Biomedical knowledge graphs and the power of ontology
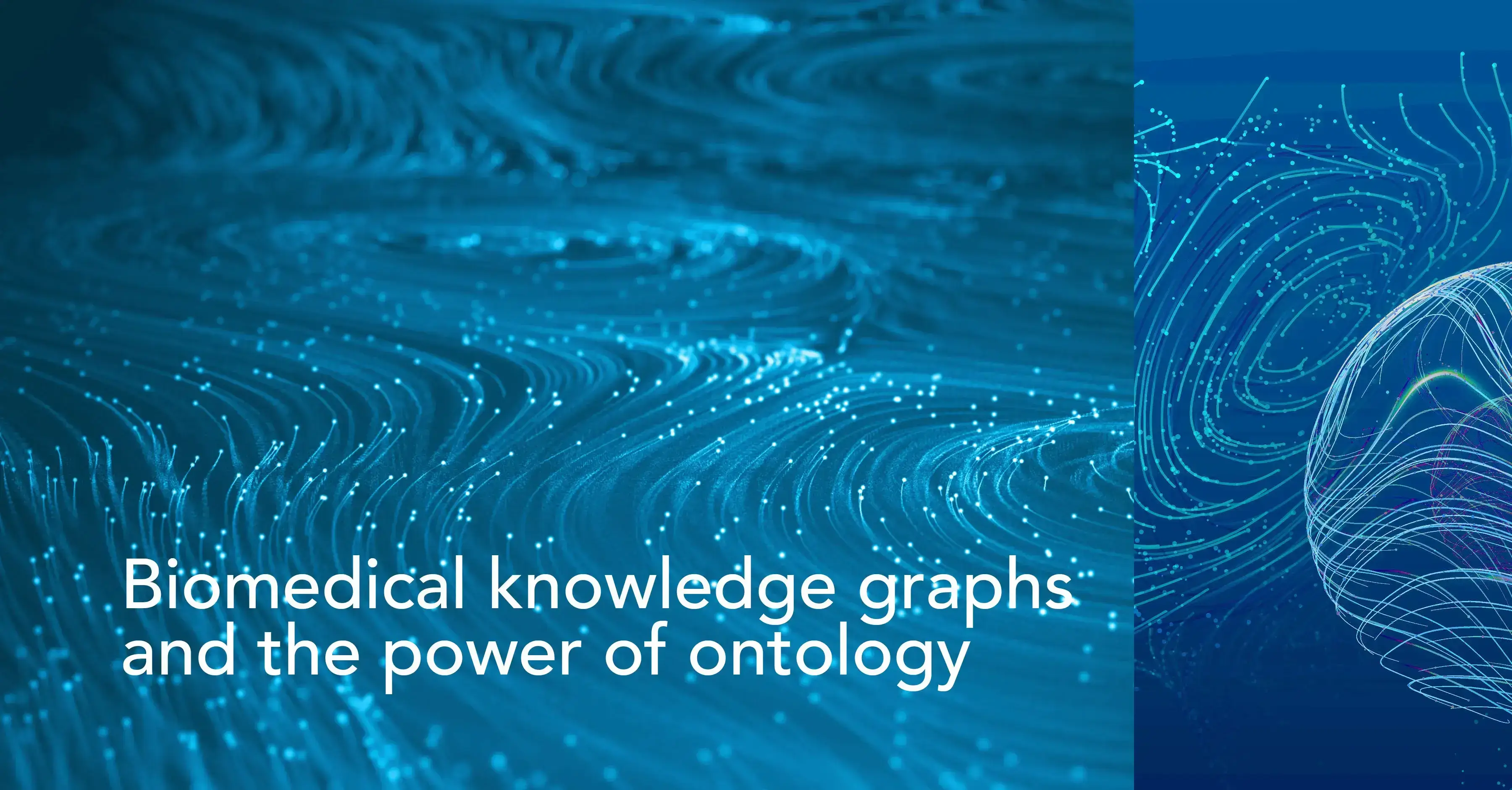
Audio version
Knowledge graphs play a crucial role in the organization, integration, and interpretation of vast volumes of heterogeneous life sciences data. They are key to the effective integration of disparate data sources. They help map the semantic or functional relationships between a million data points. They enable information from diverse datasets to be mapped to a common ontology to create a unified, comprehensive, and interconnected view of complex biological data that enables a more contextual approach to exploration and interpretation.
Though ontologies and knowledge graphs are concepts related to the contextual organization and representation of knowledge, their approach and purpose can vary. So here’s a closer look at these concepts, their similarities, individual strengths, and synergies.
What is an ontology?
An ontology is a “formal, explicit specification of a shared conceptualization” that helps define, capture, and standardize information within a particular knowledge domain. The key three critical requirements of an ontology can be further codified as follows:
‘Shared conceptualization’ emphasizes the importance of a consensual definition (shared) of domain concepts and their interrelationships (conceptualization) among users of a specific knowledge domain.
