Key elements of successful AI-native drug discovery
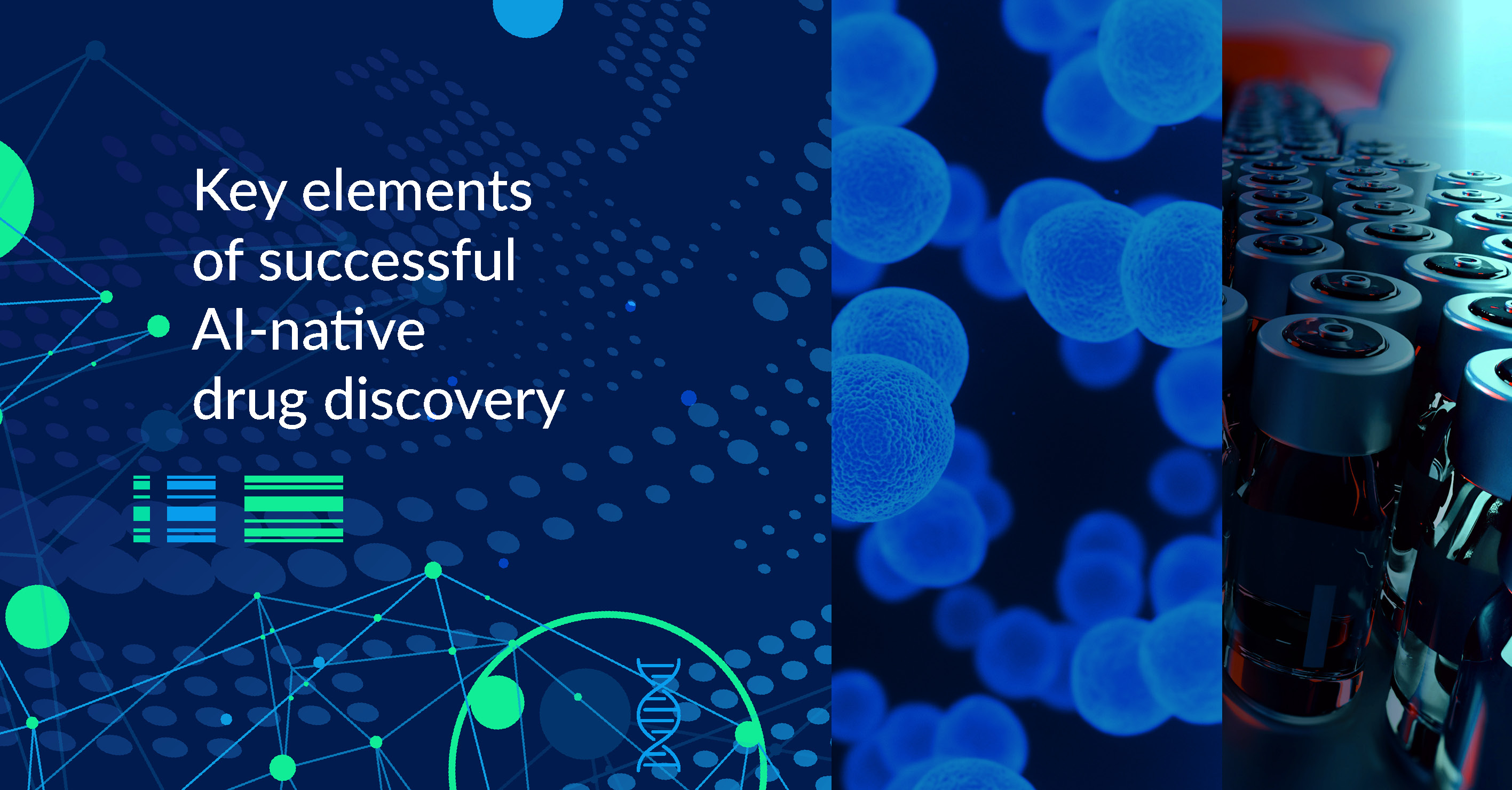
The use of AI in drug discovery has been progressively expanding over the past decade. Over the next decade, according to Morgan Stanley Research, even modest AI/ML-enabled improvements in early-stage drug development success rates could deliver an additional 50 novel therapies and create a $50 billion opportunity.
