FAIRify your data: A guiding principle
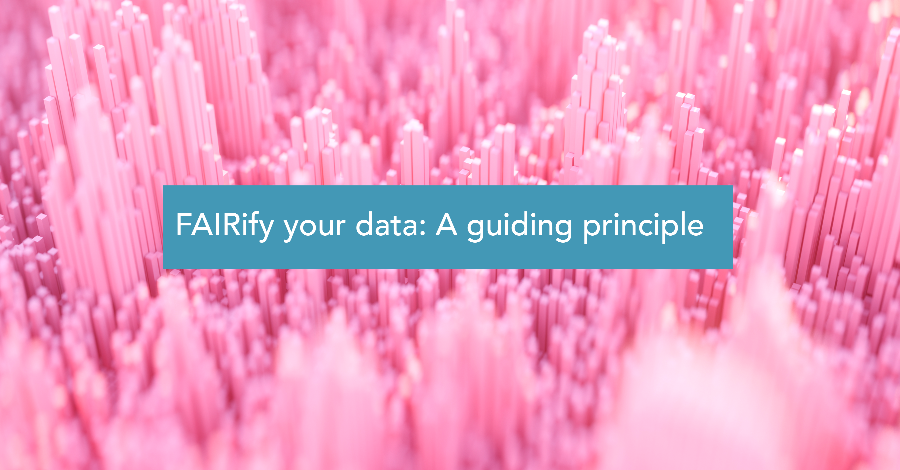
There is an increase in speed, reliability and cost-effectiveness of high throughput sequencing which led to the widespread application of genome, transcriptome in clinical, biomedical, and agricultural sectors. To keep a subtle track of sample relationships and analyses, a robust platform is needed that could unify metadata for diverse sequencing strategies with sample metadata while supporting automation and reproducibility.
Managing the data to get 100% reproducibility has become a challenge for scientific society.
When this research data cannot be found, access, interpreted and reusage is impaired, the impact can be significant. This would hamper data-driven innovation and knowledge discovery, jeopardizing the success of collaborations and mislaying the resources in the areas not contributing to the competitive edge.
Making your data “FAIR” is something you hear more and more in all the growth segments. When we create a large volume of data and a variety of data types, we experience data management difficulties in large-scale projects. This re-iterates the importance and necessity of making the data Findable, Accessible, Interoperable and Reusable.
What is “FAIR” data management?
FAIR is an acronym and stands for Findable, Accessible, Interoperable, and Reusable. The concept has been around since 2016 and gained momentum and traction not only in the academic research environment but also in growing industries. The guiding principles aim at facilitating maximum data reuse and attention as funding bodies, publishers now enforcing their implementation in academic and industrial research environments.
FAIR principles put pressure on the organizations that publish and own the data repositories to be more machine-actionable i.e., a machine that can read the metadata that describes the data and enables it to access and utilize for various applications. This implementation of the FAIR principles will be overarchingly critical to organizations that aim for reproducibility and tackling healthcare challenges.
Reputed institutes like NIH and Elixir have been big supporters of establishing standards for data curation and metadata annotation for reproducibility. These further could be involved in the integration of Big Data based on the FAIR principles.
Are you playing FAIR?
The concerns about FAIR research have long been a constant fixture in conversation among different academia, industry, and other funding organizations. It initially started and focused on the reproducibility of the protocols and techniques used in different experimental conditions across the globe. Having a valuable empirical finding of many studies, most of them suffered the reproducibility across different research settings.
The data revolution triggered by the genome projects and increasing dominance in the high throughput research areas pushed us to look for the quality over quantity of the data produced. The opportunity comes with its own set of challenges which is not surprising. We are in the era of data revolution where a single clinical experiment could produce TBs of data and it continues to grow in public repositories.
This data explosion has triggered the establishment of rigorous standards for reproducibility, accessibility, use and management which are critical requirements to enable innovation and discovery.
How can BioStrand make your data FAIR?
BioStrand has the technology to incorporate both metadata conform FAIR principles yet make it searchable without limits and facilitate complex multi-dimensional analysis across all omics levels and relevant metadata as dimensions.
1. Input data organization and concatenation
The technology developed by BioStrand allows researchers to use sequences or text to simultaneously search through all the publicly available omics databases (including patent databases and proprietary enterprise databases).
BioStrand developed HYFT™ patterns which are signature sequences in DNA, RNA and Amino acids that serve as biological fingerprints and contain a multitude of information layers, for instance, function, structure, position etc that could be used to significantly optimize sequence analysis. Current methods like dynamic programming and heuristic algorithms have so many limitations concerning speed, accuracy and scaling with the dynamics of big data.
