Knowledge Graphs & The Power Of Context
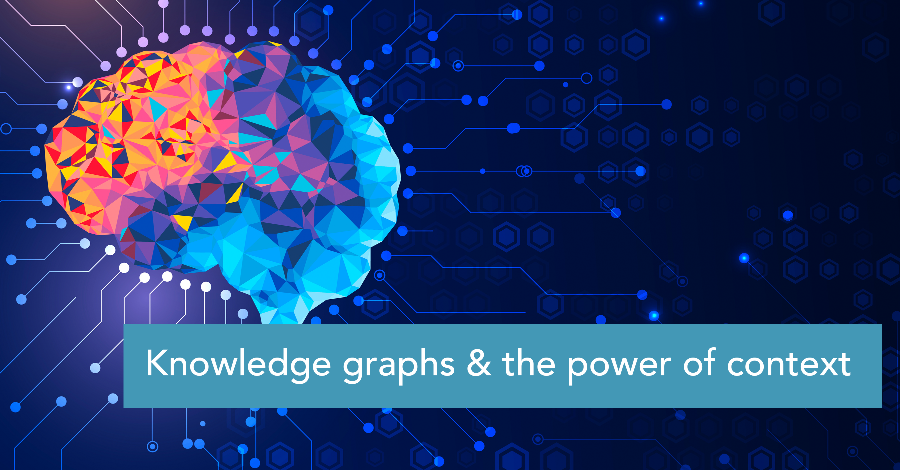
Data overload is becoming a real challenge for businesses of all stripes even as a majority continue gathering data faster than they can analyse and harness its business value.
And it’s not just about volume. Much of modern big data, as much as 93%, comes in the form of unstructured data and most if not all of which ends up as dark data i.e. collected but not analysed.
Unlocking knowledge at scale from troves of unstructured organisational data is rapidly becoming one of the most pressing needs for businesses today.
Concurrent themes in this regard include the importance of connected data, the value of applying knowledge in context and the benefits of using AI to contextualize data and create knowledge.
And the need for connected, contextualised data and continuing developments in AI has resulted in increasing interest in knowledge graphs as a means to generate context-based insights. In fact, Gartner believes that graph technologies are the foundation of modern data and analytics, noting that most client inquiries on the topic of AI typically involve a discussion on graph technology.
A brief history of knowledge graphs
In 1735s Königsberg, Swiss mathematician Leonhard Euler used a concept of nodes/objects and links/relationships to prove that there was no route across the city’s four districts that would involve crossing each of its interconnecting seven bridges exactly once, thereby laying the foundations for graph theory.
Cut to more modern times and 1956 witnessed the development of a semantic network, a well-known ancestor of knowledge graphs, for machine translation of natural languages. Fast forward to the early aughts, and Sir Timothy John Berners-Lee proposed a Semantic Web that would use structured and standardized metadata about webpages and their interlinks to make the knowledge stored in these relationships machine-readable.
Unfortunately, the concept did not exactly scale but search and social companies were quick to latch on to the value of extremely large graphs and the potential in extracting knowledge from them. Google is often credited with rebranding the semantic web and popularising knowledge graphs with the introduction of the Google Knowledge Graph in 2012.
Most of the first big knowledge graphs, from companies such as Google, IBM, Amazon, Samsung, eBay, Bloomberg, NY Times, compiled non-proprietary information into a single graph that served a wide range of interests. Enterprise knowledge graphs emerged as the second wave and used ontologies to elucidate various conceptual models (schemas, taxonomies, vocabularies, etc.) used across different enterprise systems.
Back in 2019, Gartner predicted that an annualised 100% growth in the application of graph processing and graph databases would help accelerate data preparation and enable more complex and adaptive data science. Today, graphs are considered to be one of the fastest-growing database niches, having surpassed the growth rate of standard classical relational databases, and graph DB + AI may well be the future of data management.
Defining knowledge graphs
A knowledge graph is quite simply any graph of data that accumulates and conveys knowledge of the real world. Data graphs can conform to different graph-based data models, such as a directed edge-labelled graph, a heterogeneous graph, a property graph, etc.