AI-enabled drug discovery continues to grow at a rapid pace and the industry is currently on the cusp of a new era of AI-native drug discovery.
Take small-molecule drug discovery, for instance. One analysis that reconstructed the pipelines of 20 AI-native drug discovery companies between 2010 and 2021 reported an average annual growth rate of around 36% with a combined total of over 160 discovery programs and preclinical assets and about 15 assets in clinical development. Comparatively, the combined top 20 AI-native pipelines represented the equivalent of 50% of the in-house discovery and preclinical output of the top 20 pharma companies.
And this transformation is not limited to small molecule drug discovery.
Another study of pipeline growth at 14 AI-native biologics companies between 2010 and 2022 revealed a year-on-year growth rate of 40% and a combined pipeline of at least 52 R&D programs and assets. Compared to the pharma top 20, with approximately 580 ongoing biologics R&D programs and assets, the combined AI-native biologics pipeline came in at just under 10%. But that may be the growth story set to unfold over the next 10 years.
Notwithstanding these impressive developments, the conclusive proof of value of AI-native drug discovery will depend on a critical mass of AI-derived small molecules and biologics successfully progressing to the clinical trial stage and their subsequent performance at the point of care. Both the incumbents and the startups will need a concerted set of strategies to ensure that the potential of AI-native drug discovery is translated into practice.
Top 5 drivers of successful AI-first drug discovery
Global consulting firm BCG defines five strategic and operational tracks essential for realizing AI's full potential in drug discovery.
Defining AI Vision and Strategy
Biotech companies have the choice of either leveraging AI to optimize their current discovery program or reinventing it as an AI-first model. In the latter, the focus is on building a core advantage through AI, and then using data learning on accumulated unique data to automatically and constantly strengthen and extend that competitive advantage. The emphasis has to be on defining an AI strategy that is perfectly aligned with business strategy and is connected and coordinated across the enterprise. Quite often, it can be quite challenging to realize the return on investment of AI solutions deployed across discrete, disconnected use cases.
Creating user-friendly data and technology infrastructure
Successful AI-first drug discovery requires a platform-based AI engine, instead of point-to-point data solutions, with end-to-end capabilities that redefine the drug discovery and development value chain. And since data is the foundation of these AI systems, efficient data integration of huge volumes of multimodal, heterogeneous, and often fragmented data will be key to effective AI/ML in drug discovery. Companies need to have the right technology and workflow processes in place to facilitate autonomous error-free data integration across internal and external data silos. And finally, biotech companies need to ensure that their AI platforms democratize access for non-technical domain experts with advanced, pre-trained, and pre-configured AI/ML tools that enable them to explore connections across all data.
Forging AI Partnerships
Partnerships have emerged as the fastest-moving model for accelerating the adoption of AI-enabled drug discovery. Most AI-first biotechs have already adopted an ecosystem approach whereby they focus their in-house capabilities on building expertise in selected areas and forge partnerships to access targeted expertise and experience to expand their suite of offerings. According to Deloitte, organizations with diverse ecosystems are significantly more likely to have a transformative vision for AI, enterprise-wide AI strategies, and use AI as a strategic differentiator.
Managing talent
The increasing importance of technologies like AI in pharma and life sciences has positioned these sectors in direct competition with the technology industry. As a result, attracting and retaining talent is now an existential challenge. Biotechs with a transformative vision for AI will have to adopt a proactive approach to attract new talent as well as developing internal talent. Companies are deploying a range of new tactics, such as creating structured AI training programs, revamping their employee value propositions, and assigning new hires to high-visibility projects, to acquire, upskill and retain scarce AI and data science talent.
Transforming organizational culture
Organizational culture continues to be a significant roadblock to the successful adoption of enterprise-wide AI. In the case of AI-first drug discovery, companies will have to adapt critical processes to the faster pace of in-silico work. A shift in culture for a radically new approach to drug discovery will have to be driven from the top. Company leaders must manage this long process through constant messaging and strategic incentivization. However, just as business culture affects AI deployments, AI deployments also affect business culture. A 2021 MIT SMR-BCG report on AI and business strategy identified a range of AI-related benefits, both financial and cultural, and at the team and organizational levels.
Subscribe to our Blog and get new articles right after publication into your inbox.