Accelerating pharma R&D and innovation through technology partnerships
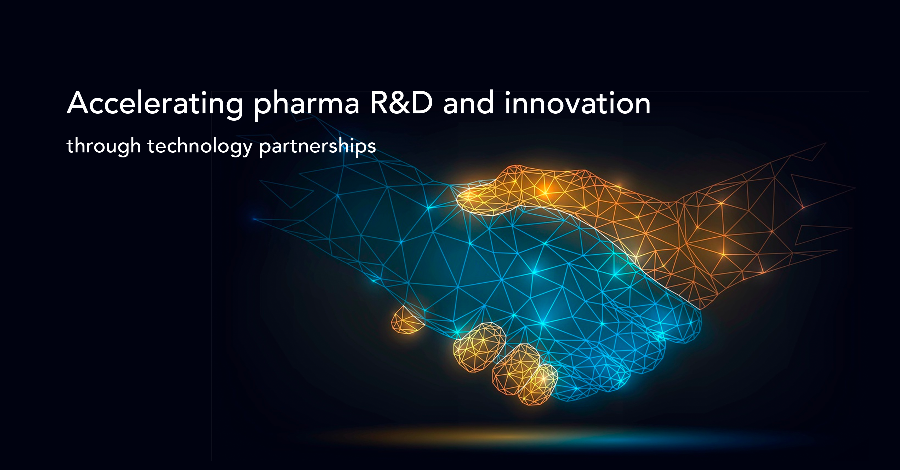
Biopharmaceutical companies are increasingly turning to alliances & partnerships to drive external innovation. Having raised over $80 billion in follow-on financing, venture funding, and initial public offerings (IPOs) between January and November 2021, the focus in 2022 is expected to be on the more sustainable allocation of capital by leveraging the potential of alliances and strategic partnerships to access new talent and innovation.
The race to market for COVID-19 vaccines has only accentuated the value of alliances as companies with core vaccine capabilities turned to external partnerships to leverage the value of emergent mRNA technology. And with alliances historically delivering higher return on investment (ROI), major biopharmaceutical companies have been deploying more capital toward alliances and strategic partnerships since 2020.
Pharma-startup partnerships represent the fastest-moving model for externalizing innovation to accelerate R&D productivity and drive portfolio growth. Within this broader trend, the AI-enabled drug discovery and development space continues to attract a lot of Big Pharma interest, spanning investments, acquisitions, and partnerships. AI is currently the top investment priority among Big Pharma players.
Biopharma majors, like Pfizer, Takeda, and AstraZeneca, have unsurprisingly also been leading the way in terms of AI start-up deals. In addition, these industry players are focusing on forging partnerships in the AI space to improve R&D activities. Just in the first quarter of 2022, leading industry players including Pfizer, Sanofi, GlaxoSmithKline, and Bristol-Myers Squibb, have announced multi-billion-dollar strategic partnerships with AI vendors.
However, the pharmaceutical sector has traditionally preferred to keep R&D and innovation in-house. Managing these strategic partnerships, therefore, introduces some new challenges that go beyond relatively simpler Build versus Buy decisions involving informatics solutions.
Managing strategic AI partnerships
According to research data from Accenture, the success rate of pharma-tech partnerships, assessed across a total of 149 partnerships between companies of all sizes, is around 60%. For early-stage partnerships, there are additional risks that can impact the success rate. The Accenture report distilled the four most common pitfalls that can impact every pharma-tech partnership.
SOURCE: Accenture
- Failing to prepare internally: According to executives of life science companies, defining partnership strategy and partner management functions are a key challenge in creating successful technology alliances.
It is important to start by defining the appropriate partnership structure and governance for the alliance, with mutually agreed partnership objectives, a dedicated team with the right technical knowledge and resources, and clearly defined partnership management functions.
- Engaging with the wrong partner: Despite the most stringent due diligence around technological relevance and strategic alignment, tech partnerships can fail because of organizational and cultural differences.
Sometimes the distinctive and complementary characteristics of each partner that make collaboration attractive can themselves create a “paradox of asymmetry” that makes working together difficult. Most corporations may be well equipped to deal with the two main phases of collaboration between large companies and startups: the Design phase, where the businesses meet and decide to engage, and the Process phase, where the interactions and collaborations kick off. New research shows that a preceding Upstream phase, to define and create conditions conducive to the Design and Process phases, can be decisive in the success of startup partnerships.
- Undefined partnership roadmap: Technological partnerships can be structured in a myriad of ways. For instance, the financial structure could be based on revenue sharing, milestone-based payments, etc. It is necessary to clearly define each engagement structure in terms of its operations, organizational, financial, legal, and IP implications. Formalize the roles, responsibilities, and accountabilities expected of each party. Establish short to medium-term goals, metrics, key milestones, and stage gates that build towards long-term partnership outcomes. Continuously reassess and fine-tune based on milestones and key performance indicators (KPIs).
- Poor execution: Effective long-term partnerships are based on executional excellence. Successful partnerships require a dedicated leader accountable for the execution and results. This role is essential for providing daily oversight of operational issues, addressing inter-organizational bottlenecks, and enforcing accountability on both sides. There also should be partnership meetings involving senior leadership to discuss how to accelerate progress or how to change tactics in the face of challenges or changing market conditions.
Building successful technology partnerships offers a fast, efficient, and cost-effective model for pharma and life sciences companies to develop new capabilities, accelerate R&D processes, and drive innovation. However, the scale and complexity of these partnerships, and the challenges of managing partnership networks, are only bound to increase over time.
Building end-to-end AI partnerships
In the race to become pharma AI leaders, many companies are looking at end-to-end AI coverage spanning biology (target discovery and disease modeling), chemistry (virtual screening, retrosynthesis, and small molecule generation), and clinical development (patient stratification, clinical trial design and prediction of trial outcomes).
This is where AI platforms like our Lensai Platform can play a key role in enabling value realization at scale. AI-native platforms based on multi-dimensional information models can seamlessly scale pharma R&D by automating data aggregation across different biological layers, multiple domains, and internal and external data repositories. Given the diverse nature of AI-driven platforms and services, pharma companies have the flexibility to choose partnerships that address strategic gaps in their R&D value chain. This includes custom data science services, drug candidate or target discovery as a service, AI-powered CROs, and platforms specializing in low-data targets. The focus has to be on enabling end-to-end AI coverage in pharma R&D, through a combination of partnerships and in-house investments in order to increase the productivity and efficiency of R&D processes while cutting the cost and the time to value.
Subscribe to our Blog and get new articles right after publication into your inbox.