Integrated multi-omics analysis: From data normalisation to biological insight
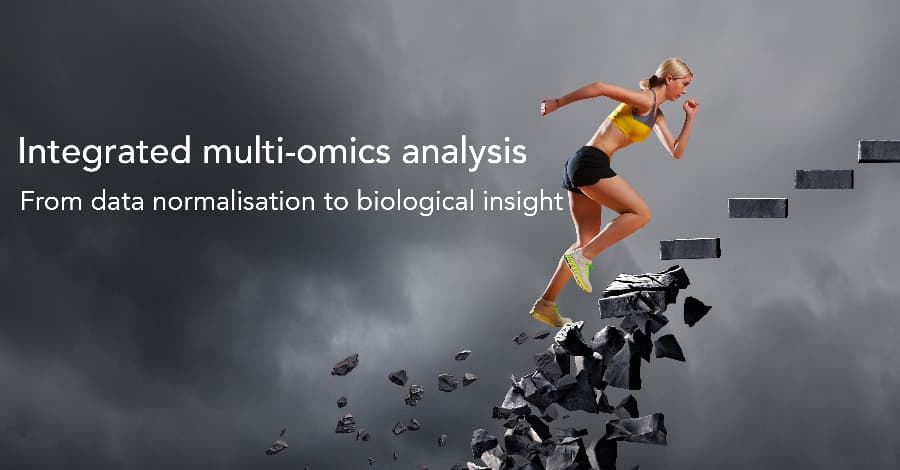
The exponential generation of data by modern high-throughput, low-cost next generation sequencing (NGS) technologies is set to revolutionise genomics and molecular biology and enable a deeper and richer understanding of biological systems. And it is not just about more volumes of highly accurate, multi-layered data. It’s also about more types of omics datasets, such as glycomics, lipidomics, microbiomics, and phenomics.
The increasing availability of large-scale, multidimensional and heterogeneous datasets has the potential to open up new insights into biological systems and processes, improve and increase diagnostic yield, and pave the way to shift from reductionist biology to a more holistic systems biology approach to decoding the complexities of biological entities.
It has already been established that multi-dimensional analysis – as opposed to single layer analyses – yields better results from a statistical and a biological point of view, and can have a transformative impact on a range of research areas, such as genotype-phenotype interactions, disease biology, systems microbiology, and microbiome analysis.
However, applying systems thinking principles to biological data requires the development of radically new integrative techniques and processes that can enable the multi-scale characterisation of biological systems. Combining and integrating diverse types of omics data from different layers of biological regulation is the first computational challenge – and the next big opportunity – on the way to enabling a unified end-to-end workflow that is truly multi-omics.
The challenge is quite colossal – indeed, a 2019 article in the Journal of Molecular Endocrinology refers to the successful implementation of more than two datasets as very rare.
Data integration challenges in multi-omics
Analysing omics datasets at just one level of biological complexity is challenging enough. Multi-omics analysis amplifies those challenges and introduces some unfamiliar new complications around data integration/fusion, clustering, visualisation, and functional characterisation.
For instance, accommodating for the inherent complexity of biological systems, the sheer number of biological variables and the relatively low number of biological samples can on its own turn out to be a particularly difficult assignment. Over and above this, there is a litany of other issues including process variations in data cleaning and normalisation, data dimensionality reduction, biological contextualisation, biomolecule identification, statistical validation, etc., etc., etc.
Data heterogeneity, arguably the raison d'être for integrated omics, is often the primary hurdle in multi-omics data management. Omics data is typically distributed across multiple silos defined by domain, type, and access type (public/proprietary), to name just a few variables. More often than not, there are significant variations between datasets in terms of the technologies/platforms that were used to generate these datasets, nomenclature, data modalities, assay types, etc. Data harmonisation, therefore, becomes a standard pre-integration process.
But the process for data scaling, data normalisation, and data transformation to harmonise data can vary across different dataset types and sources. For example, there is a difference between normalisation and scaling techniques between RNA-Seq datasets and small RNA-Seq datasets.
Multi-omics data integration has its own set of challenges, including lack of reliability in parameter estimation, preserving accuracy in statistical inference, and/or the prevalence of large standard errors. There are, however, several tools currently available for multi-omics data integration, though they come with their own limitations. For example, there are web-based tools that require no computational experience – but the lack of visibility into their underlying processes makes it a challenge to deploy them for large-scale scientific research. On the other end of the spectrum, there are more sophisticated tools that afford more customisation and control – but also require considerable expertise in computational techniques.
In this context, the development of a universal standard or unified framework for pre-analysis, let alone an integrated end-to-end pipeline for multi-omics analysis, seems rather daunting.
However, if multi-omics analysis is to yield diagnostic value at scale, it is imperative that it quickly evolves from being a dispersed syndicate of tools, techniques and processes to a new integrated multi-omics paradigm that is versatile, computationally feasible and user-friendly.
A platform approach to multi-omics analysis
The data integration challenge in multi-omics essentially boils down to this. There either has to be a technological innovation designed specifically to handle the fine-grained and multidimensional heterogeneity of biological data. Or, there has to be a biological discovery that unifies all omics data and makes them instantly computable even for conventional technologies.
At BioStrand, we took the latter route and came up with HYFTs™, a biological discovery that can instantly make all omics data computable.
Normalising/Integrating data with HYFTs™
We started with a new technique for indexing cellular blueprints and building blocks and used it to identify and catalogue unique signature sequences, or biological fingerprints, in DNA, RNA, and AA that we call HYFT™ patterns. Each HYFT™ comprises multiple layers of information, relating to function, structure, position, etc., that together create a multilevel information network.
We then designed a BioStrand parser to identify, collate and index HYFTs™ from over 450 million sequences available across 11 popular public databases. This helped us create a proprietary pangenomic knowledge database using over 660 million HYFT™ patterns containing information about variation, mutation, structure, and more.
Based on our biological discovery, we were able to normalise and integrate all publicly available omics data, including patent data, at scale, and render them multi-omics analysis-ready. The same HYFT™ IP can also be applied to normalise and integrate proprietary omics data.
Making 660 million data points accessible
That’s a lot of data points. So, we made it searchable. With Google-like advanced indexing and exact matching technologies, only exact matches to search inputs are returned. Through a simple search interface – use plain text or a FASTA file – researchers can now accurately retrieve all relevant information about sequence alignments, similarities, and differences from a centralised knowledge base with information on millions of organisms in just 3 seconds.
Synthesising knowledge with our AI-powered SaaS platform
Around these core capabilities, we built the BioStrand SaaS platform with state-of-the-art AI tools to expand data management capabilities, mitigate data complexity, and to empower researchers to intuitively synthesise knowledge out of petabytes of biological data. With our platform, researchers can easily add different types of structured and unstructured data, leverage its advanced graph-based data mining features to extract insights from huge volumes of data, and use built-in genomic analysis tools for annotation and variation analysis.
Multi-omics as a platform
As omics data sets become more multi-layered and multidimensional, only a truly sequence integrated multi-omics analysis solution can enable the discovery of novel and practically beneficial biological insights. With BioStrand Platform, delivered as a SaaS, we believe we have created an integrated platform that enables a user-friendly, automated, intelligent, and data-ingestion-to-insight approach to multi-omics analysis. It eliminates all the data management challenges associated with conventional multi-omics analysis solutions and offers a cloud-based platform-centric approach to multi-omics analysis that is paramount to usability and productivity.
Subscribe to our Blog and get new articles right after publication into your inbox.