The importance of reproducibility in in-silico drug discovery
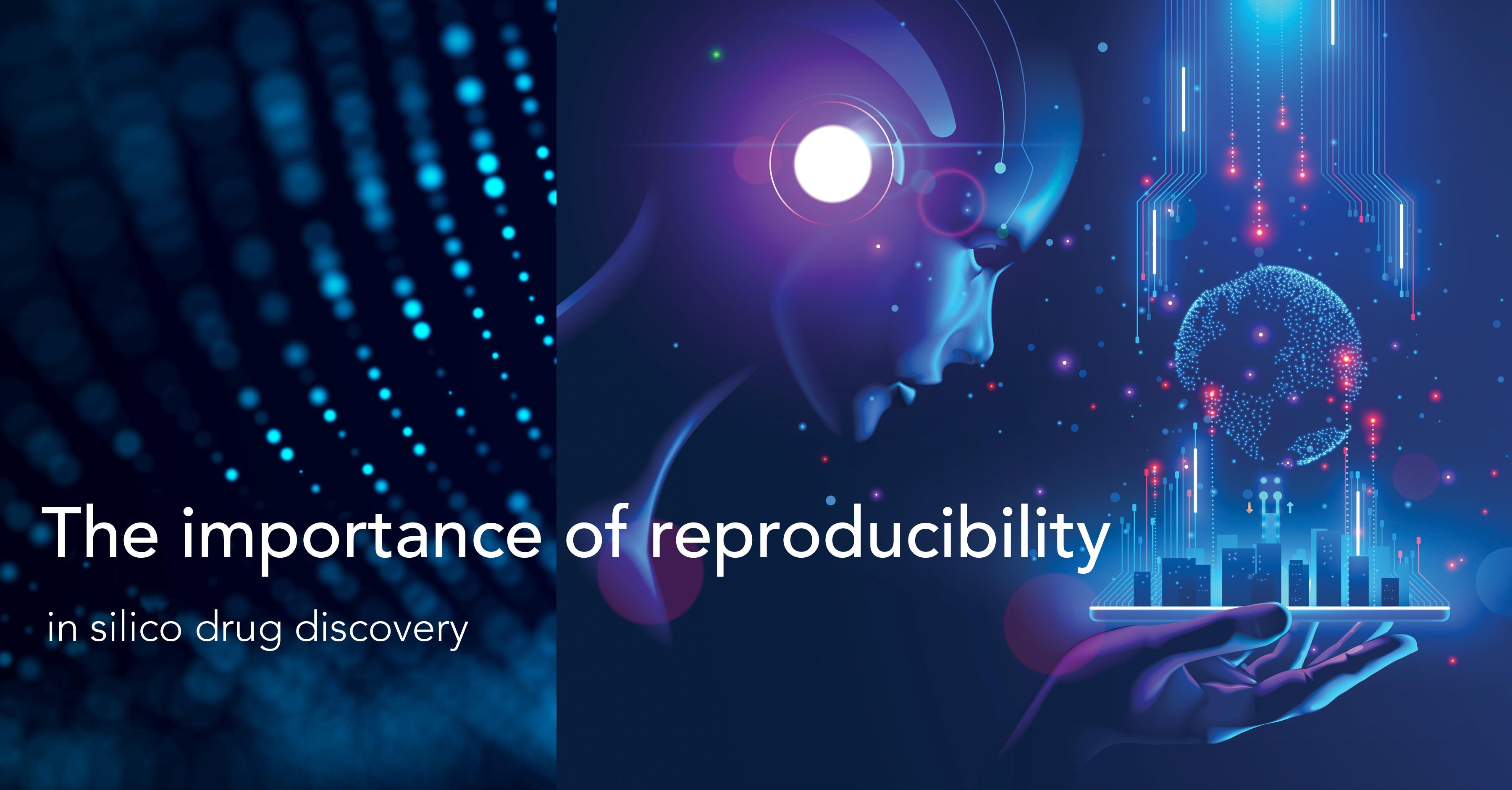
Reproducibility, getting the same results using the original data and analysis strategy, and replicability, is fundamental to valid, credible, and actionable scientific research. Without reproducibility, replicability, the ability to confirm research results within different data contexts, becomes moot.
A 2016 survey of researchers revealed a consensus that there was a crisis of reproducibility, with most researchers reporting that they failed to reproduce not only the experiments of other scientists (70%) but even their own (>50%). In biomedical research, reproducibility testing is still extremely limited, with some attempts to do so failing to comprehensively or conclusively validate reproducibility and replicability.
