Making all omics data computable
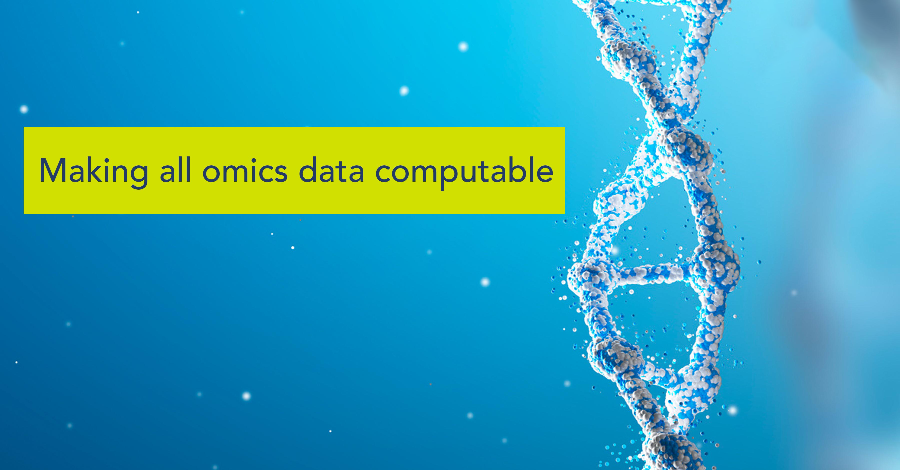
At the beginning of the last decade, the Harvard Business Review described data science as the sexiest job of the 21st century. But somewhere in the middle of the same period, reality kicked in, with one study of data scientists revealing that it was mostly janitorial data work. Data scientists were spending the majority of their time cleaning, organizing and preparing data – a tedious chore that quite understandably was rated the least enjoyable part of their work.
That past is now prologue for omic data analysis and research in this decade.
On the one hand, continuous advancements in the scale, accuracy and diversity of publicly available multi-species genome sequence data mean that there’s never been a better time for those with bioinformatic and multi-omics research aspirations. On the other, many of those aspirations will still be tempered with the reality that genomics research and analysis is predominantly data preparation/organization rather than insight and breakthrough.
State of omics data
Today, there is a wealth of publicly available genetic sequence and omics data distributed across several constantly expanding databases. However, information silos and data fragmentation remain an overarching feature of all these data sources.
For researchers, the primary challenge is to locate the datasets that are most relevant to their area of study. More often than not, researchers have to manoeuvre through multiple complicated workflows to obtain data from one data source, reformat the data and submit to another data source for analysis, parse the analysed result, combine the result with data obtained from a third data source, and so on and so forth.
Given the multiplicity of data sources, the diversity in data formats, the lack of integration between silos of domain-specific information, and the overabundance of specialised and proprietary bioinformatics tools, data preparation, integration and normalization inevitably becomes a tedious and time-consuming process. As a consequence, substantial volumes of knowledge still remain untapped.
At BioStrand, we saw that a better way to genomics research has to start with a more efficient and innovative approach to data integration and normalisation. So, we decided to design an entirely new technique to efficiently handle the large data sets in genomic science.
The BioStrand approach to omics data science
Our unique approach to omics data science combines the company’s insights into DNA, RNA, and proteins with a pipelined approach to mass scalable analytics in order to accelerate the time-to-value of genomics research projects.
At the core of our innovative new approach to omic data science is a biological discovery called HYFT™ patterns. HYFTs™ are the output of a radically new technique for indexing cellular blueprints and building blocks which significantly enhances the efficiency and scalability of analysing large genomic datasets while opening up new opportunities to explore and understand genome functionality.
HYFT™ patterns are signature sequences in DNA, RNA and AA that serve as biological fingerprints and contain a multitude of information layers covering function, structure, position, etc. At BioStrand, we have built a proprietary knowledge database using our 660 million HYFT™ patterns to index nearly 350 million sequences available across 11 public databases. In essence, we created a knowledge base that can be leveraged for a range of applications and across species. In addition, researchers can easily add self-owned databases with a single click, and then combine them with publicly available datasets to significantly expand the scope of their research.
We then augmented this knowledge base with indexing and exact matching functionality, à la Google, to make searching the network for coding and non-coding sequences simple, accurate, comprehensive and fast. Researchers can sift through the knowledge base, using sequence or text, and, in a matter of seconds, our 660 million HYFT™ patterns help retrieve all relevant information about alignments, similarities, and differences in sequences. With the BioStrand approach, information on millions of organisms is instantly searchable without having to navigate through multiple discrete data sources or switch between disparate data tools.
Our indexing solution combined with the sequence features incorporated in HYFTs™ opens up brand new avenues for data analysis at the genomic and proteomic level that were technologically impractical thus far.
Data normalization was a strategic priority quite early in the development process given the diversity of formats and types in genomic data. Together with our technology partners Data Minded, we created a path-breaking new universal schema to store genomic data and metadata for use across multiple platforms, techniques, and tools.
With these two functionalities in place, we then set out to build a robust and scalable platform to manage the normalization, storage, analysis, cross-comparison, and presentation of petabytes of genomics data. The platform utilizes highly parallelized indexing, and multi-format datasets with pre-indexed sequences, to make data analysis quick, efficient and accurate. As a fully functional self-service SaaS offering, and with researchers uploading their own data, the BioStrand platform’s container-based architecture is designed to auto scale seamlessly to handle over 200 petabytes of data with zero on-ramping issues.
And finally, we built out the BioStrand SaaS platform with powerful, state-of-the-art AI tools that mitigate data complexity and help researchers intuitively synthesise knowledge out of a multitude of information sources. With this addition, all genomics data is now easily and seamlessly integrable and computable – be it unstructured data, such as patient record data, scientific literature, clinical trial data, chemical data, or structured data, such as ICD codes, lab tests, and more.
Making omics data science sexy again
There are three reasons why an 80-percent-data-prep-and-20-percent-analysis approach to genomic data is not sustainable.
- First, it is simply an inefficient data strategy – especially so in the age of Big Data – that leaves a lot of value on the table.
- Second, it compounds the already dire situation of the ever-widening gap between data generation and data analysis in genomics.
- And third, it inexcusably deploys scarce and valuable human data science capital towards mundane monotonous activities.
The volume, complexity, diversity and siloed nature of omics has historically been seen as a reality that has to be accommodated rather than addressed. But as we have demonstrated, it is possible, with a little bit of technology, ingenuity and innovation, to make omics data research less about the data and more about the research. At BioStrand, we focus on making all omics data computable out-of-the-box so that you can focus on research, analysis, insights and breakthroughs.
Subscribe to our Blog and get new articles right after publication into your inbox.