AI/ML, Big Data, and Intelligent Bioinformatics
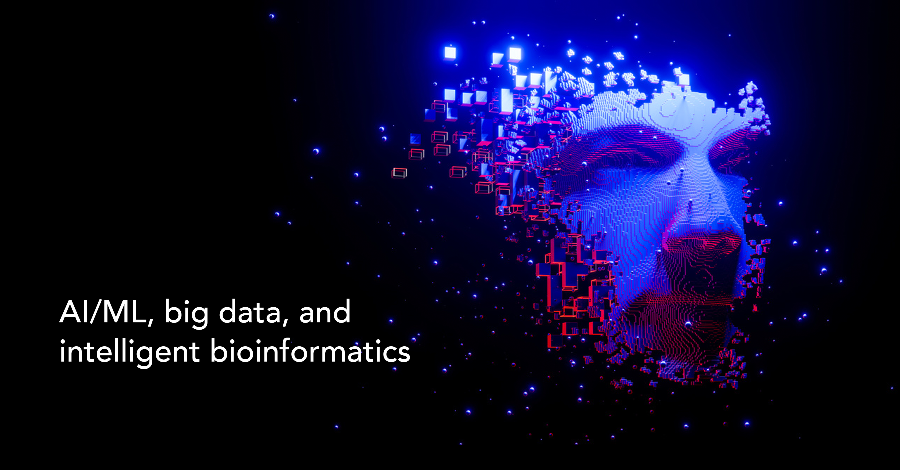
Decoding protein folding, i.e. understanding how a linear chain of amino acids folds into three-dimensional structures that determine biological function, has been an enduring problem for decades. A deep understanding of protein structures plays a central role in critical applications in biology, chemistry, and medicine.
And yet, half a century of dedicated research has gone into unlocking the structure of just 17% of the estimated 400,000 proteins in the human body.
But thanks to AlphaFold, an AI system developed from UK-based DeepMind, we now have predicted protein structures for almost the entirety of the human proteome as well as those of model organisms like the fruit fly and E.coli. In addition, DeepMind plans to keep expanding this database to cover the 100 million proteins catalogued in the UniRef90 database and make it available for free, in perpetuity, for all scientific and commercial research.
An AI-based computational approach to accelerated, accurate, and scalable protein folding research opens up a range of new opportunities for downstream innovations. For instance, researchers at the University of Washington are already exploring the potential of leveraging deep learning’s protein structure prediction capabilities to “hallucinate” a whole new world of synthetic proteins with targeted functions.
A new breed of startups is already coalescing around this seminal breakthrough to develop next-generation protein-based therapeutics targeting COVID-19 and cancer.
AI/ML in Big Data Bioinformatics
The exponential growth of biological Big Data in the post-genomics era has required a complete transformation of traditional bioinformatics and conventional approaches to the acquisition, storage, distribution, and analysis of raw data. The prodigious big data processing capability of AI technologies is often the prime mover for the integration of machine learning and deep learning capabilities to conventional research pipelines.
Genomic data also tends to be remarkably heterogeneous and extremely distributed in nature. In this context, novel AI/ML-based techniques can help streamline and accelerate the process of normalising data from multimodal sources, including multi-omics data, clinical trial data, patient records, etc., and enable the integrated analysis of all research-relevant data.
The application of intelligent technologies can also open access to new data sources that were hitherto beyond the purview of conventional data integration and analysis frameworks. For instance, the exponential increase of digital biomedical information, and the lack of automated scalable solutions to convert all that unstructured data into structured data, leaves a lot of potential research value on the table.
Biomedical-domain-specific NLP techniques open up a gamut of possibilities in automating the extraction of statistical and biological information from large volumes of text including scientific literature and medical/clinical data.
And finally, AI/ML technologies and their capacity for high-volume high-dimensional data can deliver remarkable breakthroughs – as demonstrated by the application of deep learning to protein folding – that transforms biological research. However, the emphasis has to be on the application of innovative technologies across the research spectrum, from data generation to analysis and distribution.
Three transformative applications for AI/ML
AI in rare disease detection
In a big data world, rare diseases (RDs) represent a collection of small data challenges that involve sample sizes, potential study designs, and achieving conventional levels of statistical significance. Specific to AI/ML, the biggest challenge is the unusual big data regime of high dimensions and low sample sizes.
The wider availability and lower cost of NGS technologies have already established whole-exome and whole-genome sequencing as a key part of the rare disease diagnostic and research process. AI algorithms, with mutation detection, prediction, and classification capabilities, can help uncover new disease mechanisms and therapeutic targets.
AI-based integrative analytics strategies are also enabling a new multi-omics approach to rare disease research. Besides, AI has also helped boost therapeutic development in terms of patient recruitment, identifying biomarkers, and drug discovery.
There are currently several initiatives underway, including Orphanet, European Joint Programme on Rare Diseases (EJP RD), and Undiagnosed Diseases Network (UDN), to build a global network of related data, resources and expertise. These efforts can provide the preliminary foundations to integrate AI/ML technologies in order to address the diagnosis and treatment challenges associated with rare diseases today.
AI in drug discovery/repurposing
During the early days of the pandemic, researchers were able to use a pre-trained deep learning-based drug-target interaction model to identify commercial drugs that could potentially be repurposed for SARS-CoV-2. Though not yet clinically approved, it is a perfect showcase of how big data and AI could maximize the impact of drug discovery by balancing speed to market with the cost of development.
According to a 2020 Deloitte survey, biopharma companies are already experimenting with AI to accelerate drug discovery. Over the next five years, AI is expected to become an intrinsic aspect of the drug discovery process with AI models being used to identify/validate targets, and to design, synthesise and test potential molecules.
AI imaging capabilities are being harnessed to detect cell morphology changes, and AI algorithms to create knowledge graphs that map complex relationships between compounds, genes, diseases, and proteins. Generative modelling is expected to become a key component of the computational toolkit that will enable companies to explore novel spaces and broaden the pool of drug candidates.
AI in personalised medicine
Precision medicine is about tailoring medical treatment to the individual patient rather than to the average patient. One field that has seen rapid advances is cancer care, which is undergoing a shift towards precision oncology.
Most cancers are still treated by surgical resection followed by chemotherapy. Though this approach has led to an increase in progression-free and overall survival, the regimen still falls short for a majority of patients. In oncology, therefore, there is pressing need to tailor therapeutic regimens to individual genetic profiles.
For instance, researchers have found that it is possible to accurately predict the response to breast cancer using a multi-omic machine learning model integrating clinical, molecular, digital pathology, and treatment data.
Multiple studies have also shown that quantitative imaging techniques combining radiomics and deep learning could provide an accurate and reproducible approach to personalisation, with applications in risk stratification, early diagnosis, and improved patient management.
Going forward, AI and ML will play a critical role in the integrated analysis of large datasets combining imaging (histopathology and radiology) and molecular data (genomics and proteomics). An integrated approach will further augment the scope of precision oncology by providing diagnostic, prognostic and predictive insights for more personalized cancer care.
The future of AI/ML in bioinformatics
AI/ML applications will be a dominant theme in the progression of biological data science and in the development of next-generation intelligent bioinformatics platforms. The focus, however, has to be on a more integrated approach to harness the power of these technologies so that:
- They are applied consistently across the bioinformatics research continuum, from data acquisition to analysis to distribution/collaboration.
- They enable integrated research across multiple biological subdisciplines.
- They enable research at any scale, from the molecular to the systemic.
- And they incorporate biological/biomedical specific AI/ML models to maximize the value of bioinformatics.