Everyone’s talking about LLMs: Notes from BioTechX Europe 2023
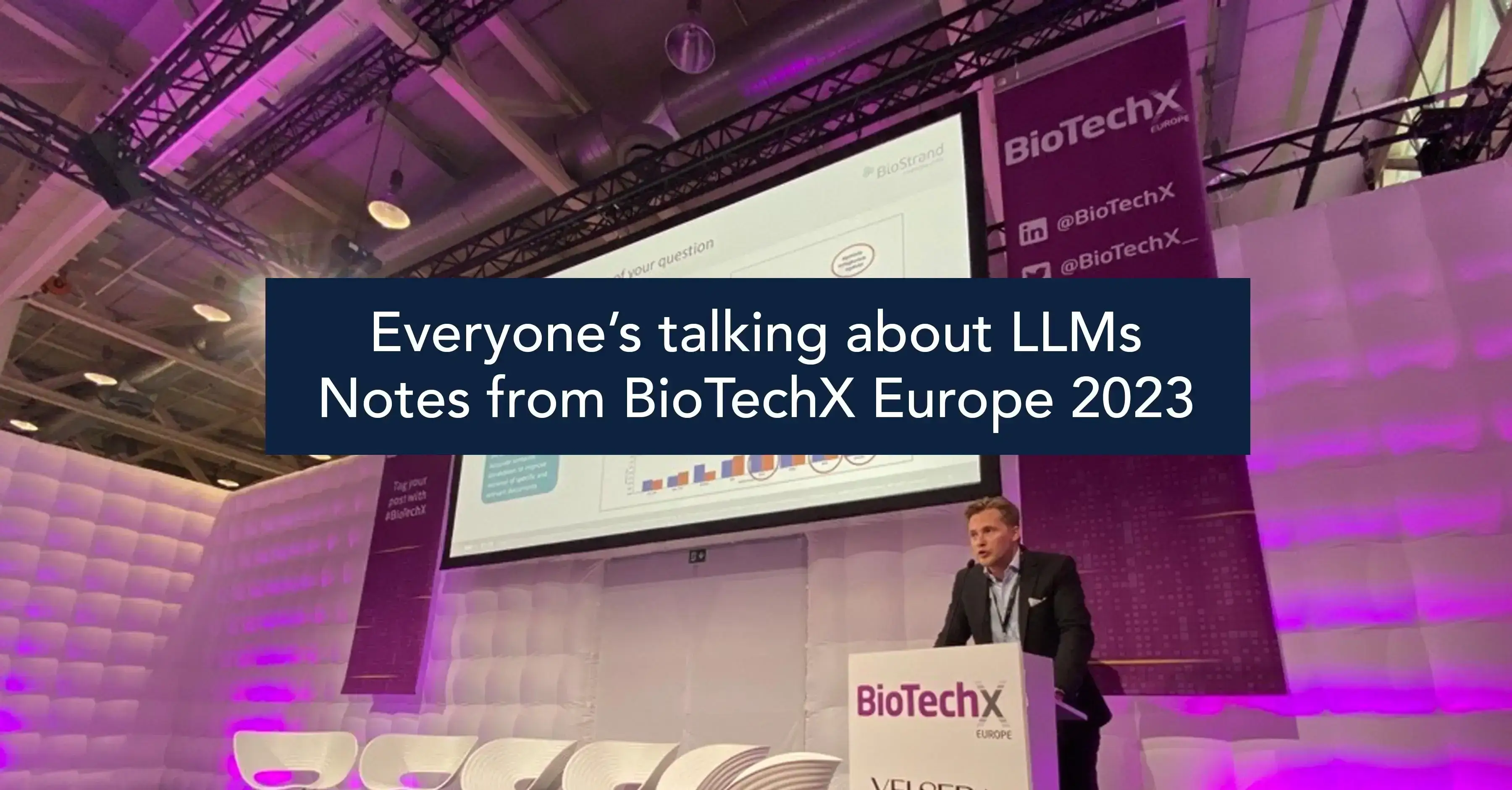
This October, we returned to BioTechX Europe 2023, Europe’s largest biotechnology congress, to present, network, and tune into the latest research and technology trends in the life science industry. It was another excellent opportunity to interact and exchange ideas with some of the best minds from the life scenes, bioinformatics, and technology sectors.
This has been a year when almost every conversation on artificial intelligence (AI) technologies has inevitably veered off into the transformative potential of large language models (LLMs), This is a trend that’s been reflected in the agenda at the conference, and understandably so. The pharma and life sciences industry has successfully leveraged AI to propel innovation and process enhancement and generative AI with LLMs represents a natural next step in that technology adoption arc. As a result, the conference showcased multiple industry perspectives on the value and challenges of integrating LLMs into the life sciences technology stack.
It was interesting to see the first real-world results emerge of what until recently was the promise of LLMs in biotechnological applications such as protein design and engineering. The focus is now transitioning to integrating these technologies into bioinformatics platforms, pipelines, and workflows that will enable every life sciences researcher to harness their potential. Some challenges, such as cataloging internal capabilities, still have to be addressed, but the critical first step towards more mainstream LLM adoption for these specific applications has been taken. Concurrently, data management strategies are evolving fairly rapidly with the emphasis now on standardizing practices for handling and testing data, managing outdated/redundant data, and building production-ready data frameworks.
In the broader context, LLMs are currently being used predominantly to streamline retrieving information from complex biomedical knowledge graphs. With knowledge graphs emerging as a significant trend in connecting and organizing vast volumes of heterogeneous biomedical information, LLM capabilities are a natural fit for enabling more efficient knowledge discovery.
However, a more strategic integration of biomedical LLMs and knowledge graphs opens up opportunities for synergistic, bidirectional data-based, and knowledge-based reasoning for more complex life sciences research applications.
In conclusion, our presentation at the BioTechX conference highlighted the strategic integration of various techniques and frameworks to overcome significant limitations associated with Large Language Models (LLMs) in the context of life sciences R&D, particularly in the domain of target validation. These limitations included the tendency for LLMs to hallucinate information, lack of interpretability, limited labeled biomedical data for training, the complexity of the biomedical vocabulary, knowledge cutoff constraints, and the need to safeguard proprietary data.
The BioStrand solution takes a semantics-first approach, unifying LLMs and knowledge graphs to enhance accuracy and specificity in knowledge retrieval and answer generation. This comprehensive approach not only addresses the challenges posed by LLMs but also ensures the extraction of context-specific results, ultimately advancing the capabilities of these models in the dynamic field of life sciences research.
Discover valuable insights from last year’s BioTechX in our notes here
Subscribe to our Blog and get new articles right after publication into your inbox.