For instance, a directed labelled knowledge graph consists of nodes representing entities of interest, edges that connect nodes and reference potential relationships between various entities, and labels that capture the nature of the relationship.
So, knowledge graphs use a graph-based data model to integrate, manage and extract knowledge from diverse sources of data at scale. Knowledge graph databases enable AI systems to deal with huge volumes of complex data by storing information as a network of data points correlated by the nature of their relationships.
Key characteristics of knowledge graphs
By connecting multiple data points around relevant and contextually related attributes, graph technologies enable the creation of rich knowledge databases that enhance augmented analytics. Some of the most defining characteristics of this approach include:
- Knowledge graphs work across structured and unstructured datasets and represent the most credible means of aggregating all enterprise data regardless of structure variation, type, or format.
- Compared to knowledge bases with flat structures and static content, knowledge graphs integrate adjacent information on how different data points are correlated to enable a human brain-like approach to derive new knowledge.
- Knowledge graphs are dynamic and can be programmed to automatically identify attribute-based associations across new incoming data.
- The ability to create connected clusters of data based on levels of influence, frequency of interaction and probability opens up the possibility of developing and training highly complex models.
- Knowledge graphs simplify the process of integrating and analysing complicated data by establishing a semantic layer of business definitions. The use of intelligent metadata enables users to even find insights that otherwise might have been beyond the scope of analytics.
Applications of knowledge graphs
Today, knowledge graphs are everywhere. Every consumer-facing digital brand, such as Google, Amazon, Facebook, Spotify, etc., has invested significantly in building knowledge and the concept of graphs has evolved to underpin everything from critical infrastructure to supply chains and policing. Here’s a quick look at how this technology can transform certain key sectors and functions.
Healthcare
In the healthcare sector, it is especially critical that classification models are reliable and accurate. But this continues to be a challenge given the volume, quality and complexity of data within the sector. Despite the application of advanced classification methodologies, including deep learning, the outcomes do not demonstrate adequate superiority over previous techniques.
Much of this boils down to the fact that conventional techniques disregard correlations between data instances. However, it has been demonstrated that knowledge graph algorithms, with their inherent focus on correlations, could significantly advance capabilities for the discovery of knowledge and insights from connected data.
Finance
Knowledge graphs, and their ability to uncover new dimensions of data-driven knowledge, are expected to be adopted by as much as 80% of financial services firms in the near future. In fact, a 2020 report from business and technology management consultancy Capco provided a veritable laundry list of knowledge graph applications across the financial services value chain.
For instance, graphs can be used across compliance, KYC and fraud detection to build a ‘deep client insight’ capability that can transform compliance from a cost to a revenue-driving function. The adoption of graph data models could also drive product innovations given the inflexibility of current tabular data structures to reflect real-world needs.
Pharma
Machine learning approaches that use knowledge graphs have the potential to transform a range of drug discovery and development tasks, including drug repurposing, drug toxicity prediction and target gene-disease prioritisation. In the context of knowledge graph-based drug discovery, In a drug discovery graph, genes, diseases, drugs etc. are represented as entities with the edges indicating relationships/interactions.
As a result, an edge between a disease and drug entity could indicate a successful clinical trial. Similarly, an edge between two drug entities could reference either a potentially harmful interaction or compatibility. The pharma sector is also emerging as the ideal target for text-enhanced knowledge graph representation models that utilise textual information to augment knowledge representations.
Knowledge graphs and AI/ML
AI/ML technologies are playing an increasingly critical role in driving data-driven decision making in the digital enterprise. Knowledge graphs will play a significant role in sustaining and growing this trend by providing the context required for more intelligent decision-making.
There are two distinct reasons for knowledge graphs being at the epicentre of AI and machine learning. On the one hand, they are a manifestation of AI given their ability to derive a connected and contextualised understanding of diverse data points. On the other, they also represent a new approach to integrating all data, structured and unstructured, required to build the ML models that drive decision-making.
The combination, therefore, of knowledge graphs and AI technologies will be critical not only for integrating all enterprise data but also add the power of context to augment AI/ML approaches.