Multidimensional scalability in multi-omics research
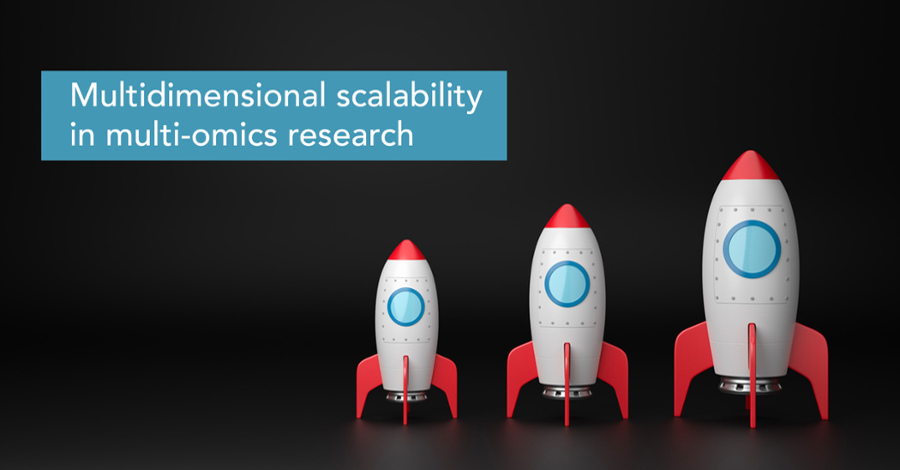
In 2018, the Joint Genome Institute (JGI) achieved a sequencing output of 200 trillion bases, a seven-fold increase over its output during the Human Genome Project. In 2020, that figure had jumped to 290 trillion bases with the institute delivering over 5 million bases/constructs delivered to researchers worldwide.
The JGI is by no measure the only prolific global provider of genomic data for research. UK Biobank, a research resource with access to in-depth genetic and health information from half a million participants, has registered a 4-fold increase in access applications and approved its 20,000th researcher earlier this year.
Today, there are numerous global sequencing projects that exemplify the importance of data sharing in genome science. The question that remains, however, is if genomic research can follow through and convert these huge volumes of unique data into novel, actionable biological insights.
Is genomics research scalable?
This is a question that is understandably triggered by comparative estimations that data volumes in genomics are getting bigger than that of astronomy, social media and video sharing combined, or that they are growing faster than the computational power forecasts of Moore’s Law.
Scalability, in one form or the other, has been a persistent challenge since the early days of bioinformatics. However, the data challenges in the post-genomic big data era are significantly more multi-dimensional. So, it is not only important to filter these challenges through some of the key characteristics of big data but the distinctive dynamics of genomics.
Key metrics of scalability in bioinformatics
Scalability, across data volume
Over the years, there has been a steady evolution of parallel and distributed computing technologies, clusters, grids, GPUs etc., in the field of bioinformatics to address volume-related scalability issues. Therefore, cloud technologies, together with their modern big data analysis frameworks, represent a natural evolutionary inflexion point for bioinformatics in the big data era.
With the cloud, researchers and institutions finally have access to a transparent, on-demand, pay-as-you-go model that can seamlessly scale computational resources, horizontally and vertically, to accommodate a range of analytical workloads.
However, many conventional bioinformatics tools based on single system and single node architectures are not designed to leverage the potential of the cloud model. Therefore, any attempts at piecemeal integrations of popular tools with cloud-based resources may only end up creating as many challenges as they resolve. The ideal approach here may be for a hybrid model that enables the secure integration of custom bioinformatics tools and workflows with cloud resources.
Scalability, to manage data velocity
The exponential rate of growth of genomics data has to be matched by a proportional increase in processing productivity and insight generation. This is absolutely essential just to narrow the ever-widening gap between data and insight in genomics.
Expecting conventional manually-intensive workflows, processes and models from an era of data scarcity to collect, clean, process, and analyse high-throughput data at scale will simply create bottlenecks along the bioinformatics value chain.
Advanced techniques like machine learning can enable data management at scale and are already used in biological research for a range of applications including gene prediction, protein structure prediction, and medical image analysis.