The term ‘explicit’ requires the unambiguous characterization and representation of domain concepts to create a common understanding.
And finally, ‘formal’ refers to the capability of the specified conceptualization to be machine-interpretable and support algorithmic reasoning.
What is a knowledge graph?
A knowledge graph, aka a semantic network, is a graphical representation of the foundational entities in a domain connected by semantic, contextual relationships. A knowledge model uses formal semantics to interlink descriptions of different concepts, entities, relationships, etc. and enables efficient data processing by both people and machines. Knowledge graphs, therefore, are a type of graph database with an embedded semantic model that unifies all domain data into one knowledge base. Semantics, therefore, is an essential capability for any knowledge base to qualify as a knowledge graph.
Though an ontology is often used to define the formal semantics of a knowledge domain, the terms ‘semantic knowledge graph’ and ‘ontology’ refer to different aspects of organizing and representing knowledge.
What’s the difference between ontology and a semantic knowledge graph?
In broad terms, the key difference between a semantic knowledge graph and an ontology is that semantics focuses predominantly on the interpretation and understanding of data relationships within a knowledge graph, whereas an ontology is a formal definition of the vocabulary and structure unique to the knowledge domain.
Both ontologies and semantics play a distinct and critical role in defining the utility and performance of a knowledge graph.
An ontology provides the structured framework, formal definitions, and common vocabulary required to organize domain-specific knowledge in a way that creates a shared understanding. Semantics focuses on the meaning, context, interrelationships, and interpretation of different pieces of information in a given domain.
Ontologies provide a formal representation, using languages like RDF (Resource Description Framework), and OWL (Web Ontology Language) to standardize the annotation, organization, and expression of domain-specific knowledge. A semantic data layer is a more flexible approach to extracting implicit meaning and interrelationships between entities, often relying on a combination of semantic technologies and natural language processing (NLP) / large language models (LLMs) frameworks to contextually integrate and organize structured and unstructured data. Semantic layers are often built on top of an ontology to create a more enriched and context-aware representation of knowledge graph entities.
What are the key functions of ontology in knowledge graphs?
Ontologies are essential to structuring and enhancing the capabilities of knowledge graphs, thereby enabling several key functions related to the organization and interpretability of domain knowledge.
The standardized and formal representation provided by ontologies serves as a universal foundation for integrating, mapping and aligning data from heterogeneous sources into one unified view of knowledge.
Ontologies provide the structure, rules, and definitions that enable logical reasoning and inference and the deduction of new knowledge based on existing information.
By establishing a shared and standardized vocabulary, ontologies enhance semantic interoperability between different knowledge graphs, databases, and systems and create a comprehensive and meaningful understanding of a given domain. They also contribute to the semantic layer of knowledge graphs, enabling a richer and deeper understanding of data relationships that drive advanced analytics and decision-making.
Ontologies help formalize data validation rules, thereby ensuring consistency and enhancing data quality.
Ontologies enhance the search and discovery capabilities of knowledge graphs with a structured and semantically rich knowledge representation that enables more flexible and intelligent querying as well as more contextually relevant and accurate results.
The importance of ontologies in biomedical knowledge graphs
Knowledge graphs have emerged as a critical tool in addressing the challenges posed by rapidly expanding and increasingly dispersed volumes of heterogeneous, multimodal, and complex biomedical information. Biomedical ontologies are foundational to creating ontology-based biomedical knowledge graphs that are capable of structuring all existing biological knowledge as a panorama of semantic biomedical data. For example, Scalable Precision Medicine Open Knowledge Engine (SPOKE), a biomedical knowledge graph connecting millions of concepts across 41 biomedical databases, uses 11 different ontologies as a framework to semantically organize and connect data. This massive knowledge engine integrates a wide variety of information, such as proteins, pathways, molecular functions, biological processes, etc., and has been used for a range of biomedical applications, including drug repurposing, disease prediction, and interpretation of transcriptomic data.
Ontology-based knowledge graphs will also be key to the development of precision medicine given their capability to standardize and harmonize data resources across different organizational scales, including multi-omics data, molecular functions, intra- and inter-cellular pathways, phenotypes, therapeutics, environmental effects, etc., into one holistic network.
The use of ontologies for semantic enrichment of biomedical knowledge graphs will also help accelerate the FAIRification of biomedical data and enable researchers to use ontology-based queries to answer more complex questions with greater accuracy and precision.
However, there are still several challenges to the more widespread use of ontologies in biomedical research. Biomedical ontologies will play an increasingly strategic role in the representation and standardization of biomedical knowledge. However, given their rapid growth proliferation, the emphasis going forward will have to on the development of biomedical ontologies that adhere to mathematically precise shared standards and good practice design principles to ensure that they are more interoperable, exchangeable, and examinable.
Subscribe to our Blog and get new articles right after publication into your inbox.