Improving drug safety with adverse event detection using NLP
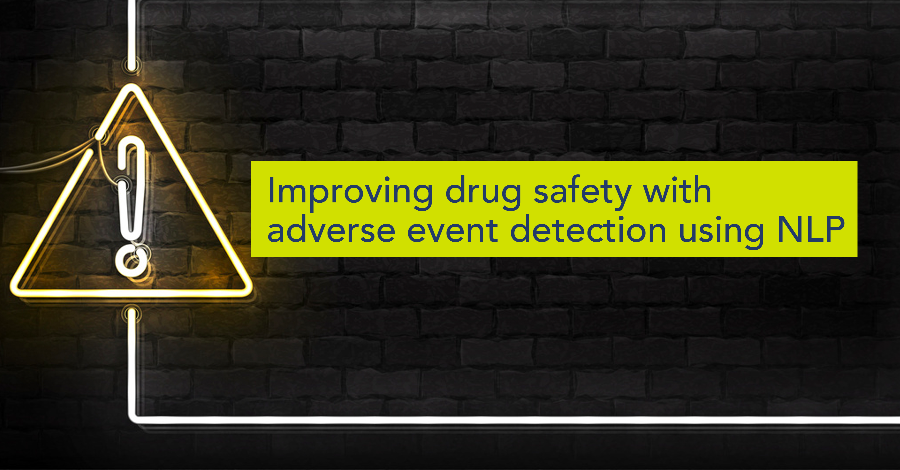
It is estimated that Adverse Events (AEs) are likely one of the 10 leading causes of death and disability in the world. In high-income countries, one in every 10 patients is exposed to the harm that can be caused by a range of adverse events, at least 50% of which are preventable. In low- and middle-income countries, 134 million such events occur each year, resulting in 2.6 million deaths.
Across populations, the incidence of AEs also varies based on age, gender, ethnic and racial disparities. And according to a recent study, external disruptions, like the current pandemic, can significantly alter the incidence, dispersion and risk trajectory of these events.
Apart from their direct patient health-related consequences, AEs also have significantly detrimental implications for healthcare costs and productivity. It is estimated that 15% of total hospital activity and expenditure In OECD countries is directly attributable to adverse events.
There is therefore a dire need for a systematic approach to detecting and preventing adverse events in the global healthcare system. And that’s exactly where AI technologies are taking the lead.
AI applications in adverse drug events (ADEs)
A 2021 scoping review to identify potential AI applications to predict, prevent or mitigate the effects of ADEs homed in on four interrelated use cases.
- First use case: Prediction of patients with the likelihood to have a future ADE in order to prevent or effectively manage these events.
- Second use case: Predicting the therapeutic response of patients to medications in order to prevent ADEs, including in patients not expected to benefit from treatment.
- Third use case: Predicting optimal dosing for specific medications in order to balance therapeutic benefits with ADE-related risks.
- Fourth use case: Predicting the most appropriate treatment options to guide the selection of safe and effective pharmacological therapies.
The review concluded that AI technologies could play an important role in the prediction, detection and mitigation of ADEs. However, it also noted that even though the studies included in the review applied a range of AI techniques, model development was overwhelmingly based on structured data from health records and administrative health databases. Therefore, the reviewers noted, integrating more advanced approaches like NLP and transformer neural networks would be essential in order to access and integrate unstructured data, like clinical notes, and improve the performance of predictive models.
NLP in pharmacovigilance
Spontaneous reporting systems (SRSs) have traditionally been the cornerstone of pharmacovigilance with reports being pooled from a wide range of sources. For instance, VigiBase, the global database at the heart of the World Health Organization’s international global pharmacovigilance system, currently holds over 30 million reports of suspected drug-related adverse effects in patients from 170 member countries.
The problem, however, is that spontaneous reporting is, by definition, a passive approach and currently fewer than 5% of ADEs are reported even in jurisdictions with mandatory reporting. The vast majority of ADE-related information resides in free-text channels: emails and phone calls to patient support centres, social media posts, news stories, doctor-pharma rep call transcripts, online patient forums, scientific literature etc. Mining these free text channels and clinical narratives in EHRs can supplement spontaneous reporting and enable significant improvements in ADE identification.
