Efficient data integration is the key to effective AI/ML
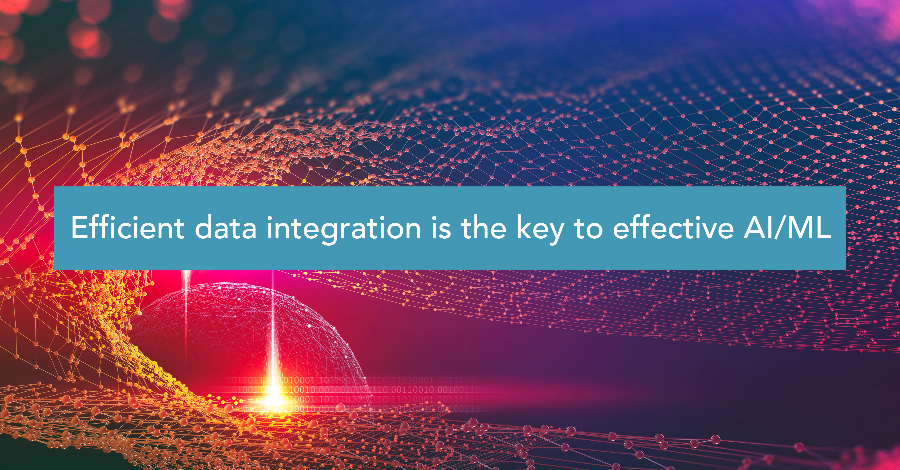
AI/ML technologies can be notoriously data-hungry with the broader concern being that certain domains may struggle to acquire and integrate enough big data for productive analytics.
In the case of genomics, the concern is quite the opposite.
The continuing evolution of 21st century NGS technologies has opened the floodgates for huge volumes of rapid low-cost high-accuracy raw data.
Concurrently, the development of multiple high-throughput technologies is generating a matrix of multimodal omics data - such as epigenomics, transcriptomics, radiomics, proteomics, metabolomics etc., from across distinct but complementary biological layers.
And then there is unstructured data, currently the largest and fastest-growing constituent of the data universe, in the form of textual data from scientific journals, medical & clinical records, social media posts etc. that lie beyond the purview of conventional bioinformatics systems.
Fragmented data, fragmented insights
With just raw sequencing data doubling every seven months, genomics would ideally have been fertile ground for data-hungry technologies. However, genomics also happens to be rife with data fragmentation, one of the biggest cross-industry challenges for data leaders in 2022. Currently, most of the data remain trapped in domain/protocol specific silos with no common language or unified tool environment for horizontal or vertical data integration.
Meanwhile, downstream omics analytics continue to evolve in terms of intelligence, sophistication and scope. And though applying advanced AI/ML technologies to fragmented subsets of biological data may yield some incremental improvements, it essentially is an exercise in extracting fragmented insights from fragmented data.
The success of AI/ML in the life sciences will depend as much on data availability for data-hungry AI engines as it will on training datasets being truly representative of the real-world issues that are being studied. A data-driven holistic understanding of complex biological systems will only be possible if researchers are empowered to seamlessly acquire and integrate data, across multiple domains, data sources, & types.
Unified omics data analytics
At BioStrand we firmly believe that there are two central attributes that will define any next-generation omics analysis platform. One, the technology will enable the smooth, effortless ingestion and integration of all research-relevant data, irrespective of experimental protocol, domain, type, location, characteristic, etc., into one multidimensional single source of truth that is ready for analysis.
And two, it will provide a composable and intelligent analytics framework that can scale across multi- and cross-disciplinary research.
Data unification using HYFTs™
In the context of this article, the scope for data unification covers two broad categories. The first is the unification of all omics data for truly multiomics analysis. The second is the integration of the knowledge embedded in textual data. At BioStrand, we have developed technologies that address the unique integration challenges of each of these data categories.
Single-click multiomics data integration with HYFTs™
HYFTs™ are essentially specific and recurring patterns across multiomics data. Each HYFT™ pattern is a unique signature sequence in DNA, RNA, and AA, based on which all biological sequence data, irrespective of species, structure or function, can be tokenized to a new new transversal language that connects all omics layers.
Every HYFT™ is also a rich data object containing multilevel information about structure, function, pharmacochemical properties, etc.
Using the BioStrand HYFT™ IP, we have successfully unified over a billion sequences from the largest and most popular publicly available databases into one multidimensional model that integrates data and metadata across all omics layers of DNA, RNA, AA. More importantly, it allows users to ingest their own research data with a single click to create a single source of truth that combines public and proprietary datasets.
From this data universe, researchers can custom-build their own data model by picking and choosing the datasets that are best aligned with their research goals.
