Streamlining in silico drug discovery with cloud computing
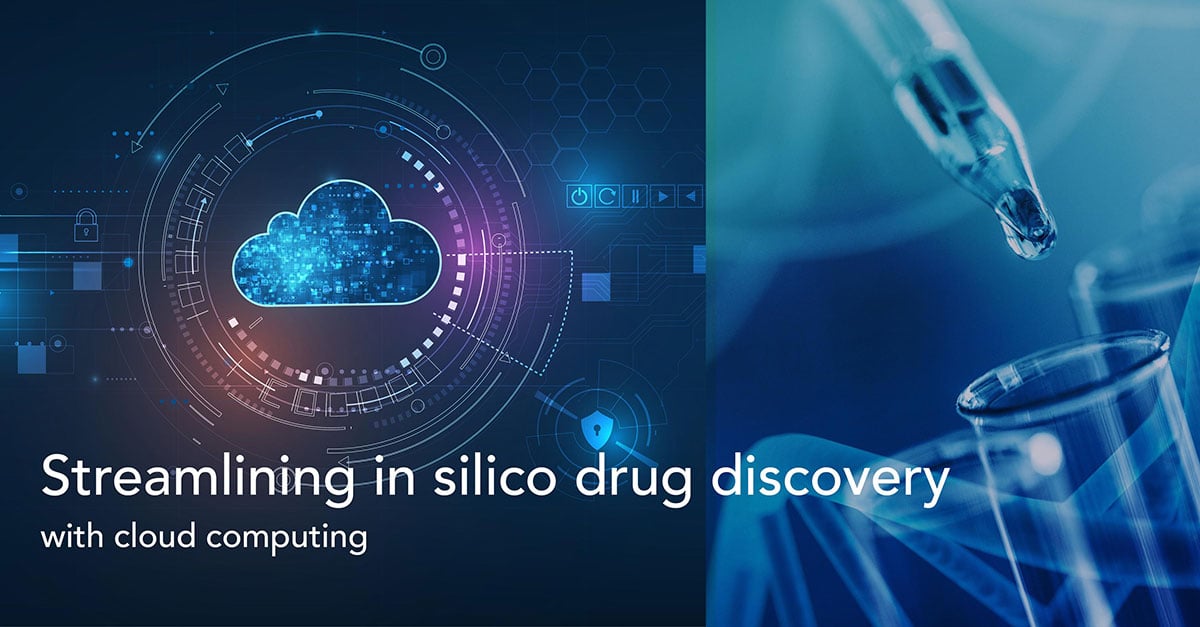
In 2020, seventeen pharmaceutical companies came together in an alliance called QuPharm to explore the potential of quantum computing (QC) technology in addressing real-world life science problems. The simple reason for this early enthusiasm, especially in a sector widely seen as being too slow to embrace technology, is QC’s promise to solve unsolvable problems.
The combination of high-performance computing (HPC) and advanced AI more or less represents the cutting-edge of drug discovery today. However, the sheer scale of the drug discovery space can overwhelm even the most advanced HPC resources available today.
There are an estimated 1063 potential drug-like molecules in the universe. Meanwhile, caffeine, a molecule with just 24 atoms, is the upper limit for conventional HPCs. QC can help bridge this great divide between chemical diversity and conventional computing. In theory, a 300-qubit quantum computer can instantly perform as many calculations as there are atoms in the visible universe (1078-1082).
And QC is not all theory, though much of it is still proof-of-concept. Just last year, IBM launched a new 433-qubit processor, more than tripling the qubit count in just a year. This March witnessed the deployment of the first quantum computer in the world to be dedicated to healthcare, though the high-profile cafeteria installation was more to position the technology front-and-center for biomedical researchers and physicians. Most pharmaceutical majors, including Biogen, Boehringer Ingelheim, Roche, Pfizer, Merck, and Janssen, have also launched their own partnerships to explore quantum-inspired applications.
If QC is the next digital frontier in pharma R&D, the combination of AI and HPC is currently the principal engine accelerating drug discovery, with in silico drug discovery emerging as a key AI innovation area. Computational in silico approaches are increasingly used alongside conventional in vivo and in vitro models to address issues related to the scale, time, and cost of drug discovery.
