Intelligent multi-omics analysis: The power of AI/ML
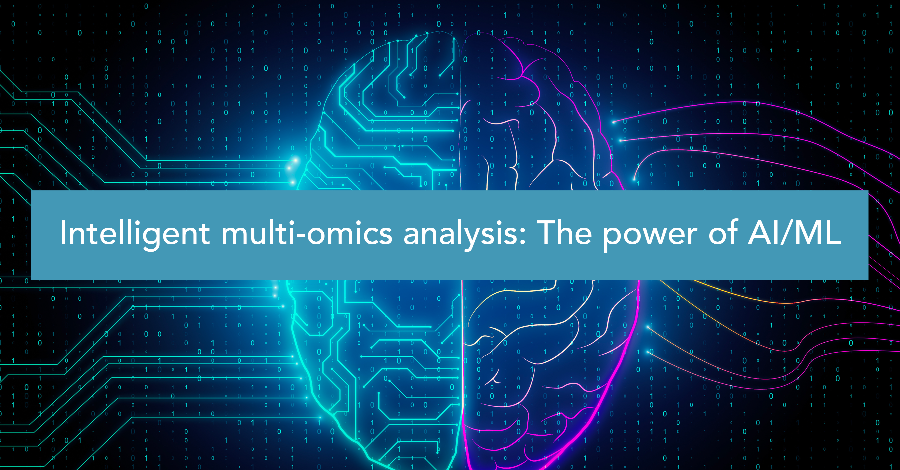
Audio version
In our previous blog on integrated multi-omics, we overviewed the need for this increase in scale, dimensionality and heterogeneity in genomic data to be matched by a shift from reductionist biology to a holistic systems biology approach to omics analysis. An integrated multi-dimensional and multivariate model for analysis is absolutely imperative for us to create a more comprehensive multi-scale characterization and understanding of biological systems.
However, there are several limitations, like scalability and reproducibility, for example, in applying conventional integration and interpretation techniques to multi-omics data. And conventional single-omics analyses are wholly ineffective in interpreting complex cellular mechanisms or identifying the underlying causes of multifaceted diseases.
As a result, multi-omics analyses increasingly rely on advanced computational methods and intelligent AI-powered technologies like ML, deep learning and NLP to optimize data management and transform multi-omics data into clinically actionable knowledge.
AI/ML in multi-omics analysis
Since then, ML and AI capabilities have evolved exponentially and have been applied consistently if not extensively for decision support in several healthcare scenarios including the management of several communicable and noncommunicable diseases.
Image Source: Biomolecules
A recent special issue of the journal Biomolecules focusing on the integrated analysis of omics data using AI, ML and Deep Learning (DL) listed three areas in medical research – medical image analysis, omics analysis, and natural language processing – where AI was currently being implemented.
Here, then, is an overview of the transformative potential of AI in these specific areas.
Medical image analysis
Intelligent technologies are a critical component of radiogenomics, which focuses on studying the relationship between imaging phenotypes and genomic phenotypes of specific diseases. Technologies such as AI/ML and DL have demonstrated their ability to extract meaningful information from medical imaging data, sometimes with greater precision than humans themselves and helped bring automated, accurate, and ultra-fast medical image analysis into the mainstream.
For example, researchers in Japan have used AI to successfully detect recurrent prostate cancer by analysing pathology images to identify features relevant to cancer prognosis that were not previously noted by pathologists.
AI techniques like deep learning have even been used to predict neurological diseases like Alzheimer's and amyotrophic lateral sclerosis (ALS) before patients become symptomatic. By training convolutional neural networks on images of motor neurons prepared from iPS cells of 15 healthy donors and 15 ALS patients, researchers were able to predict with over 0.97 of area under curve (AUC) whether donors were healthy or ALS patients.
Omics analysis
AI, ML and Dl have been used extensively across a range of applications including the improvement of disease predictions, establishing the MoAs of compounds, studying gene regulation, and molecular profiling of various diseases, to name a few.
Take the case of cancer, for instance, where treatments based solely on pathological features can yield very different outcomes. It is therefore important to be able to break down broad symptomatic classifications into more finely defined subtypes in order to administer more focused clinical care.
By applying supervised and unsupervised learning techniques to RNA, miRNA, and DNA data from hepatocellular carcinoma patients, researchers were able to identify two subgroups with significant survival differences, isolate consensus driver genes associated with survival, and establish that consensus driver mutations were associated more with mRNA transcriptomes than with miRNA transcriptomes. AI has also been used to focus cancer prognosis and therapy by detecting subtypes among cancer patients, identifying biomarkers that determine recurrence of cancer, and for drug response modelling to predict drug response behaviour.
