AI-powered genomics to advance precision medicine
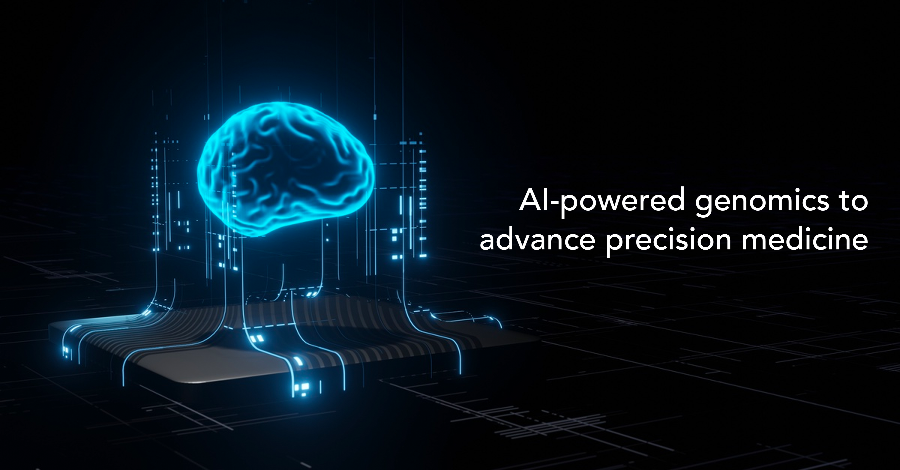
Sir William Osler, often called the father of modern medicine, was among the first to articulate a patient-centric pursuit of medicine when he said “The good physician treats the disease; the great physician treats the patient who has the disease.” Today, making this patient-centric model of healthcare the new normal is the core focus of the merging science of precision medicine.
Most medical treatments are designed for the average patient, which means that they may not be as successful for some patients as they are for others. Precision medicine represents an evolutionary shift from the conventional one-size-fits-all approach to healthcare towards matching therapies that are most effective for the individual. According to the Precision Medicine Initiative, precision medicine is "an emerging approach for disease treatment and prevention that takes into account individual variability in genes, environment, and lifestyle for each person."
This defined focus on individual patients means that the terms "personalised medicine" and "precision medicine" are often used interchangeably. In 2011, the United States National Research Council coined a new definition to emphasise that precision medicine does not literally mean the creation of therapies that are unique to every individual patient, but rather the ability to classify individuals into subpopulations that differ in their susceptibility to a particular disease or in their response to a specific treatment.
Genomics and precision medicine
The completion of the Human Genome Project in 2003 paved the way for the relatively new field of genomic medicine that has since grown quickly. Genomic medicine is an emerging medical discipline that uses individual genomic information for diagnostic/therapeutic decision-making in clinical care and has already had a significant impact on several fields including oncology, pharmacology, rare and undiagnosed diseases, infectious diseases etc.
However, genomic medicine is a subset of precision medicine and genomic information is only one piece of the puzzle. But when combined with clinical, pharmaceutical, and lifestyle information, patient genomic data can help discover early signs of disease, determine individual risk levels for specific diseases and lead to more personalised, effective treatments.
Genomic data, and modern bioinformatics solutions to analyse this data, have played a central role in the global health response to the COVID-19 pandemic. Going forward, the integrated analysis of multiple omics profiles, such as genome, transcriptome, proteome and other omics information, will be essential for the continued evolution of precision medicine.
Today, NGS technologies have significantly accelerated the generation of rich and accurate genomics data with the potential to transform precision medicine. But the analysis of these exponentially-growing volumes of data still poses quite a few challenges.
Genomic data challenges
Genomic data is key to uncovering how differences in DNA affect human health and disease. However, the capabilities to sequence DNA are growing at a faster pace than our ability to decipher the information generated. Genomic data is currently growing faster than every other big data domain, doubling in size every 7 months and projected to grow to as much as 40 exabytes within the next decade.
However, genomic data is not purely a big data problem due to several unique characteristics that present particular challenges. Genomic data is extremely heterogeneous, generated using different technologies, represented in multiple formats, and distributed across hundreds of repositories with varying levels of quality without any centralised control or coordination. Consequently, efficient, accurate, at-scale knowledge extraction continues to be a challenge. The focus is now turning to AI to help make sense of genomic data and obtain actionable insights that can be incorporated into routine clinical care.
Genomic data and AI
The increasing scale and complexity of genomic sequencing data as well as other biomedical data points is accelerating the development of AI computational tools to quickly and accurately identify genetic diseases in patients and provide the data foundation for precision medicine treatments. The AI in Genomics market is projected to expand at a CAGR of over 50% to reach accumulated revenue of USD 18 billion by 2030.
Though still at a very early stage, AI/ML tools in genomics have seen several innovative applications including facial analysis algorithms that accurately identify genetic disorders to ML techniques that can identify the primary kind of cancer from a liquid biopsy.
Deep learning approaches show great potential to reveal genomic differences, such as inherited genetic variants, that are causally linked to traits or diseases. By integrating big biological data sets, DL models have been able to predict changes based on genomic differences in the complex cellular processes that lie between genotype and phenotype. These models are able to pinpoint putative causal variants for several cardiological, neurological and immune-related conditions.
When it comes to genome-based diagnosis, especially of rare genetic diseases, clinical interpretation of genetic variants in the context of the patient’s phenotype is the largest component of cost and time expenditure. AI-based tools have been proven to significantly decrease cost and expedite case reviews by automating genetic disease diagnosis.
In fact, AI systems have not only demonstrated their abilities to cope with the idiosyncrasies of genomic data but also to transform them into actionable insights. They also engender several critical capabilities such as unifying and normalising disparate omics datasets, democratising high-throughput data analysis, accelerating discovery, and expediting diagnoses.
Genomics and precision medicine
The genome is the informational core of the life sciences and genomic medicine is a critical component of precision medicine. The healthcare industry is already in the age of precision medicine and its impact on healthcare delivery, treatment choices and patient outcomes have already been demonstrated across several disease areas such as oncology, cardiology, and endocrinology.
Even though genomic medicine has advanced considerably over the last two decades, the grand challenge of harnessing and making sense of genomic big data remains to be fully resolved. AI technologies offer enormous potential in addressing almost all of the data analysis challenges associated with genomic data and accelerating the evolution of genomic medicine.
In the broader context of precision medicine, AI is expected to deliver transformative opportunities across three levels; one, predicting pharmaceutical properties of molecular compounds and targets for drug discovery, two, faster diagnosis using pattern recognition and segmentation techniques, and three, the development of predictive models using DL techniques on multimodal data sources such as genomic and clinical data. There have already been several tangible successes in the first two. The availability of AI-powered genomics solutions will, therefore, be critical to the successful development of precision medicine.
Subscribe to our Blog and get new articles right after publication into your inbox.