Over the years, there have been several efforts to assess and improve reproducibility in biomedical research. However, there is a new front opening in the reproducibility crisis, this time in ML-based science. According to this study, the increasing adoption of complex ML models is creating widespread data leakage resulting in “severe reproducibility failures,” “wildly overoptimistic conclusions,” and the inability to validate the superior performance of ML models over conventional statistical models.
Pharmaceutical companies have generally been cautious about accepting published results for a number of reasons, including the lack of scientifically reproducible data. An inability to reproduce and replicate preclinical studies can adversely impact drug development and has also been linked to drug and clinical trial failures.
As drug development enters its latest innovation cycle, powered by computational in silico approaches and advanced AI-CADD integrations, reproducibility represents a significant obstacle to converting biomedical research into real-world results.
Reproducibility In In Silico Drug Discovery
The increasing computation of modern scientific research has already resulted in a significant shift with some journals incentivizing authors and providing badges for reproducible research papers. Many scientific publications also mandate the publication of all relevant research resources, including code and data. In 2020, eLife launched Executable Research Articles (ERAs) that allowed authors to add live code blocks and computed outputs to create computationally reproducible publications.
However, creating a robust reproducibility framework to sustain in silico drug discovery would require more transformative developments across three key dimensions: infrastructure/incentives for reproducibility in computational biology, reproducible ecosystems in research, and reproducible data management.
Reproducible computational biology
This approach to industry-wide transformation envisions a fundamental cultural shift with reproducibility as the fulcrum for all decision-making in biomedical research. The focus is on four key domains. First, creating courses and workshops to expose biomedical students to specific computational skills and real-world biological data analysis problems and impart the skills required to produce reproducible research. Second, promoting truly open data sharing, along with all relevant metadata, to encourage larger-scale data reuse. Three, leveraging platforms, workflows, and tools that support the open data/code model of reproducible research. And four, promoting, incentivizing, and enforcing reproducibility by adopting FAIR principles and mandating source code availability.
Computational reproducibility ecosystem
A reproducible ecosystem should enable data and code to be seamlessly archived, shared, and used across multiple projects. Computational biologists today have access to a broad range of open-source and commercial resources to ensure their ecosystem generates reproducible research. For instance, data can now be shared across several recognized, domain and discipline-specific public data depositories such as PubChem, CDD Vault, etc. Public and private code repositories, such as GitHub and GitLab, allow researchers to submit and share code with researchers around the world. And then there are computational reproducibility platforms like Code Ocean that enable researchers to share, discover, and run code.
Reproducible Data Management
As per a recent Data Management and Sharing (DMS) policy issued by the NIH, all applications for funding will have to be accompanied by a DMS plan detailing the strategy and budget to manage and share research data. Sharing scientific data, the NIH points out, accelerates biomedical research discovery through validating research, increasing data access, and promoting data reuse.
Effective data management is critical to reproducibility and creating a formal data management plan prior to the commencement of a research project helps clarify two key facets of the research: one, key information about experiments, workflows, types, and volumes of data generated, and two, research output format, metadata, storage, and access and sharing policies.
The next critical step towards reproducibility is having the right systems to document the process, including data/metadata, methods and code, and version control. For instance, reproducibility in in silico analyses relies extensively on metadata to define scientific concepts as well as the computing environment. In addition, metadata also plays a major role in making data FAIR. It is therefore important to document experimental and data analysis metadata in an established standard and store it alongside research data. Similarly, the ability to track and document datasets as they adapt, reorganize, extend, and evolve across the research lifecycle will be crucial to reproducibility. It is therefore important to version control data so that results can be traced back to the precise subset and version of data.
Of course, the end game for all of that has to be the sharing of data and code, which is increasingly becoming a prerequisite as well as a voluntarily accepted practice in computational biology. One survey of 188 researchers in computational biology found that those who authored papers were largely satisfied with their ability to carry out key code-sharing tasks such as ensuring good documentation and that the code was running in the correct environment. The average researcher, however, would not commit any more time, effort, or expenditure to share code. Plus, there still are certain perceived barriers that need to be addressed before the public archival of biomedical research data and code becomes prevalent.
The future of reproducibility in drug discovery
A 2014 report from the American Association for the Advancement of Science (AAAS) estimated that the U.S. alone spent approximately $28 billion yearly on irreproducible preclinical research. In the future, a set of blockchain-based frameworks may well enable the automated verification of the entire research process.
Meanwhile, in silico drug discovery has emerged as one of the maturing innovation areas in the pharmaceutical industry. The alliance between pharmaceutical companies and research-intensive universities has been a key component in de-risking drug discovery and enhancing its clinical and commercial success. Reproducibility-related improvements and innovations will help move this alliance to a data-driven, AI/ML-based, in silico model of drug discovery.
Subscribe to our Blog and get new articles right after publication into your inbox.