Omics data analysis and precision medicine
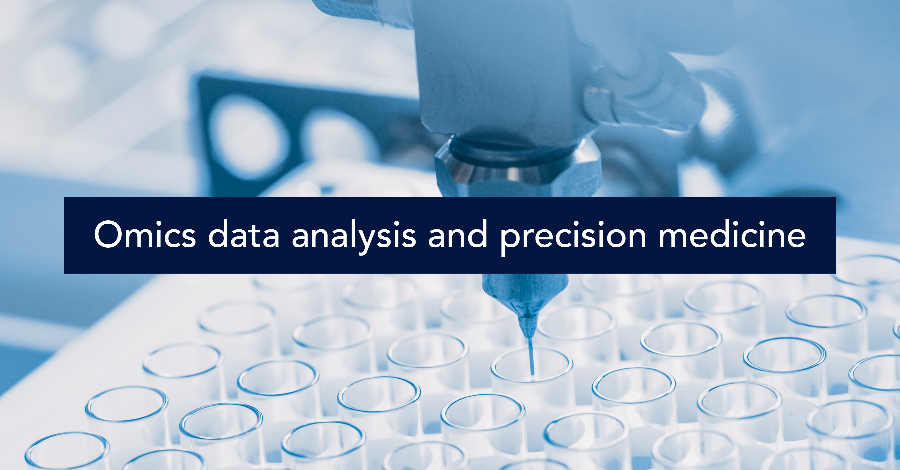
Over the past decades advances in technology have allowed us to gain insight in complex biological systems. Sequencing technologies enabled the characterisation of the genetic code of humans and other organisms. Imaging, microscopic technologies and other advanced methodologies revolutionised our insights in cellular and molecular processes. All this knowledge and research efforts lead to a better understanding of the molecular and cellular mechanisms involved in development and progression of disease.
The ultimate goal of precision medicine according to The European Union’s Horizon 2020 Advisory Group is the ‘‘characterisation of individuals’ phenotypes and genotypes for tailoring the right therapeutic strategy for the right person at the right time, and/or to determine the predisposition to disease and/or to deliver timely and targeted prevention’’. Yet, despite the major progress made in deciphering the genetic code, the implementation of precision medicine approaches in clinical practice shows to be challenging.
The recent history of ‘genomic’ medicine
The Human Genome Project, completed in 2003, resulted in the sequencing of the entire human genome and its hierarchical assembly and has been crucial to driving scientific activity.
Further technological progress led to the development of high-density DNA micro-arrays and next generation sequencing. The availability of these technologies, which over the years showed a steep decline in costs, allowed for a huge number of human individuals to be genotyped.
An example is the 100.000 Genomes Project, launched in the UK in 2012, with the target of sequencing 100.000 whole genomes by 2017 following the principles of ethics, aiming to benefit patients, and create new medical insights. Via genome-wide association studies unique and robust associations between common variants and common diseases were discovered, in 2018 nearly 90,000 of these associations were described. In the past decade, The Cancer Genome Atlas (TCGA) has been comprehensively cataloging the genes and mutations that can serve as ‘drivers’ of oncogenesis, essentially by exome or genome sequencing of thousands of tumour-normal matched sample pairs. A recent analysis across the entire TCGA dataset identified a consensus list of 299 driver genes of common cancers.
These and multiple other projects have created several opportunities for the funding of the genomic industry and moved research, and the medical field as a whole, forward.
Where are we now?
The whole healthcare sector is moving from conventional treatments to precision medicine, not only in cancer but also in cardiovascular disease and metabolic disease.
Best known in precision medicine is the use of genomic data. Genetic profiling and detection of predictive biomarkers can identify individuals at risk for a specific disease or a severe variant of a disease. Preventive interventions might be considered.
Today, more than 54,000 tests are available for over 16,400 genes. For example, the availability of non-invasive prenatal testing or NIPT (screening for foetal trisomies) has had a large impact on clinical practice. So far, most effort is put in the analysis of protein coding regions of the genome. Information on mutations in the non-coding regions, which span 98% of the human genome is limited.
Detection of biomarkers is important as a companion diagnostic to select the most appropriate treatment, patients with certain characteristics will benefit more from treatment A instead of B. Biomarkers can also indicate who will benefit the most from a certain treatment or detect patients who have a higher risk of adverse events. Biomarkers are also used to monitor treatment response and recurrence of disease.
Transcriptome data have revealed how genetic sequence variation may lead to disease by relating certain DNA variations to changes in gene expression. Characteristic gene expression signatures are helpful in predicting outcome and treatment response. Several clinical tests are on the market, for example for predicting recurrence risk in breast cancer and colorectal cancer. Recently, gene expression analysis of single cells has become available. This will greatly improve our knowledge of tumour heterogeneity in cancer and probably further influence our clinical decision making.
The study of regulation of gene expression, epigenomics, has revealed that for some conditions (for example in glioblastoma) the DNA methylation status of a certain region determines the choice of treatment.
The analysis of cellular proteins, proteomics, has for example in cancer led to detection of specific biomarkers or aided in classification and prediction of drug sensitivity and drug resistance.
What are the challenges in omics data analysis related to advancing precision medicine?
Accuracy of omics data analysis has to be improved. Due to the heuristic nature of the currently used algorithms alignment and mapping errors occur. Variation analysis of a whole genome is a cumbersome process. Different so-called ‘mutation call algorithms or pipelines’ have to be used, each detecting different kind of mutations (indels, SNV, structural variations…). Studies comparing multiple pipelines showed too many inconsistencies in results. So, novel algorithms providing more accurate data analysis must be developed.
As highlighted above, omics data reside in silos. Data obtained from one omics approach are often not well correlated with data obtained by other methods. Thus, integration of knowledge stemming from different domains and assessing different parts of the complex pathophysiology of disease development and progression remains difficult. As a result, we only have an incomplete picture of the underlying biology.
It has become clear that precision medicine is more than the use of genetic variant information to inform clinical practice. Yet, making full and integrated use of all multi-omics approaches to achieve progress in precision medicine is still in its infancy.
In addition to the generation of multi-omics datasets, real world data and detailed patient data have to be integrated. In order to extract biological and clinically relevant information from these big datasets, computational methods that excel in performance and interpretability are needed. To make precision medicine really ‘bed-side available’, we need excellent predictive power combined with easy interpretability. Further analyses of which machine learning techniques are best suited for this purpose will have to be made and further research and development on paradigms such as patient similarity networks (PSN) will have to take place.
Another challenge is the computational power needed to do all the data analysis and modelling. Also, in this area, further developments are needed. On the one hand hardware optimisations are needed but on the other hand also fundamental research into parsimonious yet powerful algorithms.
From the perspective of cost-effectiveness, the challenge will be to determine “if and when” precision medicine provides sufficient value relative to its costs, and will be part of insurance reimbursement.
Last but not least, making progress in precision medicine is not possible without active involvement and participation of patients and consumers. Issues such as truly informed consent, ownership of genetic information, privacy must be carefully addressed. Also in the choice of relevant outcomes patients must participate.
Although many challenges are ahead, technological progress in many domains has never been greater and will allow us to truly advance precision medicine.
Picture source: AdobeStock © ipopba 281210859
Subscribe to our Blog and get new articles right after publication into your inbox.