In drug development, techniques like gene signatures analysis and high-throughput screening have made it quicker and easier to identify compounds that affect a specific target or phenotype. Even so, these compounds may still induce complex downstream functional consequences that could adversely affect their chances of approval. Understanding the MoAs (modes of action) of compounds is crucial to increasing the success rate of clinical trials and drug approvals. Researchers have demonstrated that it is possible to determine unknown MoAs, even in the absence of a comparable reference, by combining multi-omics with an interpretable machine learning model of transcriptomics, epigenomics, metabolomics, and proteomics.
Natural Language Processing
As omics data continues to pile up in the petabytes, it only amplifies the urgency to convert this data into meaningful biological and clinical insights. However, not all omics researchers and bioinformaticians have the statistical expertise to do so. It is in this context that AI techniques like NLP can help accelerate the pace of multi-omics research by making analytics accessible to a wider audience.
For example, one open-access, natural language-oriented, AI-driven platform for analyzing and visualizing cancer omics data empowers users to interact with the system using a natural language interface. Researchers ask biological questions, and the system responds by identifying relevant genomics datasets, performing various analyses, returning appropriate results and even learning from user feedback.
One AI model uses NLP to analyse short speech samples from a clinically administered cognitive test to predict the eventual onset of Alzheimer’s disease within healthy people with an AUC of 0.74.
NLP can also help researchers identify, relate, and analyse datasets from public repositories with a solution that combines NLP techniques, biomedical ontologies, and the R statistical framework to simplify the association of samples from large-scale repositories to their ontology-based annotations.
BioStrand Platform - The AI-centric multi-omics platform
AI/ML technologies have become the defining component of multi-omics analysis as they provide the speed, accuracy, and sophistication to deal with voluminous, diverse, heterogeneous, and high dimensional genomic data. At a higher level, they also introduce innovative new capabilities into bioinformatics by augmenting data-driven decision-making and enabling a new era of predictive multi-omics.
The BioStrand SaaS platform was designed from the ground up to fully leverage the potential of these versatile and intelligent technologies.
Take HYFTs™, a biological discovery that enabled us to translate 440 million sequences of different species, types, and formats from across 11 popular public databases into one homogeneous pan-genomic multivariate knowledge database. With HYFTs™, we have decoded the language of omics, which means that we now have the capability to translate any data that researchers might bring to our platform and make it instantly computable.
Defining a universal framework to accommodate all omics data, public and proprietary, opens up whole new possibilities for innovation. For instance, with our platform, researchers can seamlessly integrate all kinds of structured and unstructured non-biological data including patient record data, scientific literature, clinical trial data, chemical data, ICD codes, lab tests, and more.
However, developing a framework that unifies all omics data and metadata, both public and proprietary, into one integrated model is only half the battle. Truly intelligent and integrated multiomics analysis will only become possible with the seamless integration of years of valuable experimental research insights scattered across volumes of scientific literature. These are insights with the potential to amplify, accelerate and transform bioinformatics that is often left on the table just because of the lack of easy-to-use textual data integration frameworks.
And that’s where the BioStrand platform comes into play, enabling the efficient integration of textual research data together with multiomics data for holistic analysis using sophisticated AI/ML technologies.
Though there are several biomedical NLP solutions available to the research community, they are currently rather confined in terms of application. The primary issue is that, as mentioned earlier, most of these solutions require significant computational and statistical proficiency, limiting their access to a select few. Secondly, many of these solutions use a top-down approach that is effective in extracting information related to a specific query. However, this approach is not very accurate or efficient at extracting all the information, at scale, that is pertinent to research.
BioStrand Platform is designed around a bottom-up approach that can reveal all research-relevant information about novel concepts and relationships available in scientific literature. And since our platform is domain agnostic and is, therefore, capable of identifying all meaningful relationships without the need for predefined knowledge. This means that researchers can completely bypass the training stage and get straight to their research.
Integrated, intelligent multi-omics with BioStrand Platform
BioStrand’s unique sequence + text approach enables researchers to instantly unify all omics, non-omics and unstructured data into one multidimensional dataset that serves as the single source of truth for their research. Combine this with our platform’s constantly evolving advanced AI-powered analytics capabilities and researchers have a seamless, user-friendly and integrated data-ingestion-to-insight multi-omics platform that enables holistic biological research.
To explore what the BioStrand platform can do for your research, contact us here.
Subscribe to our Blog and get new articles right after publication into your inbox.