Multi-omics data in biomarker discovery
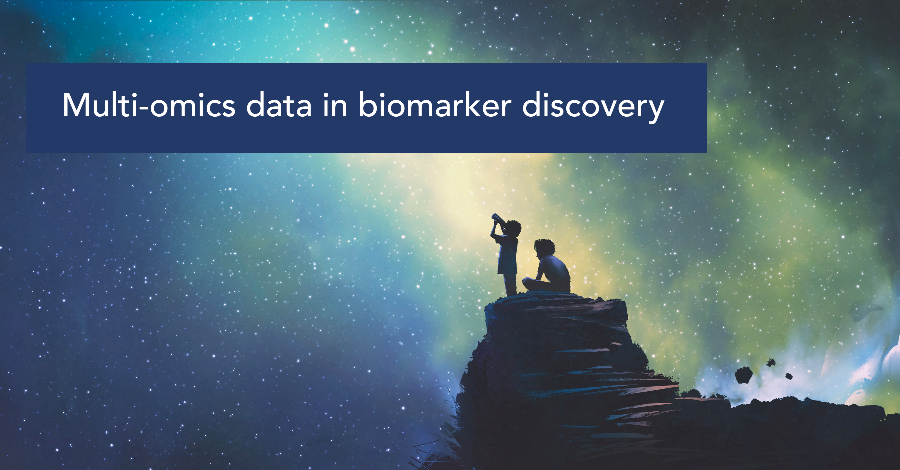
Current diagnostic alternatives for neurodegenerative diseases like Alzheimer’s, Parkinson’s, Down’s syndrome, dementia, and motor neuron disease, are either invasive lumbar punctures, expensive brain imaging scans, pen-and-paper cognitive tests, or a simple blood test in a primary care setting to check for NfL (neurofilament light chain) concentration.
Similarly, despite increasing evidence that exercise could delay or even prevent Alzheimer’s, there are currently no cost-effective or scalable procedures to validate or measure that correlation. However, research has now revealed that post-exercise increases in levels of plasma CTSB, a protease positively associated with learning and memory, could help evaluate how training influences cognitive change.
NfL and plasma CTSB are two prime examples of biomarkers, biological or characteristics found in body fluids and tissues that can be objectively measured and evaluated to differentiate between normal biological processes and pathogenic processes, or pharmacologic responses to therapeutic interventions.
The growing promise of biomarkers
In the seven decades since the term was first introduced, biomarkers have evolved from simple indicators of health and disease to transformative instruments in clinical care and precision medicine. Today, biomarkers have a wide variety of applications – diagnostic, prognostic, predictive, disease screening and detection, treatment response, risk stratification, etc., – across a broad range of therapeutic areas (cancer, cardiovascular, hepatic, renal, respiratory, neuroscience, gastrointestinal, etc.).
In keeping with the times, we now also have digital biomarkers – objective, quantifiable physiological and behavioural data collected and measured by digital devices.
Biomarkers are at the heart of ground-breaking medical research to, for instance, reveal the underlying mechanism in Acute Myelogenous Leukemia, improve prognosis of gastric cancer, establish a new prognostic gene profile for ovarian cancer, and provide novel etiological insights into obesity that facilitate patient stratification and precision prevention.
Biomarkers are also playing an increasingly critical role in the drug discovery, development and approval process. They enable a better understanding of the mechanism of action of a drug, help reduce the risk of failure and discovery costs, and allow for more precise patient stratification. Between 2015 and 2019, more than half of the drugs approved by EMA and FDA were supported by biomarker data during the development stage.
It is, therefore, hardly surprising that there is currently a lot of focus on biomarker discovery. However, this inherently complex process is only getting more complex, data-driven, and time-consuming – and that introduces some significant new challenges along the way.
