AI in Biopharma: Challenges and Opportunities
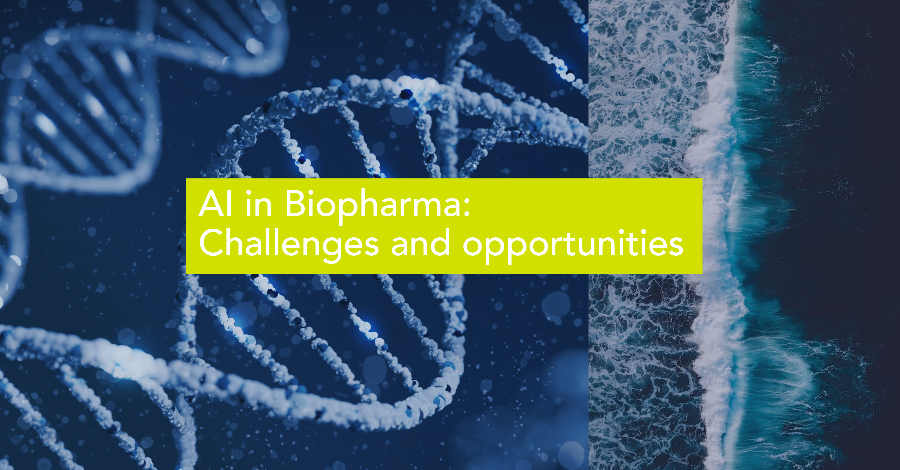
There are an estimated novemdecillion, or 1060, molecules in the known universe with potential drug-like traits that could be developed into life-saving treatments.
This is just one example of the mind-boggling scale that the biopharma industry contends with. Despite advanced computational and automation solutions capable of screening millions of compounds, drug discovery continues to be a time-, resource-, and risk-intensive process. All resulting in an abysmal 1-in-10 shot at success for compounds entering clinical trials.
And then there is the sheer scale of data.
Due to the volume of complex data, DNA sequencing data has been called the poster child of the Big Data frenzy in the industry. Studies show that the rapid evolution of sequencing capabilities will result in generation of one zettabase of sequence per year by 2025.
Yet, this type of data is just one component of biopharma Big Data, as huge volumes of data are generated from electronic health records (EHRs), patient registries, clinical trials, spontaneous adverse drug reaction reports, social media, and a host of other sources.
Take real-world data (RWD) and real-world evidence (RWE) and their potentially transformative applications across the pharmaceutical product lifecycle. In 2020 alone, the healthcare industry generated over 2,314 exabytes of real-world data. But, without the technologies and frameworks to consistently acquire this data, most biopharma companies feel that they lack access to real-world data that would make the analyses valuable.
Then there is the missed opportunity of unused or underused data in the form of unstructured text which, due to the lack of effective technologies and tools, now constitutes nearly 80% of new data in the pharmaceutical and life sciences industries.
Finally, the ongoing R&D shift from innovative small molecules to biologics is creating new challenges in the acquisition and analysis of data across the drug development pipeline.
Conventional technologies can no longer quickly, efficiently, or accurately cope with biopharma’s unique brand of Big Data defined in novemdecillions, zettabases and exabytes. Other than sheer scale, biopharma datasets are also so complex and diverse that AI technologies have become the key to transformation.
AI in biopharma
Biopharma, an industry that was traditionally slow to incorporate innovative digital technologies, is now at a digital innovation inflection point. The pandemic has compelled many biopharma companies to prioritize digital innovation investments and fast-track digital transformation road maps.
The digitalisation of information has been a strategic component of this transformation. Many biopharma companies have made considerable progress in creating an interoperable data backbone of integrated data to support the development continuum. However, achieving similar integration at the industry level remains a challenge given the complex and siloed data landscape and the volumes of knowledge locked away in text records.
The opportunities
AI technologies hold the potential to bring together the volumes of data scattered across the healthcare ecosystem and enable more holistic analyses and decision-making. As a result, industry investments in AI and Big Data technologies have been on the rise in recent years. Today, AI has become a predominant tool in drug discovery, design, and target identification.
For instance, machine learning-powered predictive modelling tools can more accurately and quickly predict drug candidate behaviours and interactions, and maximise the potential for success in early discovery. Advanced machine learning (ML) algorithms are increasingly being used in the formulation design of biological drugs. By combining patterns from large data sets with knowledge from previous campaigns, resource requirements are minimised and development time scales are accelerated.
Biopharma companies are also transitioning from traditional RWE analytics that use descriptive analyses, to analytics that use ML, predictive models, probabilistic causal models, and unsupervised algorithms. These RWE analytics extract deeper insights, “learn” relationships between thousands of variables, and predict outcomes.
The challenges
Despite the wide-ranging potential of AI-driven transformation in biopharma, the process does entail some complex challenges.
First, there are conceptual concerns pertaining to the application of AI itself, including the importance of ensuring diverse training data sets to mitigate biases, and the need for explainable and transparent algorithms that ensure regulatory compliance.
There is also the need for open and transparent data sharing and collaboration in order to realise the promise of AI-augmented biomedical research.
Then there are data management and governance challenges involved with the security, privacy, and intended use of data.
And finally, in addition to ecosystem alliances and AI-related audit and risk management systems, there is the challenge of ensuring that the right IT infrastructure, talent, and skill sets are available to leverage AI for biopharma digital transformation.
Democratisation and collaboration
There are two emergent trends that may serve to collectively address most, if not all, of these challenges.
One report forecasts a significant trend for “AI democratisation” where specialised, cloud-based AI workflow platforms enable access to various pre-trained, pre-configured AI technologies in a simple ready-to-use model. Today, platforms like LENSai Integrated Intelligence Platform provide instant access to vast volumes of biological sequence and text data along with advanced AI/ML tools to explore connections across all data points.
The second trend is toward biopharma AI collaborations. As AI emerges as the key engine for driving transformation and innovation, many biopharma companies are turning to partnerships with specialized AI companies and platforms to translate intelligent technologies into biopharma innovations. The ability to build end-to-end AI partnerships that traverse the entire biopharma value chain will be critical to realising value at scale.
Over the next five years, biologics are forecast to displace small molecules as the primary engines of value creation at biopharma companies. As the industry makes this foundational shift, the combination of integrated, high-quality Big Data and advanced computational technologies such as AI, will provide the framework required to drive innovation across the biopharma value chain – from drug discovery and development, to manufacturing and the management of supply chains.