The BioStrand Interviews: Meet Yegor Korovin
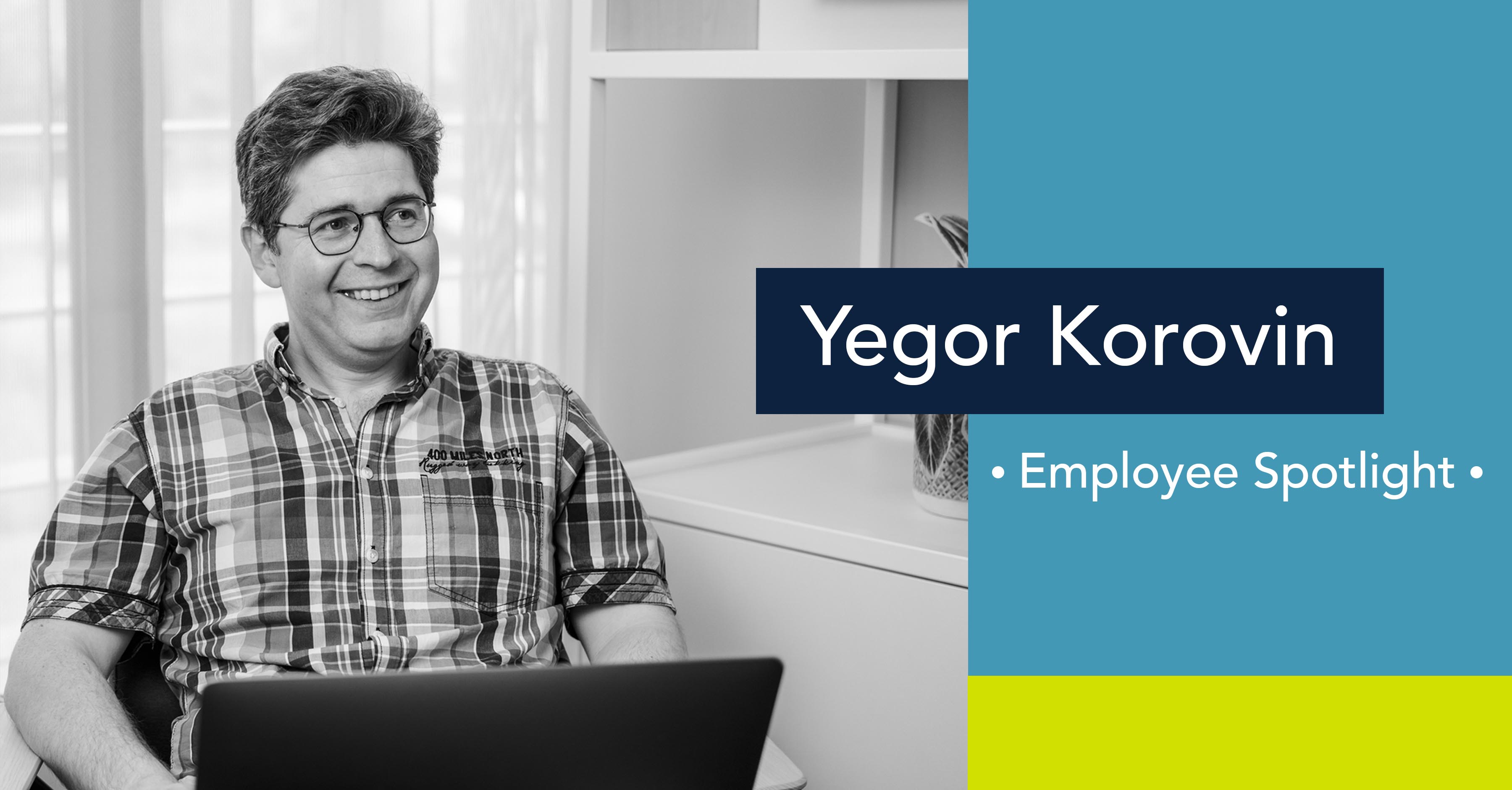
Yegor graduated with a Bachelor of Science degree in Physics before transitioning to a Masters in Theoretical and Mathematical Physics.
