Transforming in silico drug discovery with AI
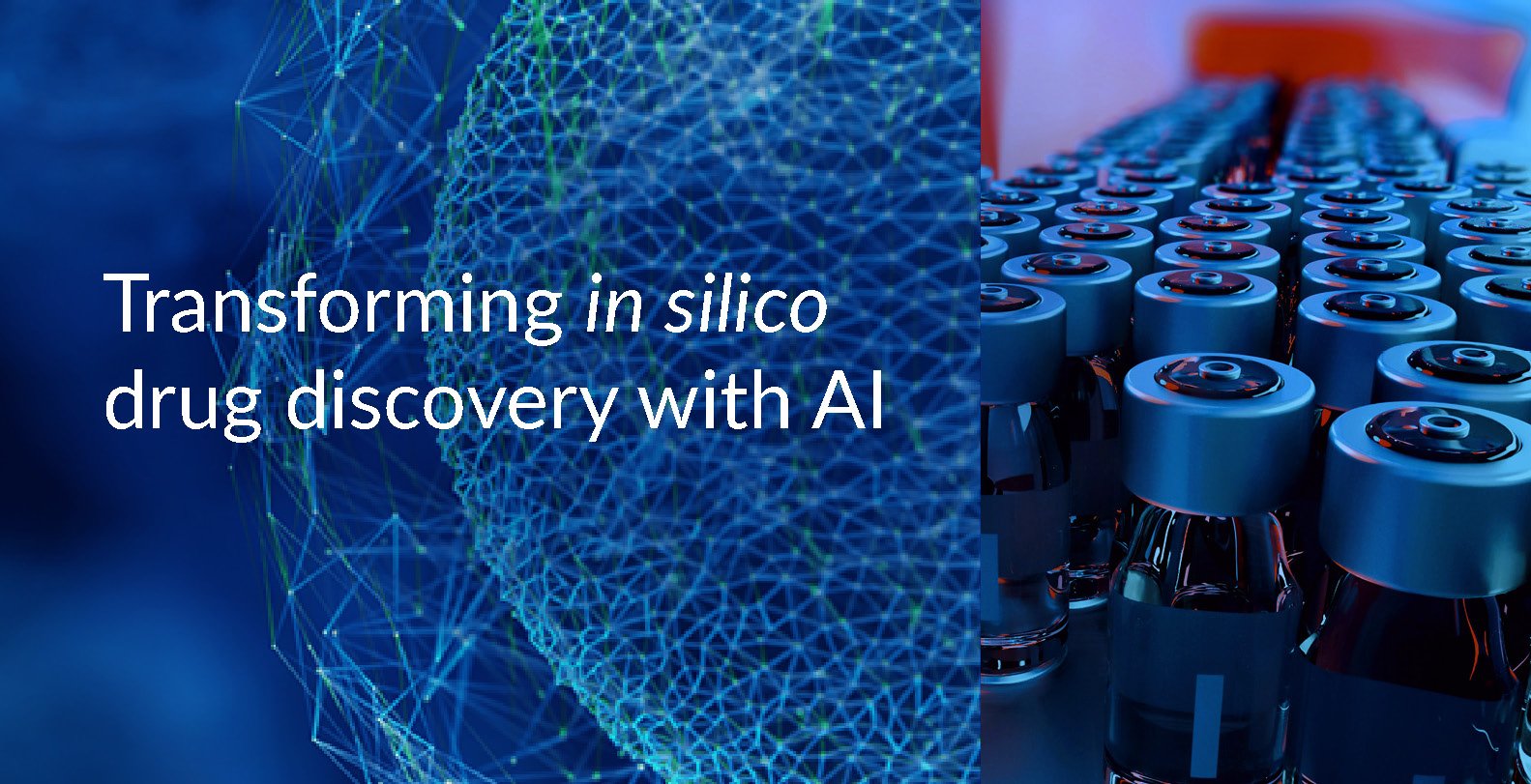
Identifying and validating optimal biological targets is a critical first step in drug discovery with a cascading downstream impact on late-stage trials, efficacy, safety, and clinical performance. Traditionally, this process required the manual investigation of biomedical data to establish target-disease associations and to assess efficacy, safety, and clinical/commercial potential.
However, the exponential growth in high-throughput data on a range of putative targets, including proteins, metabolites, DNAs, RNAs, etc., has led to the increasing use of in silico, or computer-aided drug design (CADD), methods to identify bioactive compounds and predict binding affinities at scale. Today, in silico techniques are evolving at the same pace as in vitro technologies, such as DNA-labelled libraries, and have proven to be critical in dealing with modern chemical libraries' scale, diversity, and complexity.
CADD techniques encompass structure-based drug design (SBDD) and ligand-based drug design (LBDD) strategies depending on the availability of the three-dimensional biological structure of the target of interest. Some of the most common applications for these techniques include in silico structure prediction, refinement, modelling and target validation. They are widely utilised across four phases: identifying hits with virtual screening (VS), investigating the specificity of selected hits through molecular docking, predicting ADMET properties and further molecular optimisation of hits/leads.