Download our ebook on HYFTs™: Connecting the Dots and Databases in Life Sciences
The HYFTs™ workflow: Using HYFTs™, we can instantly index nearly one million SARS-CoV-2 sequences into one multidimensional information model that contains data as well as metadata related to different geographies, epitopes, clinical outcomes etc. Moreover, any new incoming data will be automatically indexed and added to the current data model.
Not only does this eliminate the need to rebuild the model all over again but it also makes it easier to preserve and track systemic trends, like the evolution of different mutations in different geographies, for example.
One of the key objectives of this exercise was to identify any HYFT™- level interactions between the coronavirus and the microbiome. Based on the individual HYFT™ patterns of the coronavirus and the microbiome, we were able to isolate elements at the intersection between coronavirus and mycobacterium that were responsible for the development of TVC of tuberculosis.
Automated text mining with BioStrand Lensai Platform
HYFTs™ simplify and streamline the unification of all multiomics data and metadata into one integrated model. But there is still the imperative to integrate free text experimental research knowledge distributed across thousands of volumes of scientific literature that may provide new perspectives on causal interactions and correlations that could potentially augment sequence-based analyses.
Currently, most biomedical NLP solutions use the top-down approach to extract information related to a specific query. In contrast, BioStrand Lensai Platform is based on an unbiased bottom-up approach that reveals all novel concepts and relationships in the literature that are relevant to the research. This approach is also domain agnostic and does not need to be trained as it is capable of identifying all meaningful relationships without requiring predefined domain knowledge.
This is important as it enables better control over word boundaries. By combining this approach with dictionary matching and deep learning models for annotation, it is possible to create a massive index of concept-relation-concept patterns to understand how things are related to each other.
The BioStrand workflow:
BioStrand Lensai Platform uses smart indexing relation detection to identify the interaction between words, concepts and context. Smart indexing captures complex concept – relation – concept triplet structures to find direct relationships. For instance, the smart indexed version of the sentence “Two patients are suffering from congestive heart failure” would be Concept: “Two patients” — Relation: “are suffering from” — Concept: “congestive heart failure”.
One of the key differentiators of the BioStrand technology is its extensive semantic knowledge graph that links multiple concepts on multiple levels based on meaningful relationships. Since we are talking about enhancing sequence-based multiomics analysis with unstructured textual data, let’s go back to the previous SARS-CoV-2 workflow example. The HYFTs™ framework has already established an interaction between COVID-19 severity and mycobacterium tuberculosis.
With the BioStrand Lensai Platform, researchers can further explore the dynamics of the interaction based on preexisting knowledge recorded in scientific literature available in our pre-indexed database of around 30 million PubMed abstracts. And based on that we can identify that there are relations between Mycobacterium tuberculosis and Corona, not only we have found the relationship at the text level, as you can see here, but also at the sequence level. And text mining does indeed uncover more information about the relationship between COVID-19 severity and mycobacterium tuberculosis exposure history.
So, BioStrand’s unique sequence + text approach to integrated multiomics analysis combines the power of the HYFTs™ framework to index, aggregate and analyse all omics-related data. Researchers can then build on the insights revealed in this process with knowledge extracted using the Lensai Platform pipeline to gain new insights.
The Lensai Platform workflow for unified data analytics
The Lensai Platform features a unique bottom-up approach to bring meaning to data at the sequence and text level. The universal biological HYFT™data framework is central to the uniqueness of the platform.
A typical workflow on the BioStrand platform could be initiated based on any type of sequence. Researchers have immediate access to one billion analysis-ready data sets, including metadata, integrated from the most popular public repositories. The HYFT™ framework also enables them to seamlessly index and integrate proprietary data and metadata.
From here, the workflow could branch out in a number of different directions, such as identifying sequence relationships, retrieving epitope-paratope twins, etc. Once all relevant associations have been extracted and annotated, they can then be ranked as “Top Concepts” based on the dimensions that are most relevant to research.
These “Top Concepts” can then be fed into our platform pipeline to extract associations between sequence-based concepts and text-based information from scientific literature, EHRs, etc.
With end-to-end multi-level data integration in place, it then makes sense to apply advanced AI/ML techniques.
Intelligent multiomics starts with efficient data integration
The evolution of genomics from its present process, function, regulation or domain-specific focus to a systems biology will require new technological innovations that enable integrated analysis across multiomics, non-omics and textual data. Currently, most approaches involve the discrete analysis of different modalities with the results then being combined to build an integrated view.
Even if each of these discrete approaches were to be enhanced with advanced AI/ML, the results would still not allow for holistic biological interp[retation.
More importantly, though, the discrete approach can only be a stop-gap workaround for the critical upstream bottleneck that is omics data fragmentation. Simple and efficient data integration is the first step towards truly intelligent multiomics research.
With our platform, researchers now have access to powerful multimodal data integration tools like HYFT™ that can seamlessly unify fragmented multiomics, non-omics and unstructured data at scale.
Subscribe to our Blog and get new articles right after publication into your inbox.