Along with these, other challenges include fragmented data types, lack of integrated omics data enable the users to have a holistic picture and insights. The BioStrand platform is the need of an hour with an innovative approach that address these limitations required for handling big data. The platforms have been precomputed and indexed all the information to make the searching the network simple, accurate, comprehensive, and fast.
2. Restructuring the data
Results that are returned instantaneously from the search are very well organized on three levels – DNA, RNA and Amino Acids – with the added advantage. The user will be able to drill down through and explore pathways, associations that are most relevant to their research work reducing their time on restructuring and organizing the data to find some relevance.
This allows the users for more in-depth data exploration, simplifies knowledge extraction, and accelerates insightful details on a single click. The platform provides a very comprehensive set of features where users could sort, filter, group and exclude/include parameters that are appropriate for them. Conventional tools lack this kind of versatility, agility and most importantly it's time-consuming.
Platform address all the above limitations of the conventional tools and custom search solutions could be built across different databases which are powered by the proprietary algorithm of BioStrand.
3. Additional compound information
The BioStrand solution has a comprehensive knowledge base, processes search queries across all the 440 million references sequences from the curated databases to provide an exhaustive, multidimensional array of accurate results in mere seconds.
The primary search helps the users to have a quick overview and further select multiple dimensions such as Gene ontology or any other specific parameters or associations related to the research questions they are addressing. These solutions will help the user to automatically translate these functionalities and progressively applied to annotations, and high-level GO definitions.
4. Enriching the data with controlled vocabulary
To improve interoperability and simplify data re-use in other contexts, the information about the search results is provided along. The users have the option of quick filters and a detailed overview of each result. Further, they could select and explore the alignments using the alignment view, isolate the amino acids, and then dissect to discover their novel functional relationships using the explorer view.
The platform can be customize also the search results to certain databases and visualize the unique and shared results. This layered approach to discovery makes it extremely simple for researchers to explore all available translation pathways.
5. Adding user-defined data
The platform also enables the users to add their custom data for the comparisons and can also use the power of the explorer view to combine multiple dimensions like taxonomy or ontology etc to discover the novel functional relationships. The platform emphasizes a user-friendly experience to make the research through a google simple and intuitive approach.
Users can create their unique route to insights for finding the associations without distracting from the complex framework and processes.
6. Writing the data and workflow
The entire workflow is easy and faster to execute with possible customized options for the users. The BioStrand platform combines HYFT™, a new methodology for organizing and indexing combine that complex data with quick retrieval on all molecular layers. All the data which is generated is accessible, the solution scales organically as data volumes explode making it more user friendly and ready for further usage.
BioStrand platform has been designed to contribute to interoperability, accessibility and reusability,
- It can read, write, and integrate numerous different formats which could be used to extend the metadata with a controlled vocabulary
- Give qualified references for each result shown
- It can add plenty of user-defined metadata
- The workflow summary provides information about the data table that was created and could be reused for other purposes.
Principle | BioStrand | |
Findable | F1 Metadata indexed in the searchable sources with the identifier | Data is indexed with different sources and identifiers for the users |
Accessible | A1 Accessible data with their sources and possibility of reuse | All the data which is generated is accessible, user-friendly and ready for further use |
Interoperable | I1 Data use a formal, accessible, shared and broadly applicable language with qualified references | Data is provided from the qualified references in numerous different formats |
Reusable | R1 Data with relevant attributes and are associated with detailed provenance | Data comes with defined metadata that could be reused and customized |
Harnessing the next-generation computation techniques like indexing and matching for analyzing a large volume of data across different sources with high speed and accuracy is the new shift in omics research.
At BioStrand, we have been putting efforts to create a comprehensive environment that brings data and computation power together to harness the real power of the data for discovery.
We are building high throughput workflows with independent modules, cloud storage infrastructure to enable the scaling of the data analysis.
Our vision is to bring closer the power of computing environments that interact effectively with FAIR data to generate more insights valuable for the upcoming scientific discoveries.
References
- Wilkinson, M., Dumontier, M., Aalbersberg, I. et al. The FAIR Guiding Principles for scientific data management and stewardship. Sci Data 3, 160018 (2016).
- Turning FAIR into reality. European Commission Expert Group on FAIR Data reports 2018.
Image source: Adobe Stock © garrykillian159567645
Subscribe to our Blog and get new articles right after publication into your inbox.