Computational biology and drug discovery: what to expect in 2023
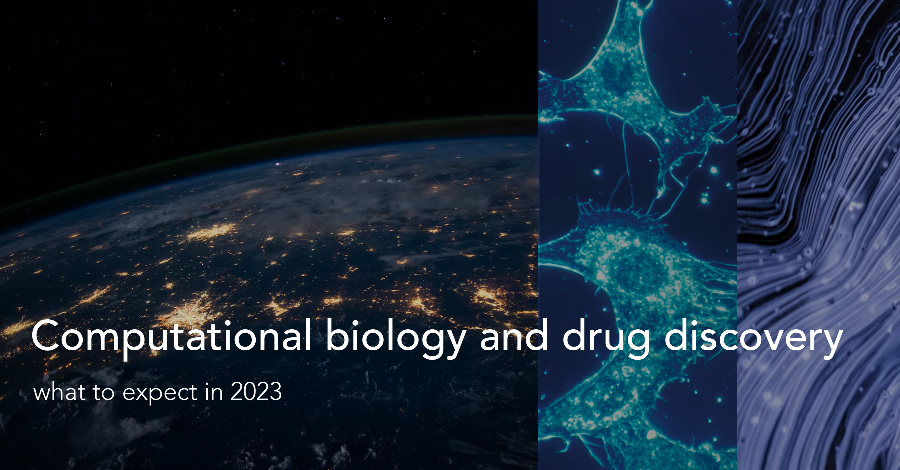
The year 2022 in computational biology was largely marked by two main advancements:
1. A range of rapid developments on the theme of protein structure prediction (started by DeepMind’s AlphaFold)
2. Spillover of recent progress in natural language processing (NLP), such as transformer models, into the world of biological sequences
In this blog we are going to take these recent advances as the basis for our guessing on the coffee grounds what the year 2023 might bring for computational biology in general and in silico drug discovery in particular.
Beyond AlphaFold
It is a truth universally acknowledged that DeepMind’s AlphaFold model for structure prediction has opened a new era in Biology. On the one hand, we now have high-quality structural models for over 200 million proteins and multiple proteomes. On the other, the openly released AlphaFold model serves as an important source of inspiration for other structure prediction tools (see https://github.com/biolists/folding_tools for a nice human curated list of folding predictors).
One can identify already several trends set by these newer tools:
· The limit in speed and scalability of structure prediction has not been reached yet. Some of the newer tools are several orders of magnitude faster than AlphaFold without sacrificing the accuracy of the predicted protein models. Importantly, scalability is of primary importance if one wants to enrich larger datasets, such as datasets of immune repertoires, with structural models.
· We also observe that many of the newer structure prediction tools are dropping the computationally and time-expensive steps of multiple sequence alignment (MSA) optimization, which has a direct impact on scalability. To compensate for the information lost by this decision, diverse groups often utilize protein encoding coming from different language models (more on that follows below).
· Specialized structure prediction models – such as those trained on structures of antibodies, or those based on antibody language models – outperform original AlphaFold in their specialized subdomain. For example, several tools have been optimized for predicting the structure of CDR3 loops (IgFold, EquiFold, ABLooper, to name a few). The speed of these tools is truly remarkable,although it seems that their accuracy has nearly converged.
We believe that in 2023, protein structure prediction will become even faster and more specialized, though it remains to be seen whether any notable progress in prediction accuracy can be achieved.
AlphaFold and friends have produced by now a number of hugely interesting structural databases. One immediate way to make these databases directly actionable is to render them searchable. The state-of-the-art solution for structural similarity search which has emerged in 2022 is FoldSeek. Available both as a server and as a standalone tool, FoldSeek is sensitive and very fast, and opens new points of view on many applications, such as remote homology search.
We are confident that in the coming year, increasingly large databases of predicted structures of antibodies will become available. In combination with sensitive structural search capabilities, this will provide previously unseen potential for in silico drug discovery projects, such as humanization efforts or finding antibodies with prescribed function.
Language models for proteins in general and antibodies in particular
Cheap and easy availability of structural models for a wide variety of proteins has changed the way we think and talk about proteins. If before the AlphaFold moment most proteins were primarily defined by their amino acid sequence, nowadays structural representation is usually favored, since it is generally more informative. However, there is a new syntaxis which has been becoming increasingly important and widely applied – language models for proteins have supplied new representations for proteins, usually in the form of vectors in some high-dimensional space. It turned out that such vectors can carry interesting information about a protein’s function, structure, and other features (visit https://embed.protein.properties to get a glimpse at the power of such representation). These vectors are already finding their way into traditional databases such as Swiss-Prot. Though interpretation of such vectorial representations typically is somewhat obscure, being simple numeric objects, they do provide extremely convenient features for many downstream tasks.
In 2023, we will continue getting used to the fact that a protein, as an entity, can have multiple representations useful in different contexts. Sequence, structure, vector, and different combinations thereof have become parts of our new metalanguage describing proteins, including antibodies. We expect that language models will keep being finetuned for many new prediction tasks. However, there is hardly any reason to stop here, and we predict that in the coming year, new useful modalities to represent proteins will be discovered and put to use.
Bonus
We cannot resist mentioning another topic, which might be less populated at the moment, but which has enormous potential. Protein generative models based on diffusion rely upon the same principles as OpenAI’s text-to-image system (check out DALL·E 2 for example). These new generative models promise to design proteins with prescribed shape, symmetries, and other properties. We believe that models of this sort as well as methods for sequence-structure co-design will gain in popularity and usage next year. The future is very exciting indeed!
Subscribe to our Blog and get new articles right after publication into your inbox.