Artificial Intelligence in early phase drug development
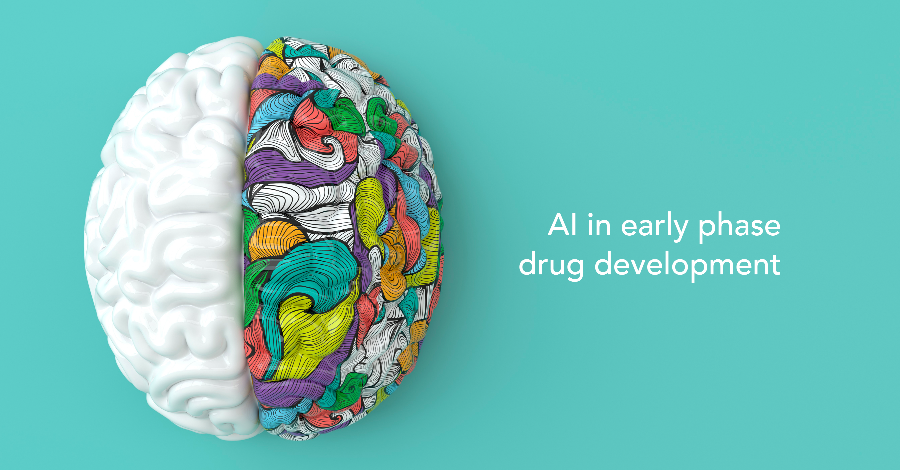
Artificial intelligence (AI) technologies are currently the most disruptive trend in the pharmaceutical industry. Over the past year, we have quite extensively covered the impact that these intelligent technologies can have on conventional drug discovery and development processes.
We charted how AI and machine learning (ML) technologies came to be a core component of drug discovery and development, their potential to exponentially scale and autonomize drug discovery and development, their ability to expand the scope of drug research even in data-scarce specialties like rare diseases, and the power of knowledge graph-based drug discovery to transform a range of drug discovery and development tasks.
AI/ML technologies can radically remake every stage of the drug discovery and development process, from research to clinical trials. Today, we will dive deeper into the transformational possibilities of these technologies in two foundational stages — Early Drug Discovery and Preclinical Development — of the drug development process.
Early Drug Discovery and Preclinical Development
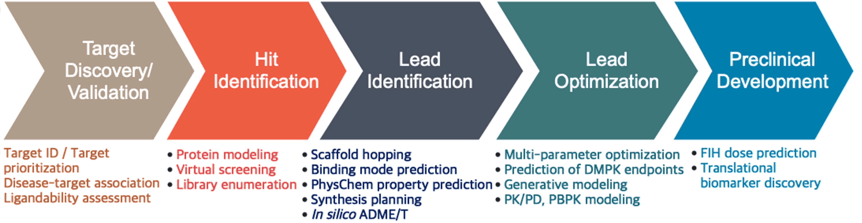
SOURCE: ScienceDirect
Early drug discovery and preclinical development is a complex process that essentially determines the productivity and value of downstream development programs. Therefore, even incremental improvements in accuracy and efficiency during these early stages could dramatically improve the entire drug development value chain.
AI/ML in early drug discovery
The early small molecule drug discovery process flows broadly, across target identification, hit identification, lead identification, lead optimization, and finally, on to preclinical development. Currently, this time-consuming and resource-intensive process relies heavily on translational approaches and assumptions. Incorporating assumptions, especially those that cannot be validated due to lack of data, raises the risk of late-stage failure by advancing NMEs without accurate evidence of human response into drug development. Even the drastically different process of large-molecule, or biologicals, development, starts with an accurate definition of the most promising target. AI/ML methods, therefore, can play a critical role in accelerating the development process.
Investigating drug-target interactions (DTIs), therefore, is a critical step to enhancing the success rate of new drug discovery.
Predicting drug-target interactions
Despite the successful identification of the biochemical functions of a myriad of proteins and compounds with conventional biomedical techniques, the limitations of these approaches come into play when scaling across the volume and complexity of data. This is what makes ML methods ideal for drug–target interaction (DTI) prediction at scale.
L techniques ideal for drug-target interaction prediction.
There are currently several state-of-the-art ML models available for DTI prediction. However, many conventional ML approaches regard DTI prediction either as a classification or a regression task, both of which can lead to bias and variance errors.
Novel multi-DTI models that balance bias and variance through a multi-task learning framework have been able to deliver superior performance and accuracy over even state-of-the-art methods. These DTI prediction models combine a deep learning framework with a co-attention mechanism to model interactions from drug and protein modalities and improve the accuracy of drug target annotation.
Deep learning models perform significantly better at high-throughput DTI prediction than conventional approaches and continue to evolve, from identifying simple interactions to revealing unknown mechanisms of drug action.
Lead identification & optimization
This stage focuses on identifying and optimizing drug-like small molecules that exhibit therapeutic activity. The challenge in this hit-to-lead generation phase is twofold. Firstly, the search space to extract hit molecules from compound libraries extends to millions of molecules. For instance, a single database like the ZINC database comprises 230 million purchasable compounds and the universe of make-on-demand synthesis compounds can be 10 billion. Secondly, the hit rate of conventional high-throughput screening (HTS) approaches to yield an eligible viable compound is just around 0.1%.