Since then, he has been a dyed-in-the-wool mathematical and theoretical physicist, completing his PhD in Physics and then advancing through a series of progressive research positions in prestigious research institutions across Europe.
Yegor’s theoretical research as a Doctoral Researcher at the University of Amsterdam focused on the interface between high energy physics, condensed matter physics and gravity.
As a Postdoctoral Researcher at the Max Planck Institute for Gravitational Physics in Germany, he ran independent research projects into gravity, quantum field theory, formal and applied holography.
And in his three years as an FNRS postdoctoral fellow at the Université libre de Bruxelles, he was involved with original research into statistical field theory, high energy physics, geometry, and mathematical modelling.
Yegor brings a wealth of international experience in theoretical and applied research to his role as a Data Scientist at BioStrand. He also has the aptitude to investigate diverse domains and discover novel concepts relevant to data science and is invested in constantly upgrading his computational skills.
Meet Yegor
What does your role as a BioStrand data scientist entail?
In a start-up environment, data scientists get the opportunity to work on a variety of things: formulating precise and well-defined research questions, trying out different tools and algorithms, coming up with benchmarks, etc. In my particular case, it also involves a lot of learning, since I still have limited expertise in biology and bioinformatics.
As a result, I have to quickly assimilate a substantial amount of domain knowledge for most projects.
Interestingly, data scientists in a start-up often end up wearing other hats as well. So at times, they may have to assume a customer-facing role and provide a marketing-led perspective or even serve as a data engineer in training.
What software or tools do you use every day?
Though it depends on context, I typically use Python as the main programming language, in conjunction with Jupyter Notebooks for quick explorations.
Traditionally, many bioinformatics tasks are directly performed from the command line, so Bash is another language I speak every day. For larger tasks and pipelines I use infrastructure provided by Amazon Web Services.
What are you currently working on?
There are currently quite a few things in the pipeline. For instance, we are gearing up to build a variant calling pipeline for whole-genome sequencing data.
Another interesting project is focused on a particularly nasty class of breast cancer and we are exploring ways to adapt therapies to certain subtypes of this cancer.
We are also launching another larger project to build a comprehensive graph database that will gather together a great variety of metadata corresponding to different biological sequences.
What project are you most proud of and why?
The project I’m most proud of is still to come.
Do you have any tips for new data scientists?
I’m certainly not qualified to dole out tips as a data scientist but from a general perspective, I think that it is important to find something that one is passionate about and enjoys doing.
Of course, being a good team player is hugely important. For something more specific I can only paraphrase someone whom I deeply respect: in data science, or any other well-developed field, look for a tool or skill that is objectively useful and yet, for some reason, remains underappreciated.
Focus on that skill and develop it and it can be truly game-changing for your company and your career.
What do you think are the more interesting areas of your field?
My answer may be biased to my background and experience but I find Geometric Deep Learning extremely interesting, for a couple of reasons.
First, it is a general formulation of Machine Learning that provides a common and unifying view of most ML architectures in use today. This is a great generalisation with extraordinary potential.
And second, my favourite language, which is the mathematical language of geometry and symmetries, plays a crucial role in achieving this generalization.
Overall, I strongly believe that a more fundamental and abstract formulation of ML will be highly beneficial for future developments in areas like applied biology or even Artificial Intelligence in general.
Tell us about what you do when you’re not working on data projects?
I spend time with my wonderful family and learn Brazilian Jiu-Jitsu.
What are some skills you have developed through your career that you think apply beyond work?
Well, I can cut onions without crying.
👏 Photo credit: Georgios Triantopoulos
Subscribe to our Blog and get new articles right after publication into your inbox.