AI, NLP and the ROI of drug development
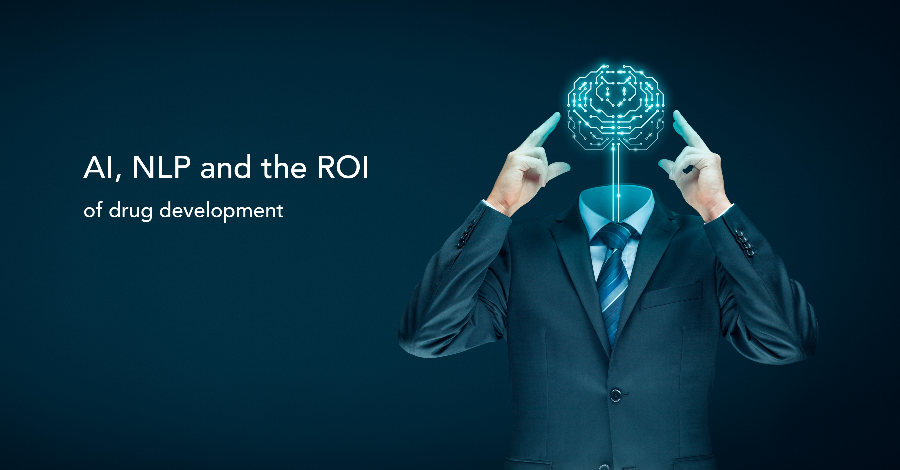
The out-of-pocket R&D costs of developing a single drug, whether successfully launched or not, are estimated to be between $280–$380 million. Less than 14% of active drugs tested in clinical trials are finally approved. Accounting for the out-of-pocket R&D costs of drugs that do not make it to market more than triples the cost of development of a single approved drug to between $1.2– $1.7 billion. Since the average R&D cycle in the pharma and life sciences industry is fourteen years, from hit identification to approval and launch, is 14 years, capital costs constitute a significant proportion of the total R&D costs. Add the cost of capital to this mix and the average R&D cost for a single approved drug more than doubles again to between $2.4–$3.2 billion.
This time-consuming and cost-intensive model of drug development has obvious price, affordability and accessibility implications. Combine this with the low productivity rate of drug development and you have a scenario where, as one study concluded, expected financial return ultimately determines which drugs are developed up to launch.
This ROI-focused model of development can have a more far-reaching and long-term impact not only on the dynamics of drug development but on the healthcare ecosystem as a whole. Using revenue potential as the key metric for development could result in fewer novel drugs being launched. This also means that important segments, like non-life-threatening diseases and areas with existing suboptimal treatments, are deprived of investments and innovation.
The challenge, therefore, is to make pharma R&D more cost-effective, resource-efficient and productive so that more compounds break through conventional ROI thresholds. And this is where AI technologies are playing a central role in transforming conventional drug discovery and development processes.
AI in drug development - an overview
Given the ever-increasing ever-increasing volume, heterogeneity and complexity of data being generated by the pharma sector, AI technologies are expected to have the greatest impact on the pharmaceutical industry according to a survey of industry professionals. There is currently a wave of global AI-first drug discovery startups, 163 as per one count, promising to revolutionise drug discovery and development.
Several of these AI-first drug discovery companies have already demonstrated the value of these technologies by progressing molecules into clinical trials at significantly accelerated timelines and lower costs. One analysis of small-molecule drug discovery at 20 ‘AI-native’ drug discovery companies revealed rapid pipeline growth, at an average annual rate of around 36%, with their combined pipelines comprising nearly 160 disclosed discovery programmes and preclinical assets and about 15 assets in clinical development. This combined pipeline was the equivalent of 50% of the in-house discovery and preclinical output of the top 20 big pharma companies. Meanwhile, big pharma itself is committing to R&D-wide AI deployments with investments strategically distributed across in-house capabilities, M&As and technology partnerships.
In this article, we will focus on NLP, rather than broader AI technologies, for the simple reason that they unlock the value in an abundant yet often neglected data resource - text data.
NLP and drug development ROI
When it comes to data-driven drug discovery, most conversations focus on the explosion of omics and other structured biomedical data generated by NGS platforms. However, nearly 80% of new data in the pharmaceutical and life sciences industries are in the form of unstructured text. The lack of the right technologies and tools to unlock the valuable information embedded in text data represents a missed opportunity to identify novel biomarkers, improve clinical trial design and enhance patient safety. Adding the opportunity cost of all this unused or underused data to drug development ROI calculations will only make a bad situation even worse.
Biomedical-domain-specific NLP techniques can enhance the efficiency, coverage and value of their drug development programs by automating the extraction of statistical and biological information from large volumes of text including scientific literature and medical/clinical data.
AI-powered language models (LMs) in particular have shown the potential to unlock new possibilities for faster, cheaper, and more effective drug discovery and development. These LMs have applications in different stages of drug discovery and development. For instance, a pharmaceutical scientist who needs to understand the biological role of a protein target to support target identification and validation could use an AI-powered Q&A to aggregate all related information from publicly available literature.
Transformer-based biomedical PLMs, or BPLMs, such as BioBERT, BioELECTRA and BioALBERT, currently represent the start of the art for biomedical NLP. Today, there are over 40 transformer-based BPLMs that have become the preferred choice for every biomedical NLP task. Take the prediction of novel drug-target interactions (DTIs), a critical yet expensive, time-consuming and low-efficiency phase in drug discovery. Transformer-based language models can efficiently and accurately extract semantic and syntactic information from vast volumes of biological data and segregate interactions between drug-target pairs as active, inactive, and intermediate.
BioNLP is also playing a critical role in automating model-informed drug development (MIDD), an approach that prioritises the integration of information from diverse data sources to help decrease uncertainty and lower failure rates. In the context of MIDD, biomedical NLP frameworks automate the extraction of structured (e.g. EHRs) and unstructured (e.g. scientific journals) information data and help optimize and accelerate the drug development lifecycle.
The use of AI-powered methodologies for drug repurposing, or repositioning, is also gaining attention in the industry. Not only does this approach have several advantages, such as reduction of development time, risk and cost, but it could also be the key to addressing diseases and conditions that lack adequate attention or investments.
Computational drug repurposing covers a range of data resources, including omics data, biomedical knowledge bases and literature, and EHRs. EHR-based drug repurposing has been specifically identified as a cost-effective opportunity for drug development. They represent an invaluable source of large-scale longitudinal, diagnostic and pathophysiological data that offers real-world perspectives rooted in clinical care. This means that a large number of drug repurposing hypotheses, based on large patient population data sets accumulated over the years, can be tested in parallel.
The challenge in this context is that over half of the information stored in EHRs is in the form of unstructured text such as provider notes, operation reports etc. However, neural network and deep learning-based approaches to NLP can now outperform conventional statistical and rule-based systems on a variety of EHR workflows.
Bridging the knowledge gap in drug discovery
AI technologies have potential applications across the entire drug lifecycle and can play a central role in addressing many of the productivity and efficiency challenges associated with pharma R&D. However, the inability to integrate unstructured data, be it from EHRs or scientific publications, is one of the biggest challenges in drug development. The predominant focus on structured data and the underutilization of text data have resulted in a vast knowledge gap in the conventional drug development process. NLP technologies may not be the solution to all of the industry’s R&D problems. But the ability to integrate what is essentially 80% of all incoming pharmaceutical and life sciences data will definitely have a material and more than incremental impact on the ROI of pharma R&D.
Subscribe to our Blog and get new articles right after publication into your inbox.