Over the years, there have been several initiatives to improve the productivity and efficiency of hit-to-lead generation, including the use of high-content screening (HCS) techniques to complement HTS and improve efficiency and CADD virtual screening methodologies to reduce the number of compounds to be tested.
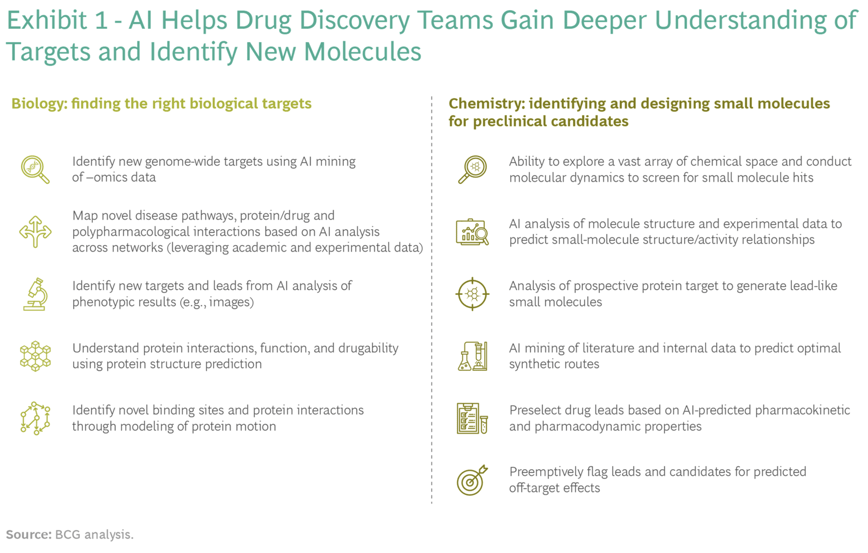
SOURCE: BCG
The availability of huge volumes of high-quality data combined with the ability of AI to parse and learn from these data has the potential to take the computational screening process to a new level.
There are at least four ways — access to new biology, improved or novel chemistry, better success rates, and quicker and cheaper discovery processes — in which AI can add new value to small-molecule drug discovery.
AI technologies can be applied to a variety of discovery contexts and biological targets and can play a critical role in redefining long-standing workflows and many of the challenges of conventional techniques.
AI/ML in preclinical development
Preclinical development addresses several critical issues relevant to the success of new drug candidates. Preclinical studies are a regulatory prerequisite to generating toxicology data that validate the safety of a drug for humans prior to clinical trials.
These studies inform trial design and provide the pharmacokinetic, pharmacodynamic, tolerability, and safety information, such as in vitro off-target and tissue-cross reactivity (TCR), that defines optimal dosage. Preclinical data also provide chemical, manufacturing, and control information that will be crucial for clinical production.
Finally, they help pharma companies to identify candidates with the broadest potential benefits and the greatest chance of success.
It is estimated that just 10 out of 10,000 small molecule drug candidates in preclinical studies make it to clinical trials. One reason for this extremely high turnover is the imperfect nature of preclinical in vivo research models, as compared to in vitro studies which can typically confirm efficacy, MoA, etc., which results in challenges to accurately predicting clinical outcomes.
However, AI/ML technologies are increasingly being used to bridge the translational gap between preclinical discoveries and new therapeutics. For instance, a key approach to de-risking clinical development has been the use of translational biomarkers that demonstrate target modulation, target engagement, and confirm proof of mechanism.
In this context, AI techniques have been deployed to learn from large volumes of heterogeneous and high-dimensional omics data and provide valuable insights that streamline translational biomarker discovery. Similarly, ML algorithms that learn from problem-specific training data have been successfully used to accurately predict bioactivity, absorption, distribution, metabolism, excretion, and toxicity (ADMET) -related endpoints, and physicochemical properties. These technologies also play a critical role in the preclinical development of biologicals, including in the identification of candidate molecules with a higher probability of providing species-agnostic reactive outcomes in animal/human testing, ortholog analysis, and off-target binding analysis.
These technologies have also been used to successfully predict drug interactions, including drug-target and drug-drug interactions, during preclinical testing.
The age of data-driven drug discovery & development
Network-based approaches that enable a systems-level view of the mechanisms underlying disease pathophysiology are increasingly becoming the norm in drug discovery and development.
This in turn has opened up a new era of data-driven drug development where the focus is on the integration of heterogeneous types and sources of data, including molecular, clinical trial, and drug label data.
The preclinical space is being transformed by AI technologies like natural language processing (NLP) that are enabling the identification of novel targets and previously undiscovered drug-disease associations based on insights extracted from unstructured data sources like biomedical literature, electronic medical records (EMRs), etc.
Sophisticated and powerful ML/AI algorithms now enable the unified analysis of huge volumes of diverse datasets to autonomously reveal complex non-linear relationships that streamline and accelerate drug discovery and development.
Ultimately, the efficiency and productivity of early drug discovery and preclinical development processes will determine the value of the entire pharma R&D value chain. And that’s where AI/ML technologies have been gaining the most traction in recent years.
Subscribe to our Blog and get new articles right after publication into your inbox.