AI, HPC & in silico drug discovery
According to Gartner, AI is one of the top workloads driving infrastructure decisions. Cloud computing provides businesses with cost-effective access to analytics, compute, and storage facilities and enables them to operationalize AI faster and with lower complexity.
When it comes to HPCs, data-intensive AI workloads are increasingly being run in the cloud, a market that is growing twice as fast as on-premise HPC. From a purely economic perspective, the cloud can be more expensive than on-premise solutions for workloads that require a large HPC cluster. For some pharma majors, this alone is reason enough to avoid a purely cloud-based HPC approach and instead augment on-premise HPC platforms with the cloud for high-performance workloads. In fact, a hybrid approach seems to be the preferred option for many users with the cloud being used mainly for workload surges rather than for critical production.
However, there are several ways in which running AI/ML workloads on cloud HPC systems can streamline in silico drug discovery.
In silico drug discovery in the cloud
The presence of multiple data silos, the proliferation of proprietary data, and the abundance of redundant/replicated data are some of the biggest challenges currently undermining drug development. At the same time, incoming data volumes are not only growing exponentially but also becoming more heterogeneous as information is generated across different modalities and biological layers. The success of computational drug discovery will depend on the industry’s ability to generate solutions that can scale across an integrated view of all this data. Leveraging a unified data cloud as a common foundation for all data and analytics infrastructure can help streamline every stage of the data lifecycle and improve data usage, accessibility, and governance.
As AI adoption in the life sciences approaches the tipping point, organizations can no longer afford to have discrete strategies for managing their data clouds and AI clouds. Most companies today choose their data cloud platform based on the support available for AI/ML model execution.
Drug development is a constantly changing process and AI/ML-powered in silico discovery represents a transformative new opportunity in computer-aided drug discovery. Meanwhile, AI-driven drug discovery is itself evolving dramatically with the emergence of computationally intensive deep learning models and methodologies that are redefining the boundaries of state-of-the-art computation. In this shifting landscape, a cloud-based platform enables life sciences companies to continuously adapt and upgrade to the latest technologies and capabilities.
Most importantly, a cloud-first model can help streamline the AI/ML life cycle in drug discovery.
Data collection for in silico drug discovery covers an extremely wide range, from sequence data to clinical data to real-world data (RWD) to unstructured data from scientific tests. The diverse, distributed nature of pharmaceutical big data often poses significant challenges to data acquisition and integration. The elasticity and scalability of cloud-based data management solutions help streamline access and integrate data more efficiently.
In the data preprocessing phase, a cloud-based solution can simplify the development and deployment of end-to-end pipelines/workflows and enhance transparency, reproducibility, and scalability. In addition, several public cloud services offer big data preprocessing and analysis as a service.
On-premise solutions are a common approach to model training and validation in AI-driven drug discovery. Apart from the up-front capital expenditure and ongoing maintenance costs, this approach can also affect the scalability of the solution across an organization's entire research team, leading to long wait times and loss of productivity. A cloud platform, on the other hand, instantly provides users with just the right amount of resources needed to run their workloads.
And finally, ensuring that end users have access to the AI models that have been developed is the most critical phase of the ML lifecycle. Apart from the validation and versioning of models, model management and serving has to address several broader requirements, such as resilience and scalability, as well as specific factors, such as access control, privacy, auditability, and governance. Most cloud services offer production-grade solutions for serving and publishing ML models.
The rise of drug discovery as a service
According to a 2022 market report, the increasing usage of cloud-based technologies in the global in-silico drug discovery sector is expected to drive growth at a CAGR of nearly 11% between 2021 and 2030, with the SaaS segment forecast to develop the fastest at the same rate as the broader market.
As per another report, the increasing adoption of cloud-based applications and services by pharmaceutical companies is expected to propel AI in the drug discovery market at a CAGR of 30% to $2.99 billion by 2026.
Cloud-based AI-driven drug discovery has well and truly emerged as the current state-of-the-art in pharma R&D. At least until quantum computing and quantum AI are ready for prime time.
Subscribe to our Blog and get new articles right after publication into your inbox.