosphere. Our first-in-class technology provides a holistic understanding of the relationships between genes, proteins, and biological pathways thereby opening up powerful new opportunities for drug discovery and development. The platform leverages the latest advances in ontology-driven NLP and AI-driven LLMs to connect and correlate syntax (multi-modal sequential and structural data ) and semantics (functions). Our unified approach to biomedical knowledge graphs, retrieval-augmented generation models, and large language models combines the reasoning capabilities of LLMs, the semantic proficiency of knowledge graphs, and the versatile information retrieval capabilities of RAG to streamline the integration, exploration, and analysis of all biomedical data.
Subscribe to our Blog and get new articles right after publication into your inbox.