The increasing complexity of biomarker discovery
Initially, a biomarker was a simple one-dimensional molecule whose presence, or absence, indicated a binary outcome. However, single biomarkers lack the sensitivity and specificity required for disease classification and outcome prediction in a clinical setting. Soon, biomarker discovery included panels – a set of biomarkers working together to enhance diagnostic or prognostic performance.
Then the field shifted again toward spatially resolved biomarkers that reflected the complexity of the underlying diseases. Rather than just provide aggregated information, these higher-order biomarkers incorporated the spatial data of cells expressing relevant molecular markers.
At the same time, biomarker developers are also integrating a whole range of omics data sets, such as genomics, proteomics, metabolomics, epigenetics, etc., in order to get a more holistic view that could augment our ability to understand diseases and identify novel drug targets.
The scope of biomarker discovery just keeps getting wider with the emergence of new data-gathering technologies like single-cell next-generation sequencing, liquid biopsy (blood sample) for circulating tumour DNA, microbiomics, radiomics, and with high-throughput technologies generating enormous volumes of data at a relatively low cost. The big challenge, therefore, will be in the integration and analysis of these huge volumes of multimodal data. Plus, biomarker data comes with some challenges of its own.
Biomarker data challenges
Data Scarcity: Despite their widespread currency, there are still very few biomarker databases available for developers. In addition, there could also be a lack of systemic omics studies and biological data relevant to biomarker research. For instance, metabolomics data, critical to biomarker research into radiation resistance in cancer therapy, is not part of large multi-omics initiatives such as The Cancer Genome Atlas. Therefore, it will require a network-centric approach to analytics that enable data enrichment and modelling with other available datasets.
Data Fragmentation: Biomarker data is typically distributed across subscription-based, commercial databases with no provision for cross-database interconnectivity, and a few open-access databases, each with its own therapeutic or molecular specialization. So, a truly multi-omics approach to analysis will depend entirely on the efficiency of data integration.
Lack of data standardization: Many sources do not follow FAIR database principles and practices. Moreover, different datasets are also generated using heterogeneous profiling technologies, pre-processed using diverse normalization procedures, and annotated in non-standard ways. Intelligent, automated normalization should be a priority.
How BioStrand can help
At BioStrand, we understand that a systems biology approach is crucial to the success of biomarker discovery. Our unique HYFT™ IP was born out of the acknowledgement that the only way to accelerate biological research was by unifying all biological data with a common computational language.
Access all biological data with HYFT™: On the BioStrand Platform, multi-omics data integration is as simple as logging in. Using HYFT™, we have already normalized, integrated, and indexed 450 million sequences available across 11 popular omics databases. That’s instant access to an extensive omics knowledge base with over a billion HYFTs™, with information about variation, mutation, structure, etc. What’s more, integrating your own biomarker research is just a click away. Add structured databases (ICD codes, lab tests, etc.) and unstructured datasets (patient record data, scientific literature, clinical trial data, chemical data, etc.) Our technology will seamlessly normalize and standardize all your data and make it computable to enable a truly integrative multi-omics approach to biomarker discovery.
Accurate annotation and analysis: The BioStrand genomic analysis tools provide unmatched accuracy in annotation and variation analysis, such as in the large-scale whole-genome data of patients with a specific disease. Use our platform’s advanced annotation capabilities to extract insights from genomic datasets and fill in the gaps in biomarker datasets.
Comprehensive data mining: Combine the power of our HYFT™ database with the graph-based data mining capabilities of our AI-powered platform to discover knowledge that can accelerate the development process.
From single biomarkers to systems biology
Biomarkers have evolved considerably since their days as simple single-molecule indicators of biological processes. Today, biomarker discovery is a sophisticated systems biology practice to unravel complex molecular interactions and expand the boundaries of clinical medicine and drug development.
As the practice gets more multifaceted, it will also require more advanced data integration, management, and analysis tools. The BioStrand Platform provides an integrated solution for normalization, integration, and analysis of high-volume high-dimensional data.
Subscribe to our Blog and get new articles right after publication into your inbox.