However, techniques like machine learning require highly specialized technology and data science skillsets that are hard to find. Moreover, this approach, though more productive than the conventional, only transposes manual intervention from one form to another. The next logical step, therefore, is to deploy techniques like AutoML to automate the selection, composition and parameterisation of machine learning models.
Approaches like this can significantly accelerate omics research and enhance analytical accuracy compared to manual managed processes.
Scalability, to integrate data variety
Heterogeneity is an innate feature of bioinformatics research traversing a veritable variety of data types and sources such as electronic health records, medical imaging data, single-cell data, omics data (epigenomics, transcriptomics, proteomics, metabolomics), omics subdisciplines (chemogenomics, metagenomics, pharmacogenomics, toxicogenomics), social networks and wearables.
The availability of these large-scale, multidimensional, and heterogeneous datasets has expanded the scope of genomics research from single-layer analysis to a holistic multi-dimensional interpretation of biological data. The emphasis in integrated multi-omics is on integrating diverse types of omics data from different layers of biological regulation to develop rich, multi-scale characterisations of biological systems.
However, integrated multi-omics cannot be achieved by a collage of tools for different -omes. It requires solutions that can simultaneously scale across diverse datasets and centralise data processing, analysis, and interpretation within a unified inference framework.
Apart from these three Vs of big data, there are two other dimensions of issues that are of particular relevance to bioinformatics.
Scalability, to unify workflows
A typical data analysis workflow/pipeline in bioinformatics consists of a sequence of 10-15 third-party tools, each with its own libraries, standards, and protocols. Take RNA sequencing, for instance, a complex workflow comprising a series of distinct processes. Existing software tools are so specialised that they only perform one step, such as the alignment of reads to a reference genome, of a larger workflow.
In such a situation, optimising scalability is focused on each individual process that constitutes a workflow. This means that the overall scalability of the workflow will be limited by the step with the least scalability. For maximum research productivity and efficiency, bioinformatics workflows have to be transitioned from this tiered approach to unified integrated workflow models that facilitate systemic optimisations to improve accuracy and speed of analysis.
Scalability, to accommodate different skill profiles
Genomic data analysis is increasingly becoming an exclusive for computationally skilled bioinformaticians and data scientists. It is not uncommon to come upon articles that reference how omics analysis takes computational skills beyond the standard repertoire of a molecular biologist or that it’s user-unfriendly for the typical lab scientist.
Given the data deluge in genomics, multi-omics analysis solutions need to focus on accessibility and usability rather than on specialised skills. This is a dimension of scalability that can have a truly transformative impact on genomics research. The focus should be on providing augmented analytics solutions that automate all aspects of data science, machine learning, and AI model development, management and deployment to democratise multi-omics analysis for everyone.
The BioStrand approach to scalability
The BioStrand platform is designed to address all dimensions of scalability.
Our platform’s container-based architecture enables seamless autoscaling to handle data volumes of over 200 petabytes with zero on-ramping issues.
We addressed data heterogeneity by developing an HYFT™ IP that allows us to integrate all types of data and make them instantly computable. We have compiled, pre-computed and pre-indexed multimodal data from across 11 public databases into one pan-genomic knowledge base to give researchers instant access to all publicly available multi-omics datasets. HYFTs™ allow citizen data scientists to normalise and integrate their own datasets with a single click, be it unstructured data such as patient record data, scientific literature, clinical trial data, chemical data computable, or structured data such as ICD codes, lab tests, and more.
With all research-relevant data in one place, the BioStrand platform offers one integrated workflow for analysis. However, it also allows unlimited customisation opportunities for users to plot and navigate their own research pathways through the data. Users also have access to a range of intelligent, convenient, and scalable tools to simplify and accelerate the discovery and synthesis of knowledge.
Finally, with the BioStrand SaaS delivery model, users have access to pay-as-you-go pricing with scale-as-you-grow pricing tiers.
Contact us to learn more about how multidimensional scalability enhances the bioinformatics experience.
Subscribe to our Blog and get new articles right after publication into your inbox.