Making sense of multi-omics data
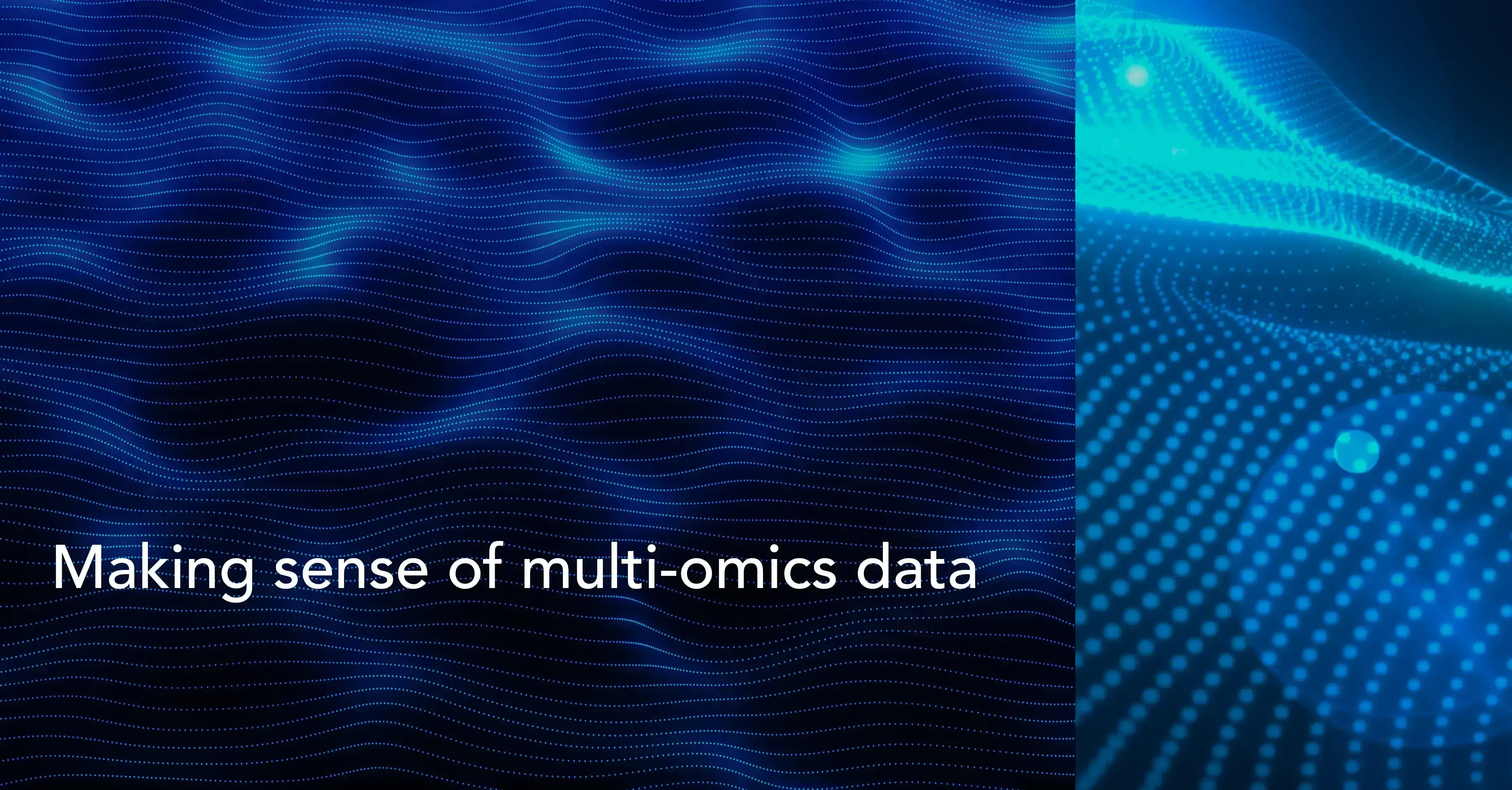
We love multi-omics analysis. It is data-driven. It is continuously evolving and expanding across new modalities, techniques, and technologies. Integrated multi-omics analysis is essential for a holistic understanding of complex biological systems and a foundational step on the road to a systems biology approach to innovation. And it is the key to innovation in biomedical and life sciences research, underpinning antibody discovery, biomarker discovery, and precision medicine, to name just a few.
In fact, if you love multi-omics as much as we do, we have an extensive library of multi-perspective omics-related content just for you.
However, today we will take a closer look at some of the biggest data-related challenges — data integration, data quality, and data FAIRness — currently facing integrative multi-omics analysis.
