Our most widely read blogs from 2022
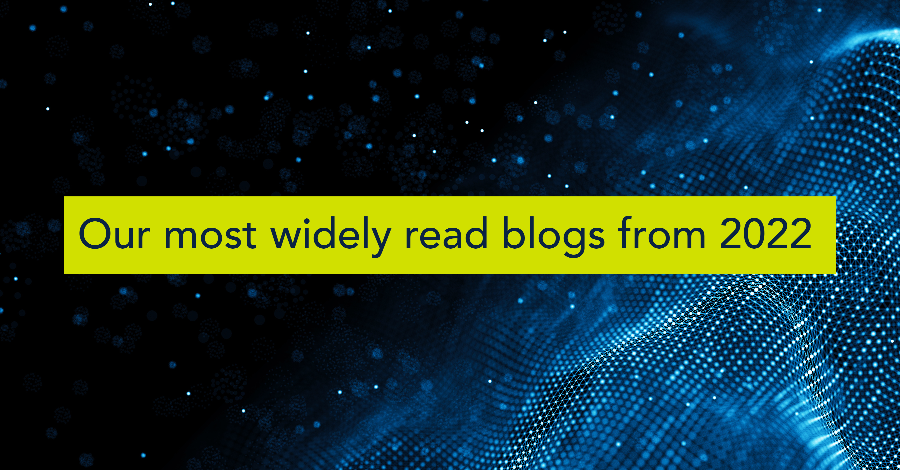
It’s been another momentous year for the global life sciences industry. Ever since the pandemic turned the world’s attention to the life sciences, the industry has collaboratively, and competitively, come together to successfully address one of the most critical threats of the times. And the momentum and motivation generated by that collective effort continue to drive the industry towards even more ambitious transformation and innovation goals.
Since we launched this blog two years ago, our effort has constantly been on documenting and elucidating some of the most groundbreaking technologies and ideas in contemporary life sciences R&D. So as a year-end sign-off, we have rounded up the most read articles this year.
Challenges In Multi-Omics Data Integration
Integrated multi-omics data analysis has emerged as an increasingly sophisticated, continuously evolving foundational process of big data-driven biological research. And yet, there is still nothing close to a universal framework that can be broadly applied to different types of biological data. Organizing biological big data for integrated analysis requires contending with data silos, missing values and precision variations across omics modalities, a variety of data distributions and types, etc. Early approaches to multi-omics data integration combined the independent analysis of different data modalities to create a sort of quasi-integrated view of biological behavior. Even today, at-scale integration of multi-omics data requires a catalog of data scaling, normalization, and transformation strategies to address the unique characteristics of each individual dataset. Though modern algorithmic meta-analysis approaches have helped mitigate integration challenges to a certain extent, there are still no gold standard models that can be applied universally across biological data. With the BioStrand HYFT™ data integration framework, it is now possible to instantly normalize and integrate all research-relevant biological data, including sequence, text, omics, and non-omics data.
Explained: A brief look into AlphaFold 2
Proteins are mostly characterized by their amino acid sequences. However, their function is defined by the complex 3-dimensional structures that these sequences fold into. Proteins with similar sequences tend to adopt similar shapes. However, proteins with similar structures can still have vastly different sequences. And while it is relatively easy to obtain protein sequences at scale, protein structure prediction has been a 50-year-old problem in search of a solution. That solution arrived in 2021, courtesy of Deepmind’s AlphaFold2, an end-to-end solution to predict protein structures based on input sequences. Using AlphaFold2 is rather straightforward, though it does require High Performance Computing resources. AlphaFold2 seems to have triggered a deluge of new models, tools, and databases that can predict protein structure with resolution.
Closing the Gap of Text and the Biosphere with LENSai™
All omics data is essentially unstructured, often in completely unique ways. Raw reads are unstructured until they are mapped to known references. Similarly, secondary research data is unstructured given the wide variance in their underlying technology, modality, process, etc. And then there is textual data, from scientific literature, EHRs, and other text-based information sources, which is, by definition, unstructured data. The key challenge in life sciences research today is to integrate all these different types of dissimilarly unstructured data for unified analysis. At BioStrand, we have addressed this challenge with two proprietary, next-generation innovations that bring structure to all biological data. Our LENSai™ Integrated Intelligence Platform uses the HYFT® universal framework to organize the entire biosphere as a multidimensional network of 660 million multi-omics data containing information about sequence, syntax, and protein structure. We then created the LENSai™ NLP pipeline to link knowledge and insights from text-based information sources to the sequence data. Life sciences researchers now have analysis-ready access to omics and non-omics data in one integrated end-to-end solution.
AI in early phase drug development
Early drug discovery and preclinical development is a complex process that is critical to the success of new drug candidates. They generate toxicology data relevant to the regulatory process, pharmacokinetic and pharmacodynamic data pertinent to dosage and trial design, and chemistry and control information crucial for clinical manufacturing. Even a quantum improvement at this phase can trigger a cascading transformation across the entire drug development value chain. Such a transformation is exigent as currently just 10 out of 10,000 preclinical candidates even make it to clinical trials. AI/ML technologies are driving this transformation and helping bridge the vast translational gap between preclinical discoveries and new therapeutics. They are being effectively deployed across the entire early drug discovery process flow, including target identification, lead identification, lead optimization, and candidate selection, and to a variety of discovery contexts and biological targets.
So, that’s it from us for 2022. Thank you for joining us and we hope to have been able to contribute some fresh perspectives and insights to your areas and topics of interest.
Subscribe to our Blog and get new articles right after publication into your inbox.