NLP & EHRs
EHRs provide a longitudinal electronic record of patient health information captured across different systems within the healthcare setting. One of the main benefits of integrating EHRs as a pharmacovigilance data source is that they provide real-time real-world data. These systems also contain multiple fields of unstructured data, like discharge summaries, lab test findings, nurse notifications, etc., that can be explored with NLP technologies to detect safety signals. And compared to SRSs, EHR data is not affected by duplication or under- or over-reporting and enables a more complete assessment of drug exposure and comorbidity status.
In recent years, deep NLP models have been successfully used across a variety of text classification and prediction tasks in EHRs including medical text classification, segmentation, word sense disambiguation, medical coding, outcome prediction, and de-identification. Hybrid clinical NLP systems, combining a knowledge-based general clinical NLP system for medical concepts extraction with a task-specific deep learning system for relations identification, have been able to automatically extract ADE and medication-related information from clinical narratives.
But some challenges still remain, such as the limited availability and complexity of domain-specific text, lack of annotated data, and the extremely sensitive nature of EHR information.
NLP & biomedical literature
Biomedical literature is one of the most valuable sources of drug-related information, stemming both from development cycles as well as the post-marketing phase. In post-marketing surveillance(PMS), for instance, scientific literature is becoming essential to the detection of emerging safety signals.
But with as many as 800,000 new articles in medicine and pharmacology published every year, the value of NLP in automating the extraction of events and safety information cannot be overstated.
Over the years, a variety of NLP techniques have been applied to a range of literature mining tasks to demonstrate the accuracy and versatility of the technology.
Take PMS, for example, a time-consuming and manual intellectual review process to actively screen biomedical databases and literature for new ADEs. Researchers were able to train an ML algorithm on historic screening knowledge data to automatically sort relevant articles for intellectual review. Another deep learning pipeline implemented with three NLP modules not only monitors biomedical literature for ADR signals but also filters and ranks publications across three output levels.
NLP & social media
There has been a lot of interest in the potential of NLP-based pipelines that can automate information extraction from social media and other online health forums.
But these data sources, specifically social media networks, present a unique set of challenges. For instance, ADR mentions on social media typically include long, varied and informal descriptions that are completely different from the formal terminology found in PubMed. One proposed way around this challenge has been to use an adversarial transfer framework to transfer auxiliary features from PubMed to social media datasets in order to improve generalization, mitigate noise and enhance ADR identification performance.
Pharmacovigilance on social media data has predominantly focused on mining ADEs using annotated datasets. Achieving the larger objective of detecting ADE signals and informing public policy will require the development of end-to-end solutions that enable the large-scale analysis of social media for a variety of drugs. One project to evaluate the performance of automated AE recognition systems for Twitter warned of a potentially large discrepancy between published performance results and actual performance based on independent data. The transferability of AE recognition systems, the study concluded, would be key to their more widespread use in pharmacovigilance.
All that notwithstanding, there is little doubt that user-generated textual content on the Internet will have a substantive influence on conventional pharmacovigilance processes.
Integrated pharmacovigilance
Pharmacovigilance is still a very fragmented and uncoordinated process, both in terms of data collection and analysis. The value of NLP technologies lies in their ability to unlock real-time real-world insights at scale from data sources that will enable a more proactive approach to predicting and preventing adverse events. But for this to happen, the focus has to be on the development of outcome-based hybrid NLP models that can unify all textual data across clinical trials, clinical narratives, EHRs, biomedical literature, user-generated content etc. At the same time, the approach to the collection and analysis of structured data in pharmacovigilance also needs to be modernised to augment efficiency, productivity and accuracy. Combining structured and unstructured data will open up a new era in data-driven pharmacovigilance.
Subscribe to our Blog and get new articles right after publication into your inbox.