Data Integration
Over the years, multiomics analysis has evolved beyond basic multi-staged integration, i.e combining just two data features at a time. Nowadays, true multi-level data integration, which transforms all data of research interest from across diverse datasets into a single matrix for concurrent analysis, is the norm.
And yet, multi-omics data integration techniques still span multiple categories based on diverse methodologies with different objectives. For instance, there are two distinct approaches to multi-level data integration: horizontal and vertical integration. The horizontal model is used to integrate omics data of the same type derived from different studies whereas the vertical model integrates different types of omics data from different experiments on the same cohort of samples. Single-cell data integration further expands this classification to include diagonal integration, which further expands the scope of integration beyond the previous two methods, and mosaic integration, which includes features shared across datasets as well as features exclusive to a single experiment.
The increasing use of AI/ML technologies has helped address many previous challenges inherent in multiomics data integration but has only added to the complexity of classification. For instance, vertical data integration strategies for ML analysis are further subdivided into 5 groups based on a variety of factors. Even the classification of supervised and unsupervised techniques covers several distinct approaches and categories.
As a result, researchers today can choose from various applications and analytical frameworks for handling diverse omics data types, and yet not many standardized workflows for integrative data analyses. The biggest challenge, therefore, in multi-omics data integration is the lack of a universal framework that can unify all omics data.
Data Quality
The success of integrative multi-omics depends as much on an efficient and scalable data integration strategy as it does on the quality of omics data. And when it comes to multi-omics research, it is rarely prudent to assume that data values are precise representations of true biological value. There are several factors, between the actual sampling to the measurement, that affect the quality of a sample. This applies equally to data generated from manual small-scale experiments and from sophisticated high-throughput technologies.
For instance, there can be intra-experimental quality heterogeneity where there is variation in data quality even when the same omics procedure is used to conduct a large number of single experiments simultaneously. Similarly, there can also be inter-experimental heterogeneity in which the quality of data from one experimental procedure is affected by factors shared by other procedures. In addition, data quality also depends on the computational methods used to process raw experimental data into quantitative data tables.
An effective multi-omics analysis solution must have first-line data quality assessment capabilities to guarantee high-quality datasets and ensure accurate biological inferences. However, there are currently few classification or prediction algorithms that can compensate for the quality of input data. However, in recent years there have been efforts to harmonize quality control vocabulary across different omics and high-throughput methods in order to develop a unified framework for quality control in multi-omics experiments.
Data FAIRness
The ability to reuse life sciences data is critical for validating existing hypotheses, exploring novel hypotheses, and gaining new knowledge that can significantly advance interdisciplinary research. Quality, for instance, is a key factor affecting the reusability of multi-omics and clinical data due to the lack of common quality control frameworks that can harmonize data across different studies, pipelines, and laboratories.
The publishing of the FAIR principles in 2016 represented one of the first concerted efforts to focus on improving the quality, standardization, and reusability of scientific data. The FAIR Data Principles, designed by a representative set of stakeholders, defined measurable guidelines for “those wishing to enhance the reusability of their data holdings” both for individuals and for machines to automatically find and use the data. The four foundational principles — Findability, Accessibility, Interoperability, and Reusability — were applicable to data as well as to the algorithms, tools, and workflows that contributed to data generation.
Since then there have been several collaborative initiatives, such as the EATRIS-Plus project and the Global Alliance for Genomics and Health (GA4GH) for example, that have championed data FAIRness and advanced standards and frameworks to enhance data quality, harmonization, reproducibility, and reusability. Despite these efforts, the use of specific and non-standard formats continues to be quite common in the life sciences.
Integrative multi-omics - The BioStrand Model
Our approach to truly integrated and scalable multi-omics analysis is defined by three key principles. One, we have created a universal and automated framework, based on a proprietary transversal language called HYFTs®, that has pre-indexed and organized all publicly available biological data into a multilayered multidimensional knowledge graph of 660 million data objects that are currently linked by over 25 billion relations. We then further augmented this vast and continuously expanding knowledge network, using our unique LENSai Integrated Intelligence Platform, to provide instant access to over 33 million abstracts from the PubMed biomedical literature database. Most importantly, our solution enables researchers to easily integrate proprietary datasets, both sequence- and text-based data. With our unique data-centric model, researchers can integrate all research-relevant data into one distinct analysis-ready data matrix mosaic.
Two, we combined a simple user interface with a universal workflow that allows even non-data scientists to quickly explore, interrogate, and correlate all existing and incoming life sciences data.
And three, we built a scalable platform with proven Big Data technologies and an intelligent, unified analytical framework that enables integrative multi-omics research.
In conclusion, if you share our passion for integrated multi-omics analysis, then please do get in touch with us. We’d love to compare notes on how best to realize the full potential of truly data-driven multi-omics analysis.
Subscribe to our Blog and get new articles right after publication into your inbox.