Bring Meaning to the Chaotic Space of Omics & Text
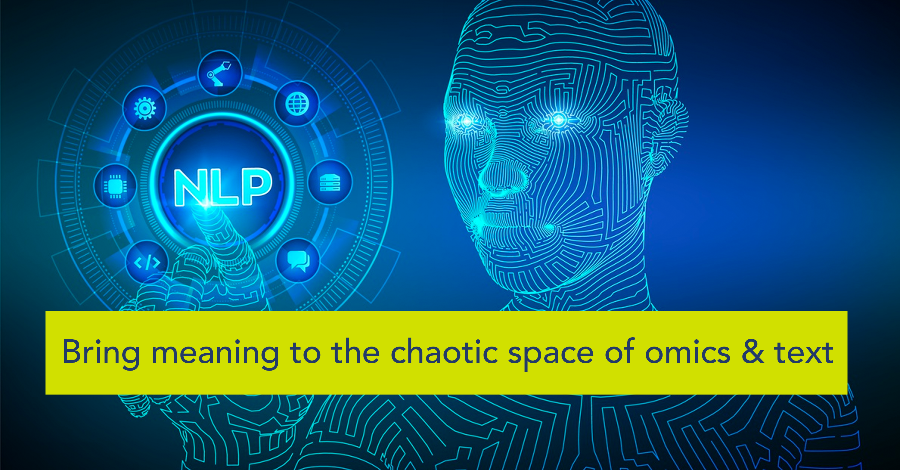
Learn how to integrate and normalise omics and biomedical text data into a single data model/frame at our webinar Bring Meaning to the Chaotic Space of Omics & Text – 15:30, 17 November 2021. Watch it now.
What if the immune system interprets viruses the same way that we humans process sentences?
That is the intriguing hypothesis being explored by researchers at the Massachusetts Institute of Technology.
In order to understand the complex rules that govern viral escape – the rapid mutation of viruses that enable them to evade antibodies – the research team has turned to linguistic concepts of grammar and semantics. In the context of interpreting the evolutionary fitness of a virus to infect a host, a successful infectious virus is not only one that is grammatically correct, but one that is also capable of mutating, or altering its meaning, to make itself invisible to antibodies.
In order to map this process, the researchers trained an NLP model on small volumes of HIV, influenza, and coronavirus sequence data. They then used it to predict sequences of the coronavirus spike protein, HIV envelope protein, and influenza hemagglutinin (HA) protein that could be more likely to generate escape mutations.
AI/ML-based language models are being increasingly applied across the spectrum in computational biology, clinical care, and healthcare.
Take the pharmaceutical and life sciences industries, for instance, where 80% of the data with the potential to drive clinical and commercial outcomes still exists as unstructured text. The availability of the right tools to extract insights from this vast data trove would enable researchers in these industries to explore innovative new opportunities in enhancing patient safety, improving clinical trial design, and identifying previously undetected biomarkers.
In drug discovery, NLP has potentially critical applications across the development pipeline, from analysing clinical trial digital pathology data to identifying predictive biomarkers, and can significantly accelerate data analysis while reducing failure rates. AI-powered language models could also play a potential role in the development of new treatment strategies for pandemics, including drug repurposing for the treatment of other infectious diseases.
Natural language processing is also a viable alternative to the laborious manual encoding of clinical data and has the potential to enhance reproducibility at scale. Research shows that NLP-based pipelines for the extraction of Human Phenotype Ontology (HPO) terms can replace manual extraction in over 90% of cases and identify the correct causal gene.
In oncology, NLP could significantly improve cancer research and epidemiology by eliminating the inaccuracies and biases of manually identifying and abstracting data for cancer registries. Recent advances in NLP techniques have also opened up potential new applications in clinical oncology, including clinical timeline creation and automated clinical trial matching.
One study of an NLP-based trial eligibility pre-screening model found that it could drastically reduce physician workloads in identifying patients eligible for trials, as well as identifying trials for which patients were eligible – both with a high degree of precision.
NLP techniques indeed open up a gamut of possibilities, from protein research, extracting protein-protein interactions affected by mutations from large volumes of literature, automated information extraction and relevance scoring for proactive event-based surveillance of potential outbreaks, extracting meaningful statistical and biological information from high-dimensional data, and more.
And as the volume of textual biomedical data continues to increase exponentially, advanced NLP techniques will provide an invaluable approach to knowledge extraction.
However, the application of advanced NLP techniques in biomedical research is not without its challenges.
For instance, approaches for computing semantic sentence similarity in generic English may not necessarily cope effectively with biomedical knowledge or produce the best results for biomedical text. In fact, one study was able to demonstrate that even state-of-the-art general domain sentence similarity computation systems were rather ineffective at dealing with biomedical texts.
Therefore, the focus has to be on the development of biomedical domain-specific approaches using string similarity measures, ontology-based measures, a distributional vector model, and a supervised method combining these different measures.
However, there is no doubt that advances in AI/ML-based text mining techniques can help address the gaps in omics data and enable novel biomedical discoveries. The biggest challenge in this context will be in the integration of omics and textual data under one unified and comprehensive analytic framework.
The BioStrand Platform
This is the challenge that we are seeking to address with the BioStrand Platform – the perfect mix of cross-domain expertise in the domain of omics data and NLP technology. Researchers can now add any data, biological or text, from any source, and the BioStrand data management platform integrates and normalises this data into a single data model/frame.
The platform’s bottom-up approach to omics and NLP means that users require zero pre-training to leverage our advanced data analysis capabilities to detect novel associations or validate existing relationships.
Webinar – Bring Meaning to the Chaotic Space of Omics & Text
In this BioStrand Webinar Wednesday session, We discussed BioStrand’s unique approach to omics & text data analytics.
The webinar features Dirk van Hyfte, our Head of Innovation, explaining the concept of BioStrand’s CRC approach, Sébastien Lemal, our Data Scientist, detailing how you can use our NLP technology to extend any bioinformatics pipeline, and Arnout van Hyfte, our Head of Products & Platform, discussing the importance of putting data to the centre of your research to validate and find hypotheses using a bottom-up data-driven approach.
Plus, we had an extended Q&A session to address any specific queries you may have about integrating omics and NLP in your current research projects.
Watch the replay now of the Bring Meaning to the Chaotic Space of Omics & Text webinar.
Subscribe to our Blog and get new articles right after publication into your inbox.