Scaling up drug discovery: Exploring the LENSᵃⁱ approach
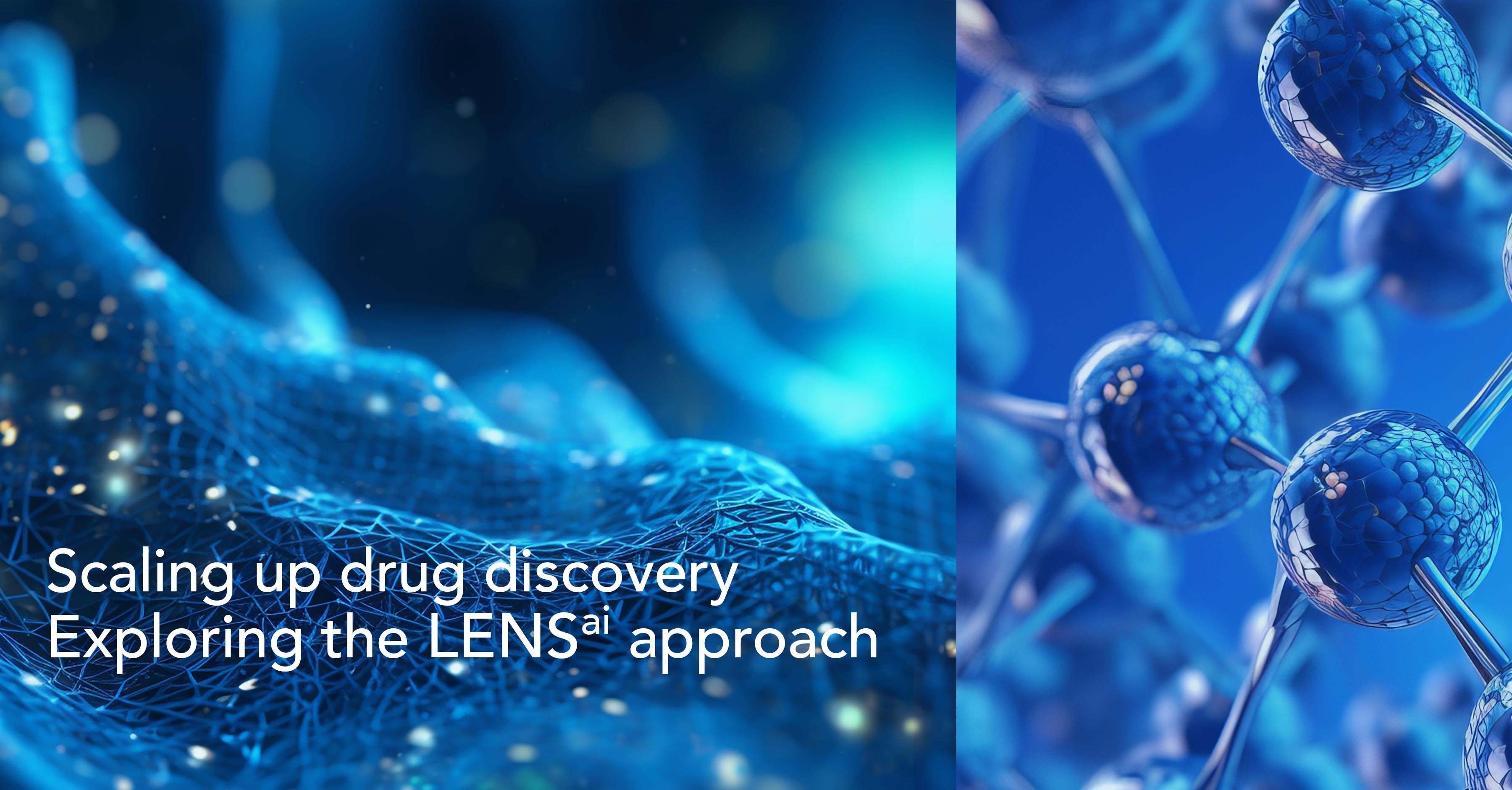
In the first blog in our Through LENSai series, we discussed the Information Integration Dilemma (IID) in the context of systems biology and how the LENSai Integrated Intelligence Platform helps crack that dilemma.
Today, we will look at the integration dilemma as it applies to drug design and development and provide a quick overview of the LENSai approach to addressing it.
For years, pharma R&D has been characterized by some rather staggering KPIs. The process of bringing a new drug to market took up to 15 years, cost approximately $2.5 billion, and entailed a failure rate of 90%. However, with the advent of AI and high-throughput biological Big Data, the stage’s now set for a new optimistic era of cost-effective, productive, and efficient drug development.
Data-driven AI-powered drug development comes with some pretty ambitious predictions. It could potentially create an additional $50 billion market over the next decade. It could enable R&D cost savings of as much as $400 million per new drug. And could accelerate drug development processes, sometimes by more than a factor of 1000, and slash time-to-market for new drugs.
It is unsurprising, therefore, that industry professionals are looking to AI and its partner Big Data to play a disruptive role in the pharmaceutical industry in 2023.