As drug discovery becomes increasingly computational and data-driven, it is becoming common practice to combine CADD with advanced technologies like Artificial Intelligence (AI), Machine Learning (ML), and Deep Learning (DL) to cost- and time-efficiently convert biological big data into pharmaceutical value.
In this article, we’ll take a closer look at how AI/ML/DL technologies are transforming three of the most widely used in silico techniques in drug discovery, virtual screening (VS), molecular docking and molecular dynamics (MD) simulation.
Virtual Screening
Virtual screening (VS), a computational approach to screening large libraries for hits, when integrated with an experimental approach, such as high-throughput screening, can significantly enhance the speed, accuracy and productivity of drug discovery. In silico screening techniques are classified as ligand-based VS (LBVS) and structure-based VS (SBVS). These distinct approaches can be combined, for instance, to identify active compounds using ligand-based techniques and follow through with structure-based methods to find favourable candidates. However, there are some shortcomings to CADD-based VS technologies with biochemical assays typically confirming desired bioactivity in only 12% of the top-scoring compounds derived from standard VS applications.
Over the past two decades, the application of AI/ML tools to virtual screening has evolved considerably with techniques like multi-objective optimization and ensemble-based virtual screening being used to enhance the efficiency, accuracy and speed of conventional SBVS and LBVS methodologies. Studies show that deep learning (DL) techniques perform significantly better than ML algorithms across a range of tasks including target prediction, ADMET properties prediction and virtual screening. DL-based VS frameworks have proven to be more effective at extracting high-order molecule structure representations, accurately classifying active and inactive compounds, and enabling ultra-high-throughput screening.
The integration of quantum computing is expected to be the next inflexion point for VS, with studies demonstrating that quantum classifiers can significantly outperform classical ML/DL-based VS.
Molecular Docking
Molecular docking, a widely used method in SBVS for retrieving active compounds from large databases, typically relies on a scoring function to estimate binding affinities between receptors and ligands. This docking-scoring approach is an efficient way to quickly evaluate protein–ligand interactions (PLIs) based on a ranking of putative ligand binding poses that is indicative of binding affinity.
The development of scoring functions (SFs) for binding affinity prediction has been evolving since the 90s and today includes classical SFs, such as physics-, regression-, and knowledge-based methods, and data-driven models, such as ML- and DL-based SFs. However, accuracy is a key challenge with high-throughput approaches as binding affinity predictions are derived from a static snapshot of the protein-ligand binding state rather than the complex dynamics of the ensemble.
ML-based SFs perform significantly better than classical SFs in terms of comparative assessment of scoring functions (CASF) benchmarks and their ability to learn from PLI data and deal with non-linear relationships. But the predictions are based on approximations and data set biases rather than the interatomic dynamics that guide binding. The performance of ML-based SFs also depends on the similarity of targets across the training set and the test set, which makes generalisation a challenge.
DL-based SFs have demonstrated significant advantages, including feature generation automation and the ability to capture complex binding interactions, over traditional ML methods. Recently, a team of MIT researchers took the novel approach of framing molecular docking as a generative modelling problem to develop DiffDock, a new molecular docking model that delivers a much higher success rate (38%) than state-of-the-art of traditional docking (23%) and deep learning (20%) methods.
Molecular Dynamics Simulations
Since molecular docking methods only provide an initial static protein–ligand complex, molecular dynamics (MD) simulations have become the go-to approach for information on the dynamics of the target. MD simulations capture changes at the molecular and atomistic levels and play a critical role in elucidating intermolecular interactions that are essential to assess the stability of a protein-ligand complex.
There are, however, still several issues with this approach including accuracy-versus-efficiency trade-offs, computational complexity, large timescale requirements and errors due to the underlying force fields. ML techniques have helped address many of these challenges and have proven vital to the development of MD simulations for three reasons: objectivity in model selection, enhanced interpretability due to the statistically coherent representation of structure–function relationships, and the capability to generate quantitative, empirically-verifiable models for biological processes.
Deep learning methods are now emerging as an effective solution to dealing with the terabytes of dynamic biomolecular Big Data generated by MD simulations with other applications including the prediction of quantum-mechanical energies and forces, extraction of free energy surfaces and kinetics, and coarse-grained molecular dynamics.
Shifting the in silico paradigm with AI
A combination of in silico models and experimental approaches has become a central component of early-stage drug discovery, facilitating the faster generation of lead compounds at lower costs and with higher efficiency and accuracy. Advanced AI technologies are a key driver of disruption in in silico drug discovery and have helped address some of the limitations and challenges of conventional in silico approaches. At the same time, they are also shifting the paradigm with their capability to auto-generate novel drug-like molecules from scratch. By one estimate, AI/ML in early-stage drug development could result in an additional 50 novel therapies, a $50 billion market, over a 10-year period.
Subscribe to our Blog and get new articles right after publication into your inbox.