However, a November 2022 McKinsey survey of digital and analytics leaders in life sciences revealed that despite applied AI having permeated life sciences R&D, accessing and integrating data remained the top hurdle to at-scale deployment.
Addressing this integration dilemma will be the key first step for pharma R&D to fully exploit the potential of AI and big data.
The Information Integration Dilemma (IID) in drug development
Drug design and development encompasses a striking medley of data types, sources, and formats, with each presenting its own unique challenge to the data integration process.
To start with, there are the compound libraries that are at the core of traditional drug discovery. These datasets scale into the millions and are distributed across several proprietary, public and academic silos. Often it is necessary to combine data sources, either to tailor data sets to high-throughput screening requirements or to expand coverage of the chemical space. However, the diversity of these data sources, in terms of assay protocols, annotation conventions, etc., poses significant challenges to data curation and homogenization.
Then there is the challenge of integrating increasing volumes of multi-omics data that is critical to obtaining a comprehensive picture of particular disease states. However, different omics layers are characterized by different technologies and assays, resulting in a heterogeneity of datasets with different sources, modalities, formats, etc.
Linking multi-omics data with longitudinal phenotypic information from electronic health records (EHRs) under a unified analytics framework facilitates a more effective approach to the evaluation and validation of drug targets. However, since phenotypic information is embedded as unstructured data in free-text documents, this requires specialized biomedical
natural language processing (BioNLP) frameworks to extract relevant information.
Meanwhile, BioNLPs are growing as a distinct category to accelerate knowledge discovery by identifying novel biomedical relations from text-based sources including scientific literature, and clinical notes. At the same time, real-world data (RWD) is expanding beyond information from EHRs, medical claims, product/disease registries, and digital health technologies to non-health data sources, such as credit-card spending, geospatial data, etc. Integrating real-world data opens up new opportunities for optimizing the drug development pipeline and can help accelerate the approval of more effective and affordable treatments. Then there’s the huge potential in integrating rich multimodal imaging data for the anatomical, functional, and molecular information they can provide to accelerate drug discovery.
And all this still represents an indicative rather than an exhaustive inventory of potential data sources for drug development. To date, data integration frameworks and strategies have predominantly focused on addressing the challenges presented by specific data types or application areas. The LENSai Integrated Intelligence Platform exemplifies a unified approach to drug development, from the seamless integration of multimodal, multidimensional big data to AI-powered, scalable analysis, insight generation, and knowledge discovery.
LENSai and data-driven AI-powered drug development
The LENSai Integrated Intelligence Platform’s fully integrated biotherapeutic intelligence framework is designed around three core principles:
1. Create one comprehensive integrated view across sequence, structure, and textual information
The LENSai platform leverages the power of HYFTs, Universal FingerprintTM patterns found across the entire biosphere, to unify all datasets/ types into one framework. The platform seamlessly scales across different data silos and sources, diverse data formats and structures, and disparate schemas, standards, and semantics.
Apart from generating a unified data view, HYFTs also detect biologically and functionally relevant cross-data relationships. This relation-centric approach to data integration and visualization provides researchers with a systems view of biological complexity that facilitates holistic, accurate, and actionable insights based on contextual biological connections.
The LENSai approach to data integration connects the biosphere’s fundamental pillars of Sequence (DNA-RNA-Protein), Structure (Alpha Fold, ESM-2, Rosetta Fold, Cryo-EM, Crystallography), and Text (peer-reviewed literature, patents, clinical Trials) into one comprehensive, interconnected, multidimensional knowledge graph with over 25 billion sequence-structure-text relationships.
2. Enable explainable insights
The LENSai knowledge graph integrates heterogeneous data and facilitates a connected and contextual understanding of complex data relationships that is critical to accelerating end-to-end clinical discovery. Apart from enabling (sub)sequence-, structure-, and text-based insights, the platform also provides researchers with the tools to query the knowledge graph and explore the interconnected world of sequence-structure-text.
As AI becomes prevalent in the drug discovery pipeline, the emphasis is now on developing white-box models that enhance the transparency, interpretability, and explainability of pharma AI. Our platform’s white-box approach to AI ensures that all concepts and relations can be traced back to the source to assess the validity and reliability of information.
3. Facilitate deeper analysis with advanced AI
A rich suite of integrated AI tools allows researchers to perform in-depth subanalysis, for instance with subsets of data, test hypotheses, create predictions and further explore the biosphere for new insights and hidden relationships. The built-in graph-based AI clustering algorithms can be used to detect entities that share syntactical and structural ‘closeness’. For instance, the clustering approach can be used to significantly improve antibody characteristics and augment the quality and quantity of leads during high-throughput screening in early-stage discovery.
Actionable Data + Integrated Intelligence = Real-time, At-scale Analysis
The LENSai framework seamlessly blends instantly actionable data with integrated intelligence to empower researchers to analyze large-scale, multi-source, multi-dimensional data in real time. Our API-first platform facilitates the frictionless integration of distributed research teams and provides a consistent, unified user experience that promotes collaboration and productivity. So, if you’re looking for a quick, convenient, and comprehensive solution to the Information Integration Dilemma in drug discovery and development, please do get in touch.